filmov
tv
Factor Graphs, Belief Propagation, and Density Evolution 2/2
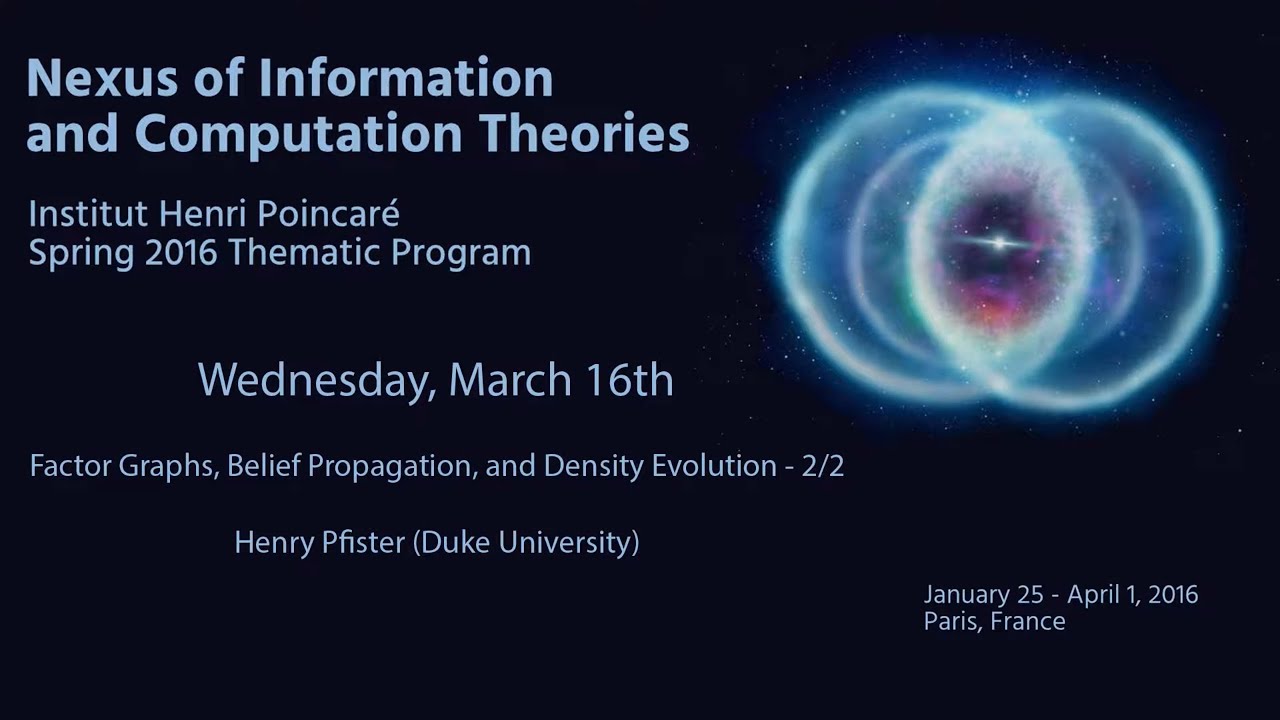
Показать описание
By Henry Pfister (Duke University)
Abstract: The goal of this mini-course is to introduce students to marginal inference techniques for large systems of random variables defined by sparse random factor graphs. Over the past 20 years, these techniques have revolutionized error-correcting codes, compressed sensing, and random satisfiability. In particular, we consider approximate marginal inference based on the low-complexity iterative algorithm called belief propagation (BP). In general, this algorithm is quite effective when the neighborhoods of most variable nodes do not contain short cycles. Density evolution is a technique that, in some cases, allows one to rigorously analyze the asymptotic performance of BP as the size of the sparse random graph increases. Each technique will be illustrated via worked examples and descriptions of how they are used in practice.
Abstract: The goal of this mini-course is to introduce students to marginal inference techniques for large systems of random variables defined by sparse random factor graphs. Over the past 20 years, these techniques have revolutionized error-correcting codes, compressed sensing, and random satisfiability. In particular, we consider approximate marginal inference based on the low-complexity iterative algorithm called belief propagation (BP). In general, this algorithm is quite effective when the neighborhoods of most variable nodes do not contain short cycles. Density evolution is a technique that, in some cases, allows one to rigorously analyze the asymptotic performance of BP as the size of the sparse random graph increases. Each technique will be illustrated via worked examples and descriptions of how they are used in practice.