filmov
tv
Introduction to Deep Reinforcement Learning | Deep RL Course
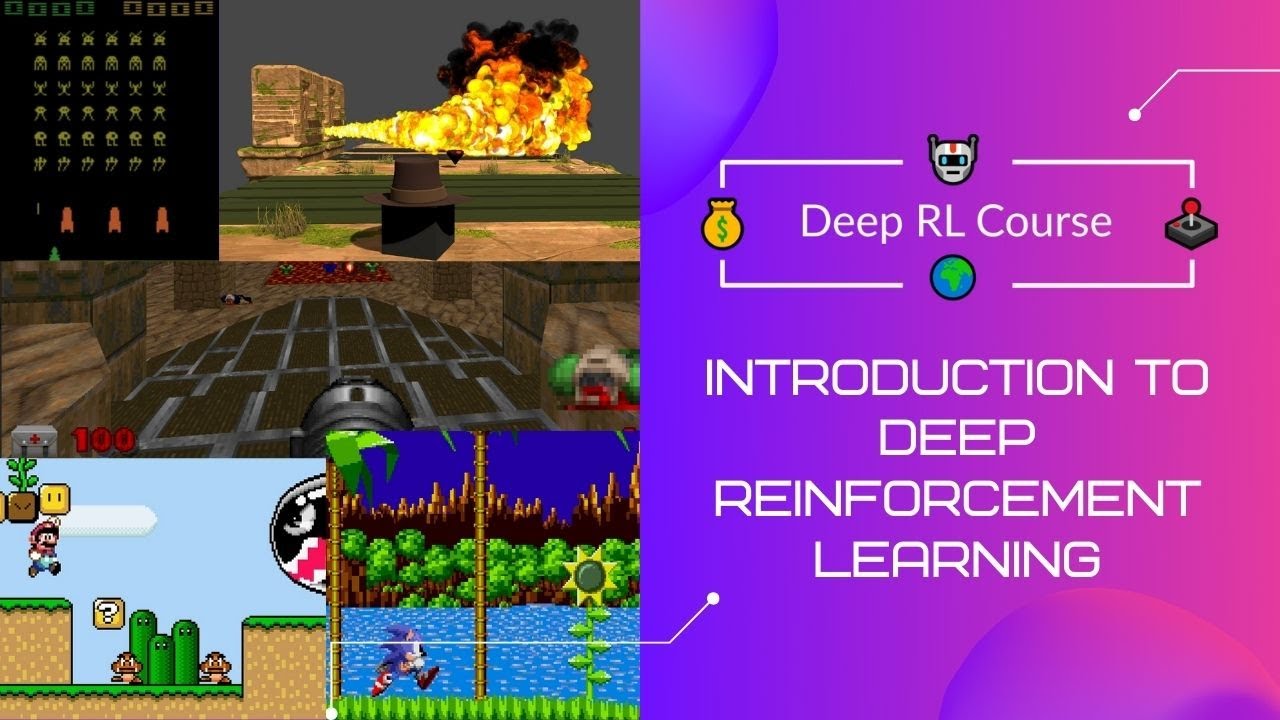
Показать описание
In this first video of the Hugging Face Deep Reinforcement Learning Class 🤗 you’ll learn the fundamentals of deep reinforcement learning: the RL process, the reward hypothesis, policy-based methods, value based-methods... Then, you'll train your first lander agent 🚀 to land correctly on the Moon 🌕 using Stable-Baselines3 and share it with the community.
This video is the first Unit of the Hugging Face Deep Reinforcement Learning Class 🤗, a free course from beginner to expert where you will:
- 📖 Study Deep Reinforcement Learning in theory and practice.
- 🧑💻 Learn to use famous Deep RL libraries such as Stable Baselines3, RL Baselines3 Zoo, and RLlib.
- 🤖 Train agents in unique environments such as SnowballFight, Huggy the Doggo 🐶, and classical ones such as Space Invaders and PyBullet.
- 💾 Publish your trained agents in one line of code to the Hugging Face Hub. But also download powerful agents from the community.
- 🏆 Participate in challenges where you will evaluate your agents against other teams.
- 🖌️🎨 Learn to share your own environments made with Unity and Godot.
Timeline:
0:00 - 2:05: Introduction
2:06 - 4:03: The RL definition
4:04 - 5:03: The RL Loop
5:04 - 5:32: Reward Hypothesis
5:34 - 6:12: Markov Property
6:13 - 7:34: Observation Space
7:35 - 8:34: Action Space
8:35 - 10:43: Rewards and discounting
10:44 - 13:54: Exploration/Exploitation tradeoff
13:55 - 15:02: The Policy
15:03 - 17:03: Policy-based methods
17:04 - 19:23: Value-based methods
19:24 - 20:43: The Deep in Deep RL
20:44 - 22:14: Conclusion
Don’t forget 👍 to like the video, subscribe, and ring the bell 🔔 so that you don’t miss the next video.
Keep Learning, Stay Awesome
This video is the first Unit of the Hugging Face Deep Reinforcement Learning Class 🤗, a free course from beginner to expert where you will:
- 📖 Study Deep Reinforcement Learning in theory and practice.
- 🧑💻 Learn to use famous Deep RL libraries such as Stable Baselines3, RL Baselines3 Zoo, and RLlib.
- 🤖 Train agents in unique environments such as SnowballFight, Huggy the Doggo 🐶, and classical ones such as Space Invaders and PyBullet.
- 💾 Publish your trained agents in one line of code to the Hugging Face Hub. But also download powerful agents from the community.
- 🏆 Participate in challenges where you will evaluate your agents against other teams.
- 🖌️🎨 Learn to share your own environments made with Unity and Godot.
Timeline:
0:00 - 2:05: Introduction
2:06 - 4:03: The RL definition
4:04 - 5:03: The RL Loop
5:04 - 5:32: Reward Hypothesis
5:34 - 6:12: Markov Property
6:13 - 7:34: Observation Space
7:35 - 8:34: Action Space
8:35 - 10:43: Rewards and discounting
10:44 - 13:54: Exploration/Exploitation tradeoff
13:55 - 15:02: The Policy
15:03 - 17:03: Policy-based methods
17:04 - 19:23: Value-based methods
19:24 - 20:43: The Deep in Deep RL
20:44 - 22:14: Conclusion
Don’t forget 👍 to like the video, subscribe, and ring the bell 🔔 so that you don’t miss the next video.
Keep Learning, Stay Awesome
Комментарии