filmov
tv
Neural Networks in pure JAX (with automatic differentiation)
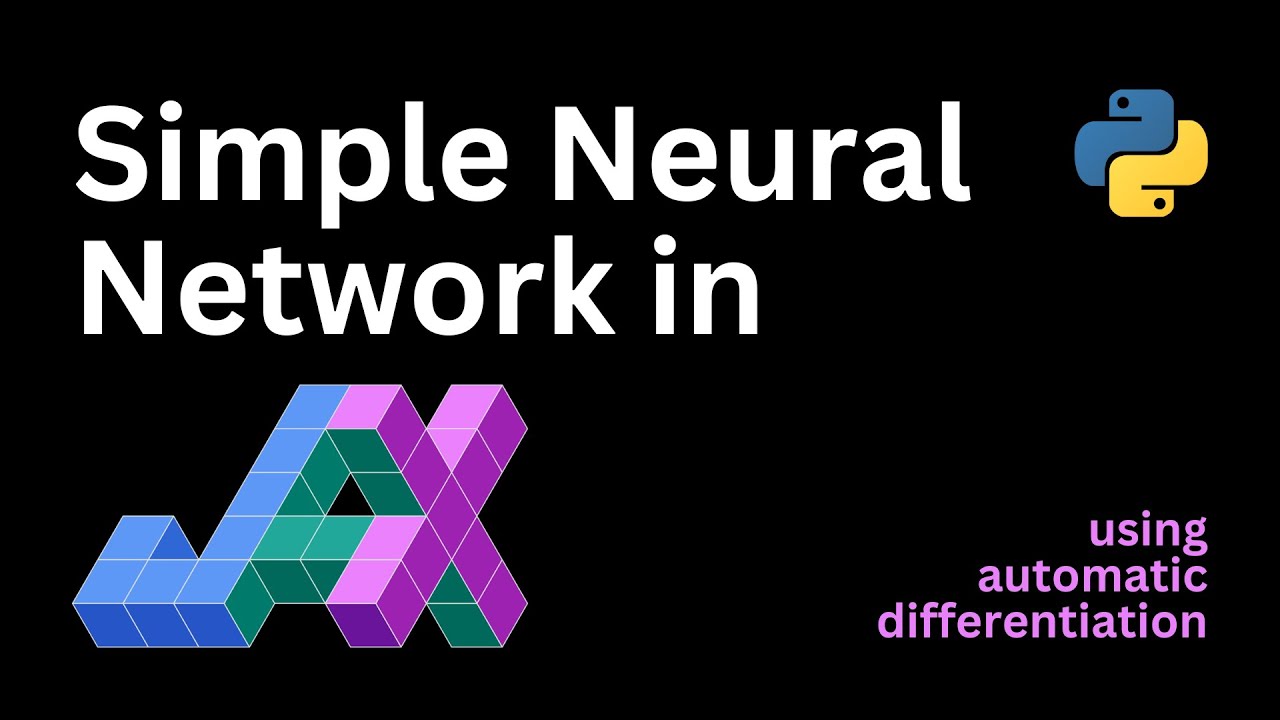
Показать описание
-------
-------
-------
Timestamps:
00:00 Intro
01:18 Dataset that somehow looks like a sine function
01:56 Forward pass of the Multilayer Perceptron
03:22 Weight initialization due to Xavier Glorot
04:20 Idea of "Learning" as approximate optimization
04:49 Reverse-mode autodiff requires us to only write the forward pass
05:34 Imports
05:52 Constants and Hyperparameters
06:19 Producing the random toy dataset
08:33 Draw initial parameter guesses
12:05 Implementing the forward/primal pass
13:58 Implementing the loss metric
14:57 Transform forward pass to get gradients by autodiff
20:03 Training loop (using plain gradient descent)
23:21 Improving training speed by JIT compilation
24:25 Plotting loss history
24:47 Plotting final network prediction & Discussion
25:44 Summary
26:59 Outro
Neural Networks in pure JAX (with automatic differentiation)
Coding a Neural Network from Scratch in Pure JAX | Machine Learning with JAX | Tutorial #3
What is JAX?
Implementing Neural Network in Mid Night from Scratch using JAX with Power Level quite fun 😁😁🐱🏍...
JAX in 100 Seconds
Neural Networks in Equinox (JAX DL framework) with Optax
Machine Learning with JAX - From Zero to Hero | Tutorial #1
Who uses JAX?
Intro to JAX: Accelerating Machine Learning research
What is the Jax Deep Learning Framework?
Comparing Automatic Differentiation in JAX, TensorFlow and PyTorch #shorts
WHY JAX? Why the Hell a 3rd ML framework in 2023?
Why FLAX Could Be Your New Favorite Deep Learning Library for NN
Simon Pressler: Getting started with JAX
Create a Neural Network using Jax and predict on the seeds dataset
Introduction to JAX 2023
JAX Course - 2. Working with Neural Networks in JAX
Intro to Machine Learning with JAX
Tensorflow 2 One Year Later (plus PyTorch, JAX, and Julia) (Episode 13)
Strange JRAPH - Deep Mind's GNN Library for Graph Neural Networks (w/ JAX)
03. Jit Explained | JAX For Deep Learning
Fourier Neural Operators (FNO) in JAX
EI Seminar - Matthew Johnson - JAX: accelerated ML research via composable function transformations
JAX Crash Course - Accelerating Machine Learning code!
Комментарии