filmov
tv
Building LLM Agents in 3 Levels of Complexity: From Scratch, OpenAI Functions & LangChain
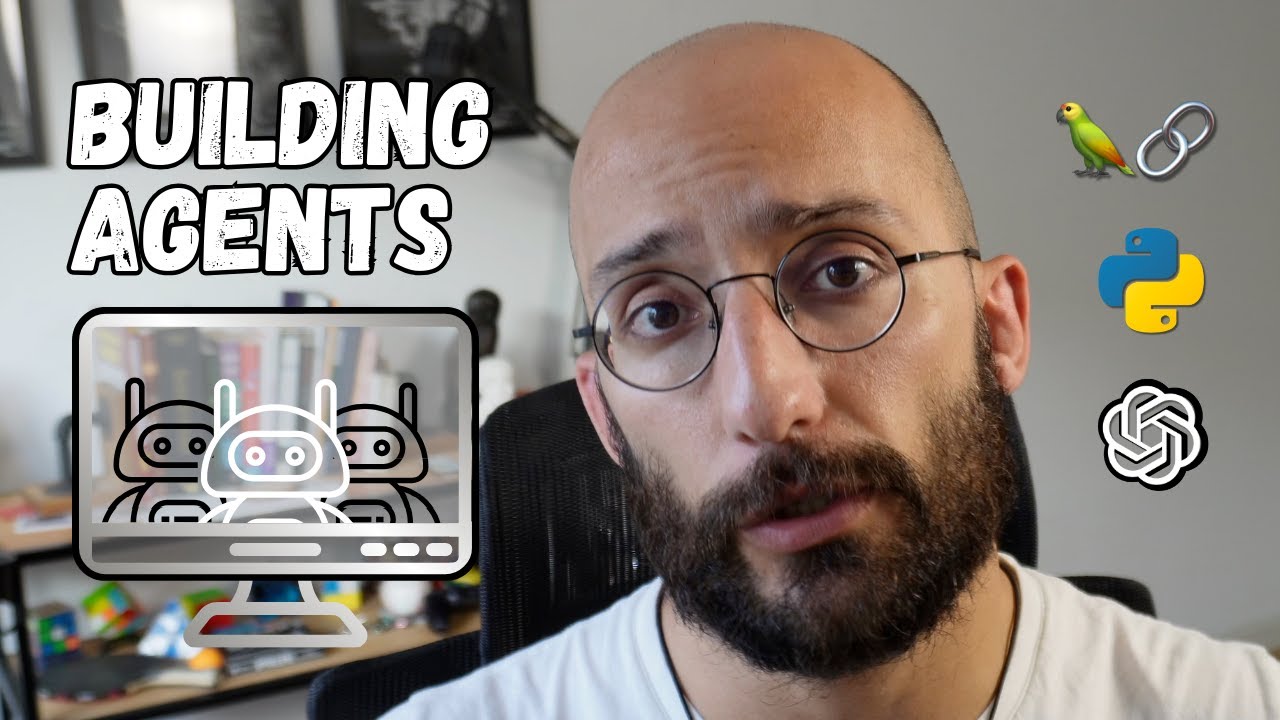
Показать описание
In this video, let's work through the basics of building LLM-based agents. First we'll use only basic openai API calls coupled with some hacky prompting to allow the models to call Python functions. Then, we'll look at OpenAI functions and LangChain as more advanced options.
📚 Chapters:
00:02 - Introduction to the video and overview of agents and their capabilities.
00:41 - Discussion on choosing frameworks for building agents.
00:54 - Exploring large language models for real world actions.
01:48 - Building simple agents using LangChain.
02:39 - Setting up and testing OpenAI API.
03:40 - Connecting large language models with Python functions.
04:25 - Creating and testing directory and file management functions.
05:50 - Toolformer paper.
06:14 - Writing a class to organize functionalities.
06:56 - Planning tasks and executing actions with the model.
07:21 - Improving functions for practical use.
07:43 - Setting up and testing tasks with the model.
08:03 - Examining model output for function calls.
09:19 - Using Python's exec function for model outputs.
10:37 - Discussion on extending model capabilities without frameworks.
11:05 - Improving prompt engineering for function calls.
12:10 - Testing model's ability to organize function calls.
13:06 - Challenges in scaling model complexity.
14:11 - Introduction to OpenAI function calling with JSON.
16:00 - Detailed explanation of OpenAI function calling.
18:10 - Using OpenAI function calling for directory creation.
19:06 - Structuring function calls for better control.
20:49 - Jumping from strings to JSON in function calling.
21:51 - Example of function calling using OpenAI API.
23:08 - Exploring tool functionality in function calls.
24:34 - Setting up a run function for OpenAI function calling.
26:31 - Discussion on layers of complexity in agent building.
27:32 - Implementing OpenAI function calling in previous examples.
29:23 - Testing function calls with OpenAI API.
30:13 - Transition to using LangChain for agent development.
31:44 - Setting up tools and agent executor in LangChain.
33:21 - Running an agent task with LangChain.
34:21 - Advantages of LangChain in agent development.
35:56 - Adding and testing new functions with LangChain.
38:07 - Discussing the composability of LangChain components.
40:57 - Live example of extending LangChain functionality.
42:48 - Concluding thoughts on building agents with different frameworks.
🔗 Links:
🔗 More Links
📚 Chapters:
00:02 - Introduction to the video and overview of agents and their capabilities.
00:41 - Discussion on choosing frameworks for building agents.
00:54 - Exploring large language models for real world actions.
01:48 - Building simple agents using LangChain.
02:39 - Setting up and testing OpenAI API.
03:40 - Connecting large language models with Python functions.
04:25 - Creating and testing directory and file management functions.
05:50 - Toolformer paper.
06:14 - Writing a class to organize functionalities.
06:56 - Planning tasks and executing actions with the model.
07:21 - Improving functions for practical use.
07:43 - Setting up and testing tasks with the model.
08:03 - Examining model output for function calls.
09:19 - Using Python's exec function for model outputs.
10:37 - Discussion on extending model capabilities without frameworks.
11:05 - Improving prompt engineering for function calls.
12:10 - Testing model's ability to organize function calls.
13:06 - Challenges in scaling model complexity.
14:11 - Introduction to OpenAI function calling with JSON.
16:00 - Detailed explanation of OpenAI function calling.
18:10 - Using OpenAI function calling for directory creation.
19:06 - Structuring function calls for better control.
20:49 - Jumping from strings to JSON in function calling.
21:51 - Example of function calling using OpenAI API.
23:08 - Exploring tool functionality in function calls.
24:34 - Setting up a run function for OpenAI function calling.
26:31 - Discussion on layers of complexity in agent building.
27:32 - Implementing OpenAI function calling in previous examples.
29:23 - Testing function calls with OpenAI API.
30:13 - Transition to using LangChain for agent development.
31:44 - Setting up tools and agent executor in LangChain.
33:21 - Running an agent task with LangChain.
34:21 - Advantages of LangChain in agent development.
35:56 - Adding and testing new functions with LangChain.
38:07 - Discussing the composability of LangChain components.
40:57 - Live example of extending LangChain functionality.
42:48 - Concluding thoughts on building agents with different frameworks.
🔗 Links:
🔗 More Links
Комментарии