filmov
tv
Visualizing Data Using t-SNE
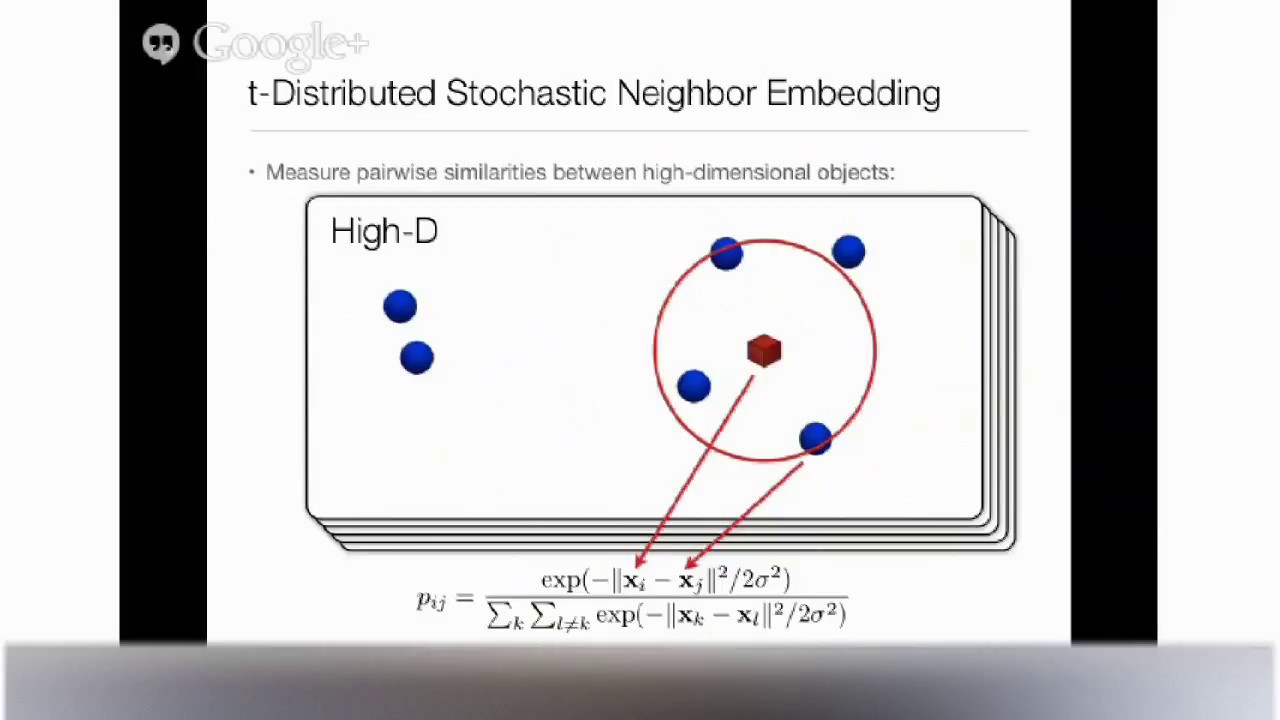
Показать описание
Google Tech Talk
June 24, 2013
(more info below)
Presented by Laurens van der Maaten, Delft University of Technology, The Netherlands
ABSTRACT
Visualization techniques are essential tools for every data scientist. Unfortunately, the majority of visualization techniques can only be used to inspect a limited number of variables of interest simultaneously. As a result, these techniques are not suitable for big data that is very high-dimensional.
An effective way to visualize high-dimensional data is to represent each data object by a two-dimensional point in such a way that similar objects are represented by nearby points, and that dissimilar objects are represented by distant points. The resulting two-dimensional points can be visualized in a scatter plot. This leads to a map of the data that reveals the underlying structure of the objects, such as the presence of clusters.
We present a new technique to embed high-dimensional objects in a two-dimensional map, called t-Distributed Stochastic Neighbor Embedding (t-SNE), that produces substantially better results than alternative techniques. We demonstrate the value of t-SNE in domains such as computer vision and bioinformatics. In addition, we show how to scale up t-SNE to big data sets with millions of objects, and we present an approach to visualize objects of which the similarities are non-metric (such as semantic similarities).
This talk describes joint work with Geoffrey Hinton.
June 24, 2013
(more info below)
Presented by Laurens van der Maaten, Delft University of Technology, The Netherlands
ABSTRACT
Visualization techniques are essential tools for every data scientist. Unfortunately, the majority of visualization techniques can only be used to inspect a limited number of variables of interest simultaneously. As a result, these techniques are not suitable for big data that is very high-dimensional.
An effective way to visualize high-dimensional data is to represent each data object by a two-dimensional point in such a way that similar objects are represented by nearby points, and that dissimilar objects are represented by distant points. The resulting two-dimensional points can be visualized in a scatter plot. This leads to a map of the data that reveals the underlying structure of the objects, such as the presence of clusters.
We present a new technique to embed high-dimensional objects in a two-dimensional map, called t-Distributed Stochastic Neighbor Embedding (t-SNE), that produces substantially better results than alternative techniques. We demonstrate the value of t-SNE in domains such as computer vision and bioinformatics. In addition, we show how to scale up t-SNE to big data sets with millions of objects, and we present an approach to visualize objects of which the similarities are non-metric (such as semantic similarities).
This talk describes joint work with Geoffrey Hinton.
Комментарии