filmov
tv
Geometric Deep Learning - Understanding DL 24
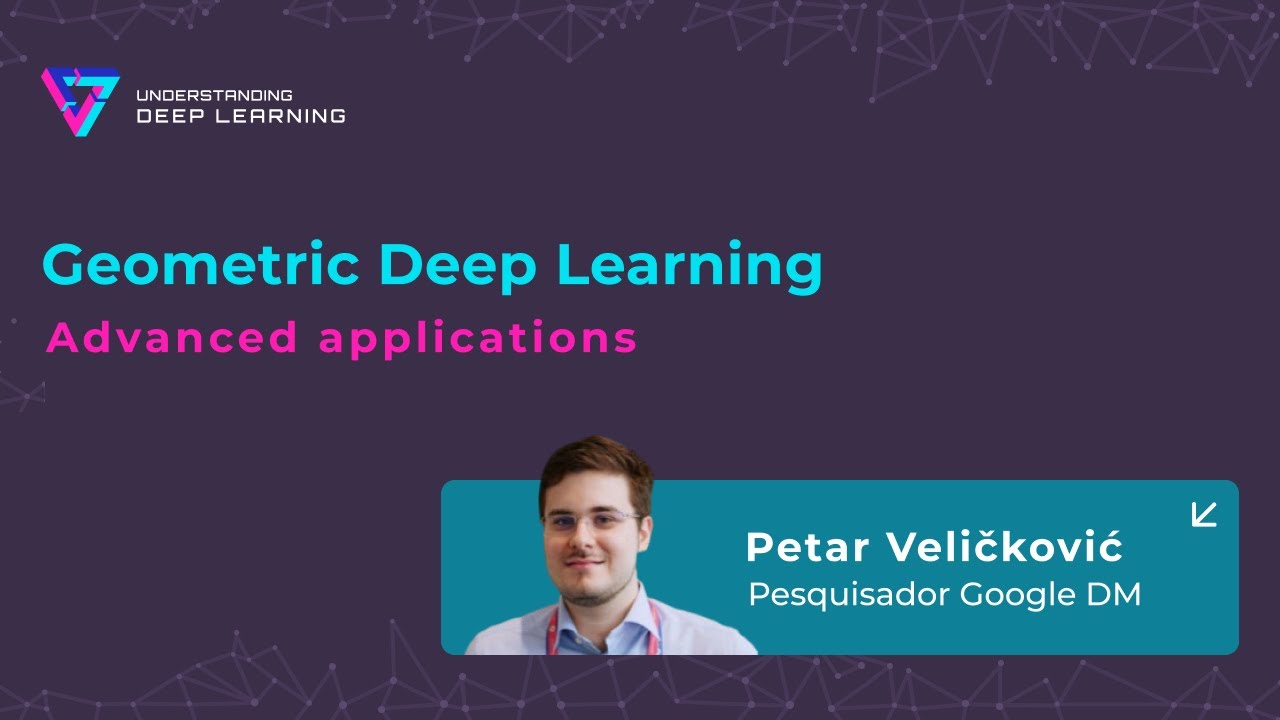
Показать описание
Speaker: Petar Veličković
Abstract:
While learning generic functions in high dimensions is a cursed estimation problem, most tasks of interest are not generic, and come with essential pre-defined regularities arising from the underlying low-dimensionality and structure of the physical world. Exploiting the known symmetries of a large system is a powerful and classical remedy against the curse of dimensionality, and forms the basis of most physical theories. Deep learning systems are no exception.
Petar Veličković is a Staff Research Scientist at Google DeepMind, Affiliated Lecturer at the University of Cambridge, and an Associate of Clare Hall, Cambridge. He holds a PhD in Computer Science from the University of Cambridge (Trinity College), obtained under the supervision of Pietro Liò. His research concerns geometric deep learning—devising neural network architectures that respect the invariances and symmetries in data (a topic he has co-written a proto-book about). He is recognised as an ELLIS Scholar in the Geometric Deep Learning Program. Particularly, he focuses on graph representation learning and its applications in algorithmic reasoning (featured in VentureBeat). He is the first author of Graph Attention Networks—a popular convolutional layer for graphs—and Deep Graph Infomax—a popular self-supervised learning pipeline for graphs (featured in ZDNet).
His research has been used in substantially improving travel-time predictions in Google Maps (featured in the CNBC, Endgadget, VentureBeat, CNET, the Verge and ZDNet), guiding intuition of mathematicians towards new top-tier theorems and conjectures (featured in Nature, Science, Quanta Magazine, New Scientist, The Independent, Sky News, The Sunday Times, la Repubblica and The Conversation), and the first full AI system for tactical suggestions in association football (featured in Financial Times, The Economist, New Scientist, Wired, the Verge and El País).
Abstract:
While learning generic functions in high dimensions is a cursed estimation problem, most tasks of interest are not generic, and come with essential pre-defined regularities arising from the underlying low-dimensionality and structure of the physical world. Exploiting the known symmetries of a large system is a powerful and classical remedy against the curse of dimensionality, and forms the basis of most physical theories. Deep learning systems are no exception.
Petar Veličković is a Staff Research Scientist at Google DeepMind, Affiliated Lecturer at the University of Cambridge, and an Associate of Clare Hall, Cambridge. He holds a PhD in Computer Science from the University of Cambridge (Trinity College), obtained under the supervision of Pietro Liò. His research concerns geometric deep learning—devising neural network architectures that respect the invariances and symmetries in data (a topic he has co-written a proto-book about). He is recognised as an ELLIS Scholar in the Geometric Deep Learning Program. Particularly, he focuses on graph representation learning and its applications in algorithmic reasoning (featured in VentureBeat). He is the first author of Graph Attention Networks—a popular convolutional layer for graphs—and Deep Graph Infomax—a popular self-supervised learning pipeline for graphs (featured in ZDNet).
His research has been used in substantially improving travel-time predictions in Google Maps (featured in the CNBC, Endgadget, VentureBeat, CNET, the Verge and ZDNet), guiding intuition of mathematicians towards new top-tier theorems and conjectures (featured in Nature, Science, Quanta Magazine, New Scientist, The Independent, Sky News, The Sunday Times, la Repubblica and The Conversation), and the first full AI system for tactical suggestions in association football (featured in Financial Times, The Economist, New Scientist, Wired, the Verge and El País).