filmov
tv
Rethinking Feature Stores
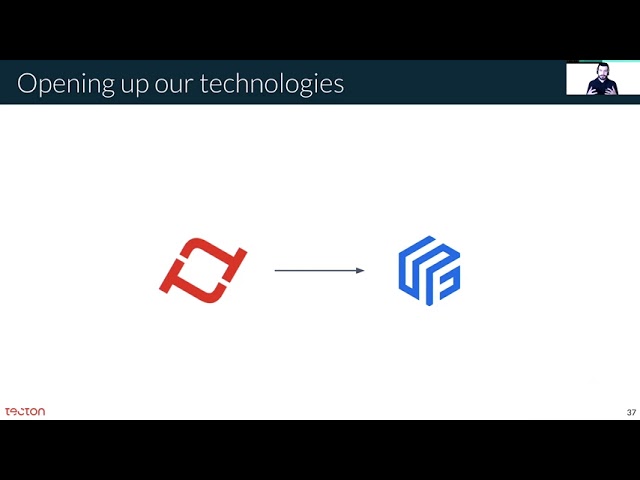
Показать описание
Feature stores have emerged as a pivotal component in the modern machine learning stack. They solve some of the toughest challenges in data for machine learning, namely feature computation, storage, validation, serving, and reuse.
However, the deployment of feature stores still requires a coordinated effort from multiple teams, comes with a large infrastructural footprint, and leads to integration costs and significant operational overheads. This large investment places feature stores completely out of reach for the average data team. What’s needed is a fundamental redesign of the feature store.
In this talk we will introduce the virtual feature store, a framework that allows any data source to be operationalized by declaring them as dependencies to production ML applications.
The virtual feature store provides a single lightweight abstraction to feature data, creating a view of features that decouples models from environment specific infrastructure. By publishing model-centric logical feature definitions, data scientists can now build ML applications that depend on any data source, using their tools of choice, and deploy to their existing production infrastructure.
In this talk we will also demonstrate how this new paradigm empowers individual data scientists to develop and serve a production-grade ML application in less than one minute.
Connect with us:
However, the deployment of feature stores still requires a coordinated effort from multiple teams, comes with a large infrastructural footprint, and leads to integration costs and significant operational overheads. This large investment places feature stores completely out of reach for the average data team. What’s needed is a fundamental redesign of the feature store.
In this talk we will introduce the virtual feature store, a framework that allows any data source to be operationalized by declaring them as dependencies to production ML applications.
The virtual feature store provides a single lightweight abstraction to feature data, creating a view of features that decouples models from environment specific infrastructure. By publishing model-centric logical feature definitions, data scientists can now build ML applications that depend on any data source, using their tools of choice, and deploy to their existing production infrastructure.
In this talk we will also demonstrate how this new paradigm empowers individual data scientists to develop and serve a production-grade ML application in less than one minute.
Connect with us:
Комментарии