filmov
tv
Decoding AI's Blind Spots: Solving Causal Reasoning
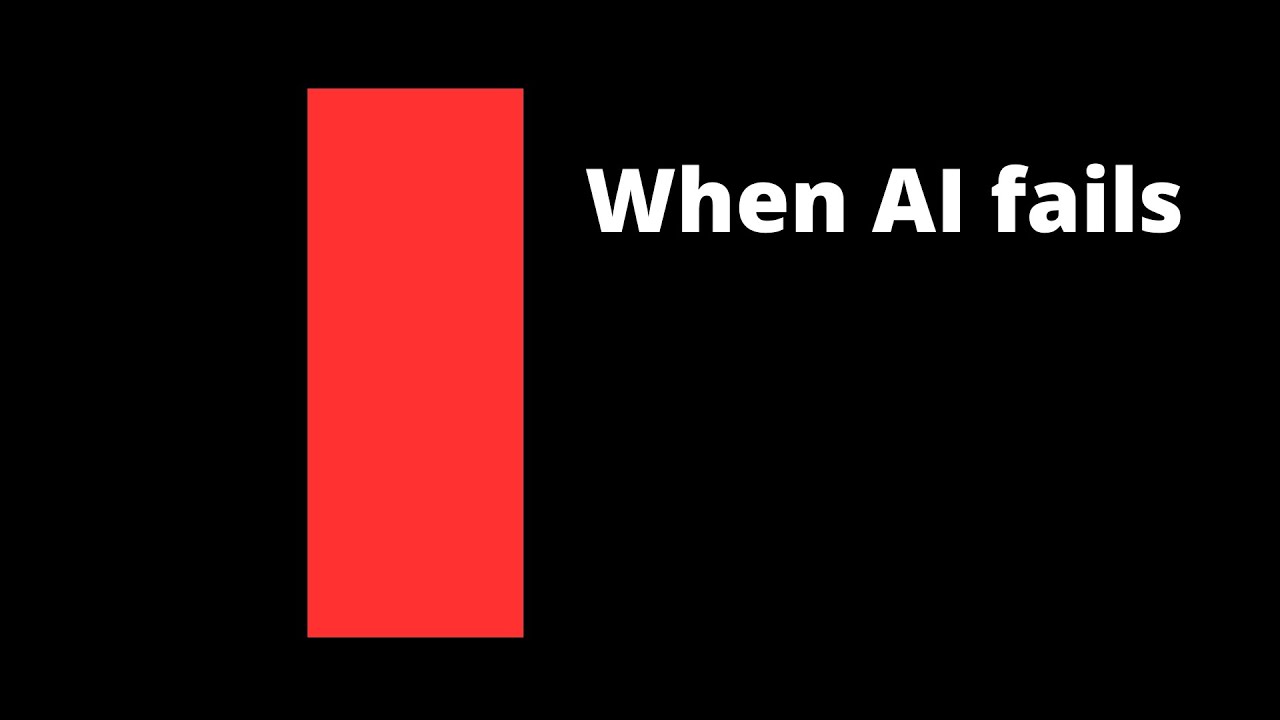
Показать описание
Great response from the AI community on my prompt, testing causal reasoning, where all LMMs failed.
Here now my response.
#airesearch
#aieducation
#failure
Decoding AI's Blind Spots: Solving Causal Reasoning
xavier memes #memes
Crazy tick removal? Or fake?
Sudoku Secrets No. 2: The X-Wing #shorts
Shortest ever IQ test: The Donut Question 🍩 #shorts
Dark web disturbing videos #shorts
Mr. Robot Sucks
Christian destroys Islam … Muslim shocked 😳 #christianity #islam #shorts
8 patterns to solve 80% Leetcode problems
But what are Hamming codes? The origin of error correction
How To Handle Passive Aggressive Attacks #shorts #meghanmarkle #katemiddleton #practicalpsychology
NEVER buy from the Dark Web.. #shorts
voice Translator app | voice Translator
The Secret To Chess...
Why did this happen to me (Rubik's Cube FAIL)
IShowSpeed is a delight
Clinically proven stretch mark treatment ❤️
I was wrong about this trick 😧 (true magic?)
Bryan Cranston says 'MAGA' could be viewed as a racist remark
Turn Anything Into A Speaker😱 #travelhack
RegInt: Decoding AI Regulation #6 | Unmasking AI - Faces Behind the Code
This Video is not in Reverse.
Is Rainbolt Cheating? How He Does It
Decoding AI | Session 1: What is AI? || Harvard Radcliffe Institute
Комментарии