filmov
tv
Linear Regression in 5 minutes! → 4 Easy Steps in EXCEL- Project Management & Quality Assurance
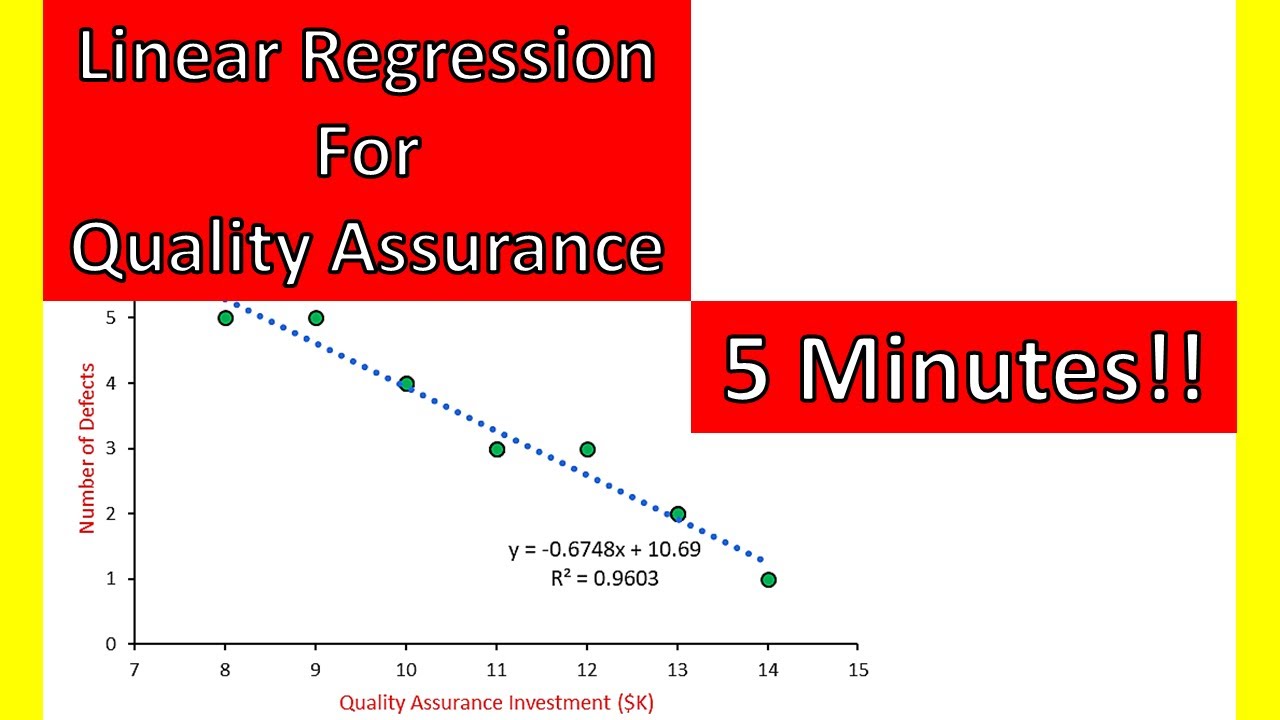
Показать описание
In this video of #engineeringmanagementacademy #LinearRegression is used for #ProjectQualityManagement by #drmehrdadarashpour
⌛ TIMESTAMPS
0:00 - Introduction to Linear Regression in Excel
0:06 - Excel’s Dynamic Template, Scatter Plot with a Trendline, LINEST Function, Linear Regression in Data Analysis Tools
0:26 - Step 1 (Identifying Variables & Creating the Scatter Plot with a Trendline)
1:29 - Step 2 (Using LINEST Function or Linear Estimation to find the best-fit line)
2:15 - Step 3 (Using Data Analysis Tools for Linear Regression)
3:04 - Step 4 (Interpreting Regression Results in Excel)
4:00 - Forecasting Future Outcomes
Step 1 is Identifying Variables & Creating Scatter Plot with a Trendline
Our case study includes 10 projects with observations on the number of defects and investment amounts on quality assurance in thousand dollars. To investigate relationship between the two variables, we can draw a scatter plot from the “insert” menu. The intercept of 10.69 shows the number of defects when zero investment is made on QA! R-squared value or coefficient of determination is 0.9603. This high R-squared value suggests a good fit of the model & indicates that 0.96% of the variability observed in quality defects is explained by QA investment.
Step 2 is Using LINEST Function or Linear Estimation to find the best-fit line
We can numerically calculate the slope & intercept of the fitted line in our model using the LINEST Function. Select an array of 2 cells & insert the function. The first argument is known_ys, which is number of defects in this example. The 2nd argument after the comma is known_xs, which is QA investment. Remember to press “shift+Ctrl+Enter” for array calculations in Excel. There is another method for array calculations by using the INDEX Function. Slope is calculated using INDEX(LINEST) with the last argument equal to 1. Intercept is calculated using INDEX(LINEST) with the last argument equal to 2. Numeric calculations by Excel functions provide the same results as the scatter plot with trend lines. Both methods try to find the best-fit line that minimizes the sum of squared differences between observed and predicted values.
