filmov
tv
Multiple Regression in R, Step-by-Step!!!
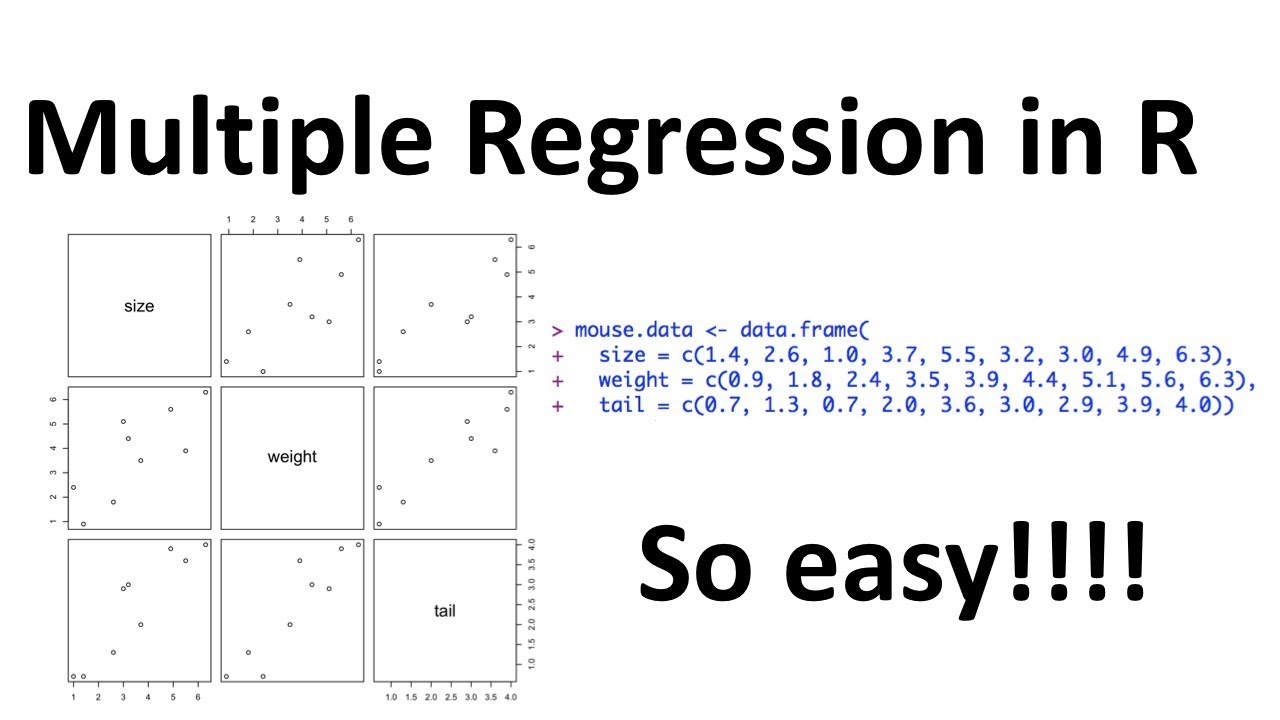
Показать описание
For a complete index of all the StatQuest videos, check out:
If you'd like to support StatQuest, please consider...
...or...
...buy my book, a study guide, a t-shirt or hoodie, or a song from the StatQuest store...
...or just donating to StatQuest!
Lastly, if you want to keep up with me as I research and create new StatQuests, follow me on twitter:
#statquest #regression
Multiple Regression in R, Step by Step!!!
Multiple Regression in R, Step-by-Step!!!
Multiple Linear Regression in R | R Tutorial 5.3 | MarinStatsLectures
Multiple lineare Regression in R [ALL IN ONE] - Voraussetzungen, Durchführung und Interpretation
Multiple regression in R: example
How To... Create a Multiple Linear Regression Model in R #101
Linear Regression in R, Step by Step
R Tutorial: Multiple Linear Regression
Learn Linear regression in 6 hours session 37
Quick tutorial on how to run Multiple regression in R
Multiple linear regression in R
Multiple linear regression using R studio (Aug 2022)
Schritt für Schritt - Multiple lineare Regression in R rechnen und interpretieren
Multiple linear regression with interaction in R
Multiple Regression, Clearly Explained!!!
Econometrics - Multiple Regression in R
Multiple Linear Regression Using R
Linear Regression in R, Step-by-Step
Multiple regression: how to select variables for your model
MULTIPLE REGRESSION IN R SOFTWARE
Multivariable Logistic Regression in R: The Ultimate Masterclass (4K)!
Multiple regression in R tutorial (Jan 2020)
Multiple Linear Regression using R ( All about it )
How To... Make a Prediction using a Multiple Linear Regression Model in R #102
Комментарии