filmov
tv
Deep Phenotyping of Heart Failure: Integrating Mechanistic Modelling and Machine Learning
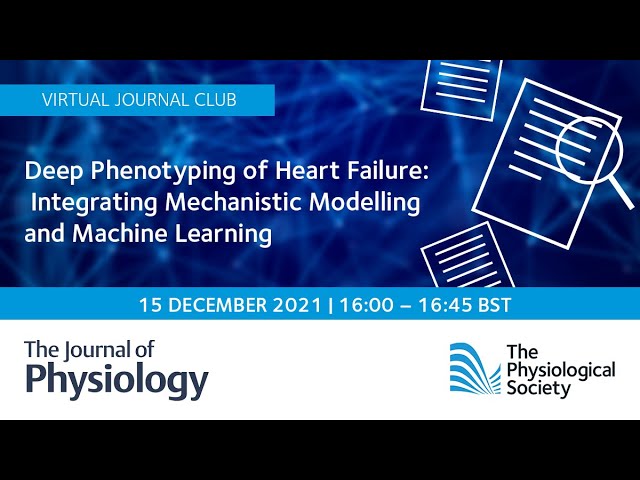
Показать описание
Paper : Phenotyping heart failure using model-based analysis and physiology-informed machine learning (Jones E., Randall E.B., Hummel S.L., Cameron D.M., Beard D.A., Carlson B.E.), The Journal of Physiology; DOI:10.1113/JP281845.
Heart Failure (HF) poses a significant individual and public health burden and is often categorized based on ejection fraction (EF) and into two types: heart failure with preserved ejection fraction (HFpEF) and heart failure with reduced ejection fraction (HFrEF). However, HF patients present substantial physiological and phenotypical heterogeneities. Therefore, improving HF treatment requires identifying and discerning subgroups of HF based on physiological and clinical data at the systems level. In this Virtual Journal club, the panellists discussed an integrative mechanistic modelling and machine learning study to identify and characterize mechanistic differences between HFpEF subgroups using patient-specific clinical data.
Each meeting of The Journal of Physiology’s Virtual Journal Club explores a different paper, recently published in The Journal of Physiology. Discussions also consider best practice for publication.
Heart Failure (HF) poses a significant individual and public health burden and is often categorized based on ejection fraction (EF) and into two types: heart failure with preserved ejection fraction (HFpEF) and heart failure with reduced ejection fraction (HFrEF). However, HF patients present substantial physiological and phenotypical heterogeneities. Therefore, improving HF treatment requires identifying and discerning subgroups of HF based on physiological and clinical data at the systems level. In this Virtual Journal club, the panellists discussed an integrative mechanistic modelling and machine learning study to identify and characterize mechanistic differences between HFpEF subgroups using patient-specific clinical data.
Each meeting of The Journal of Physiology’s Virtual Journal Club explores a different paper, recently published in The Journal of Physiology. Discussions also consider best practice for publication.