Step 3 is Using Data Analysis Tools for Linear Regression
Click on ‘Data analysis’ & select ‘Regression’ from the list. ‘Input Y Range’ is the dependent variable, which is the column related to ‘number of defects’ in our example. ‘Input X Range’ is the independent variable, which is the column related to QA investment. Remember to check the box for ‘labels’ as we have selected column headings. The outputs can appear in a new worksheet & we check the box for ‘Residuals’ to be calculated.
In the ‘summary output’ worksheet, regression statistics are shown, which will be interpreted in our next step.
Step 4 is Interpreting Regression Results in Excel
we can find Standard Error For our 10 observations, which is standard deviation of the error term & a measure of prediction accuracy. R Square is the coefficient of determination & measures how much of the variation in the dependent variable can be explained by the independent variable. It ranges from 0 to 1, with desirable values close to 1. Multiple R is the correlation coefficient & measures the strength & direction of the linear relationship between the dependent & independent variables. Its value ranges from -1 to 1.
Analysis of Variance or ANOVA shows some results, including df or Degrees of Freedom, which is associated with the sources of variance. Number of observations minus 1 gives the total df, which is 9 in our example. SS or Sum of Squares is a measure of variation. MS or Mean Square is SS divided by the degree of freedom. F is the test statistic for the analysis of variance. Significance F is the p-value associated with the F statistic. We can also find the intercept & slope of the fitted line under coefficients.
The residuals in the last table show the difference between model’s prediction of defects and the ground truth in each observed project.
Finally, let’s focus on Forecasting future outcomes.
We can use the best-fitting model to predict number of defects in future projects. Let’s focus on the worst-case scenario in which no investment is made on QA.
And here it is, an easy& short implementation of linear regression using only 4 simple steps in Microsoft Excel.
⌛ TIMESTAMPS
0:00 - Introduction to Linear Regression in Excel
0:06 - Excel’s Dynamic Template, Scatter Plot with a Trendline, LINEST Function, Linear Regression in Data Analysis Tools
0:26 - Step 1 (Identifying Variables & Creating the Scatter Plot with a Trendline)
1:29 - Step 2 (Using LINEST Function or Linear Estimation to find the best-fit line)
2:15 - Step 3 (Using Data Analysis Tools for Linear Regression)
3:04 - Step 4 (Interpreting Regression Results in Excel)
4:00 - Forecasting Future Outcomes
Step 1 is Identifying Variables & Creating Scatter Plot with a Trendline
Our case study includes 10 projects with observations on the number of defects and investment amounts on quality assurance in thousand dollars. To investigate relationship between the two variables, we can draw a scatter plot from the “insert” menu. The intercept of 10.69 shows the number of defects when zero investment is made on QA! R-squared value or coefficient of determination is 0.9603. This high R-squared value suggests a good fit of the model & indicates that 0.96% of the variability observed in quality defects is explained by QA investment.
Step 2 is Using LINEST Function or Linear Estimation to find the best-fit line
We can numerically calculate the slope & intercept of the fitted line in our model using the LINEST Function. Select an array of 2 cells & insert the function. The first argument is known_ys, which is number of defects in this example. The 2nd argument after the comma is known_xs, which is QA investment. Remember to press “shift+Ctrl+Enter” for array calculations in Excel. There is another method for array calculations by using the INDEX Function. Slope is calculated using INDEX(LINEST) with the last argument equal to 1. Intercept is calculated using INDEX(LINEST) with the last argument equal to 2. Numeric calculations by Excel functions provide the same results as the scatter plot with trend lines. Both methods try to find the best-fit line that minimizes the sum of squared differences between observed and predicted values.
Step 3 is Using Data Analysis Tools for Linear Regression
Click on ‘Data analysis’ & select ‘Regression’ from the list. ‘Input Y Range’ is the dependent variable, which is the column related to ‘number of defects’ in our example. ‘Input X Range’ is the independent variable, which is the column related to QA investment. Remember to check the box for ‘labels’ as we have selected column headings. The outputs can appear in a new worksheet & we check the box for ‘Residuals’ to be calculated.
In the ‘summary output’ worksheet, regression statistics are shown, which will be interpreted in our next step.
Step 4 is Interpreting Regression Results in Excel
we can find Standard Error For our 10 observations, which is standard deviation of the error term & a measure of prediction accuracy. R Square is the coefficient of determination & measures how much of the variation in the dependent variable can be explained by the independent variable. It ranges from 0 to 1, with desirable values close to 1. Multiple R is the correlation coefficient & measures the strength & direction of the linear relationship between the dependent & independent variables. Its value ranges from -1 to 1.
Analysis of Variance or ANOVA shows some results, including df or Degrees of Freedom, which is associated with the sources of variance. Number of observations minus 1 gives the total df, which is 9 in our example. SS or Sum of Squares is a measure of variation. MS or Mean Square is SS divided by the degree of freedom. F is the test statistic for the analysis of variance. Significance F is the p-value associated with the F statistic. We can also find the intercept & slope of the fitted line under coefficients.
The residuals in the last table show the difference between model’s prediction of defects and the ground truth in each observed project.
Finally, let’s focus on Forecasting future outcomes.
We can use the best-fitting model to predict number of defects in future projects. Let’s focus on the worst-case scenario in which no investment is made on QA.
And here it is, an easy& short implementation of linear regression using only 4 simple steps in Microsoft Excel.
Комментарии