filmov
tv
Approximating Functions in a Metric Space
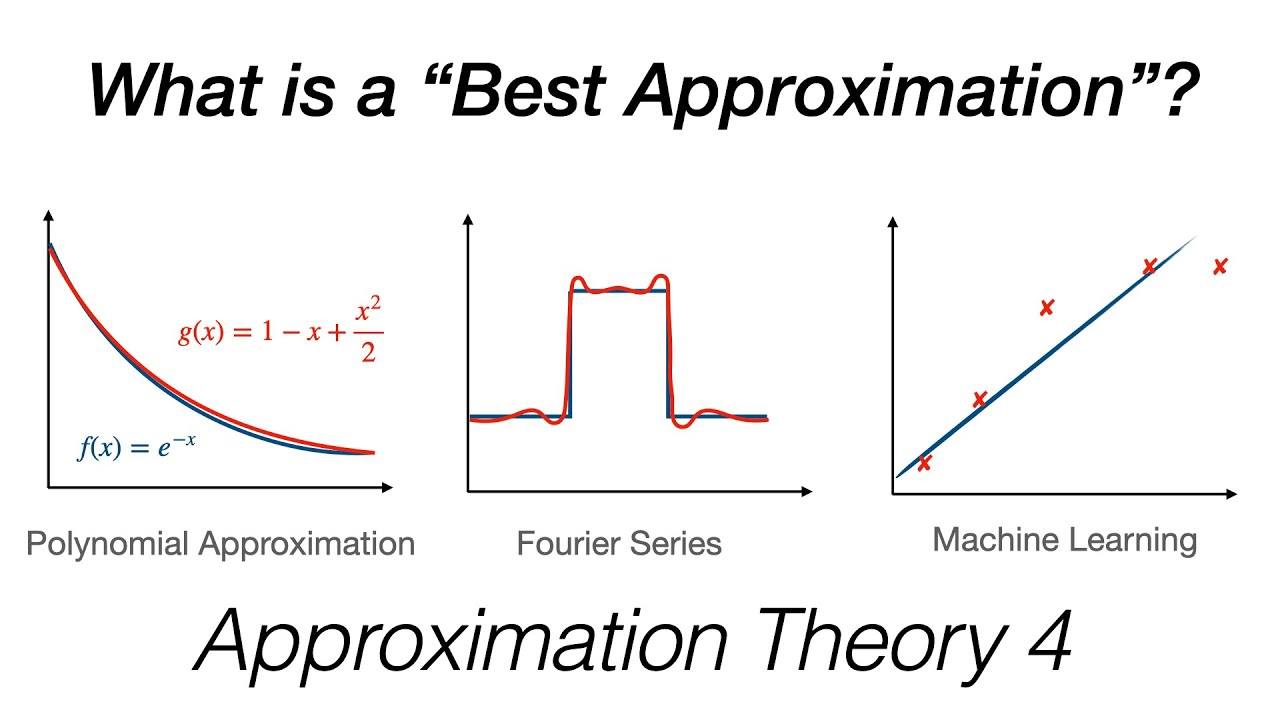
Показать описание
Approximations are common in many areas of mathematics from Taylor series to machine learning.
In this video, we will define what is meant by a best approximation and prove that a best approximation exists in a metric space.
Chapters
0:00 - Examples of Approximation
0:46 - Best Aproximations (definition)
2:32 - Existence proof
7:18 - Summary
The product links below are Amazon affiliate links. If you buy certain products on Amazon soon after clicking them, I may receive a commission. The price is the same for you, but it does help to support the channel :-)
The Approximation Theory series is based on the book "Approximation Theory and Methods" by M.J.D. Powell:
Errata and Clarifications:
A compact subset of a metric space is closed and bounded but a closed and bounded subset of a metric space is not always compact. Please consider the closed versus open set section as illustrative only as the proof strictly requires compactness.
This video was made using:
Animation - Apple Keynote
Editing - DaVinci Resolve
Supporting the Channel.
If you would like to support me in making free mathematics tutorials then you can make a small donation over at
Thank you so much, I hope you find the content useful.
In this video, we will define what is meant by a best approximation and prove that a best approximation exists in a metric space.
Chapters
0:00 - Examples of Approximation
0:46 - Best Aproximations (definition)
2:32 - Existence proof
7:18 - Summary
The product links below are Amazon affiliate links. If you buy certain products on Amazon soon after clicking them, I may receive a commission. The price is the same for you, but it does help to support the channel :-)
The Approximation Theory series is based on the book "Approximation Theory and Methods" by M.J.D. Powell:
Errata and Clarifications:
A compact subset of a metric space is closed and bounded but a closed and bounded subset of a metric space is not always compact. Please consider the closed versus open set section as illustrative only as the proof strictly requires compactness.
This video was made using:
Animation - Apple Keynote
Editing - DaVinci Resolve
Supporting the Channel.
If you would like to support me in making free mathematics tutorials then you can make a small donation over at
Thank you so much, I hope you find the content useful.
Комментарии