filmov
tv
Is Data Science Dying in 2022?
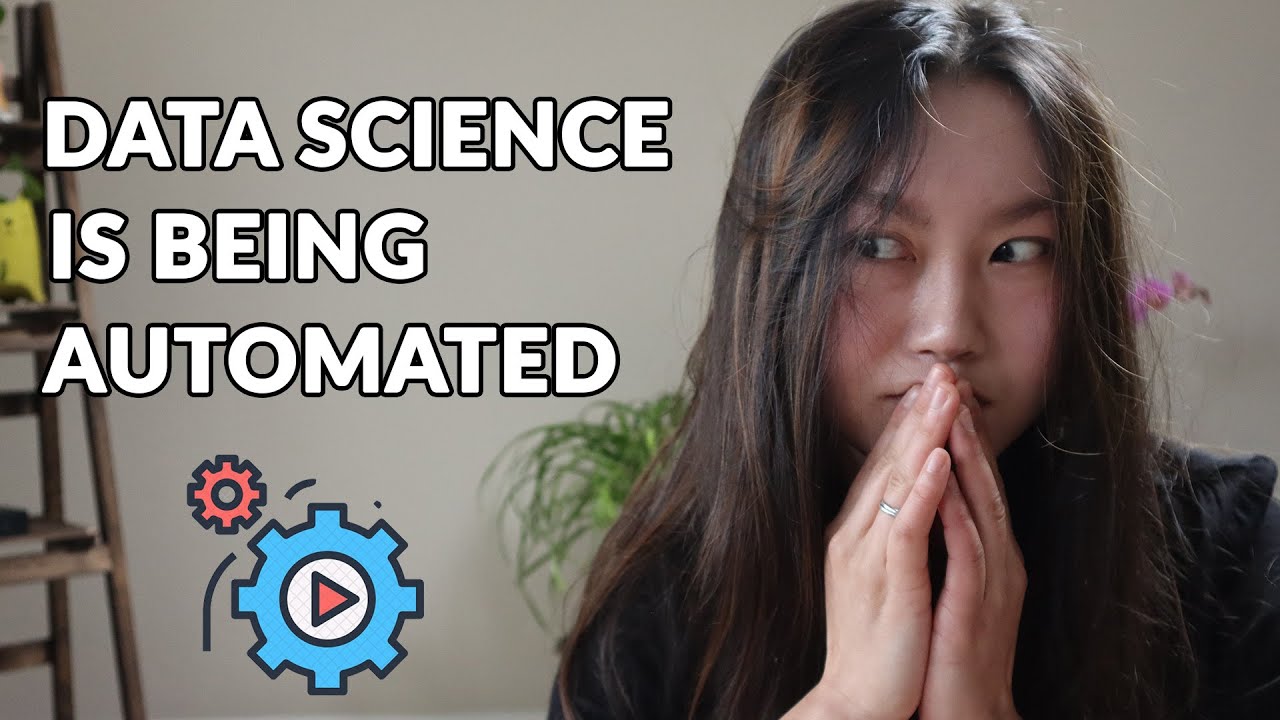
Показать описание
Free Dataiku Learning Resource:
In this video, I talk about if data science is a dying field in 2022. I think this is a very legitimate concern since we've seen whole industries become obsolete in a matter of years as technology keeps advancing.
From LEARN Media
In this video, I talk about if data science is a dying field in 2022. I think this is a very legitimate concern since we've seen whole industries become obsolete in a matter of years as technology keeps advancing.
From LEARN Media
Is Data Science Dying?
Is Data Science Really Dying? Techie-La
Is Data Science dying? End of 2022 UPDATE
Is Data Science a Dying Career? Data Science Job Market in 2023
Will AI Replace Data Analyst Jobs?
Is Data Science Dying in 2022?
Is Data Science Dying?
What is Data Science?
Data Science Training : Explore Life and Death Issues in 2024 Call or WhatsApp +91 8886552866
'Data Science is Dying'
Is Data Scientist Role Dying?
What Does Data Scientist Actually do?
Is Data Science Dying?
Is Data Science A Good Career?
Is data science a hype? | Is data science a bubble?
Is Data Science Dying? ( Scope & Job Market in 2024)
Is Becoming a Data Scientist Hard?
Why you should not be a data scientist
Data Science Has Changed - Here's What to Do
Is Python Really Needed For a Data Analyst Job?
Is Data Science Dying in 2024? || AI and Automation
How could Data Science by Dying?
Data Science Career: (Is Becoming A Data Scientist ACTUALLY Worth It?)
Is data science dying? #datascience #machinelearning #shorts #deeplearning #python
Комментарии