filmov
tv
ICLR 2021 Keynote - 'Geometric Deep Learning: The Erlangen Programme of ML' - M Bronstein
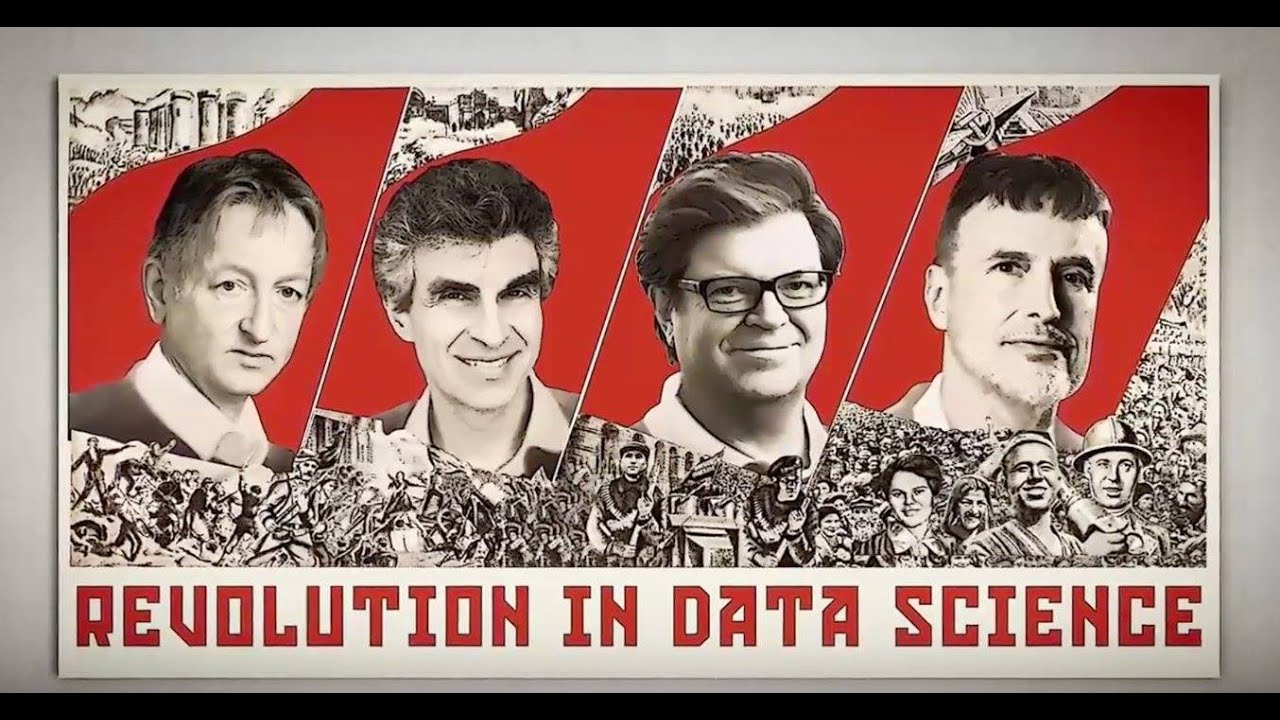
Показать описание
Geometric Deep Learning: The Erlangen Programme of ML - ICLR 2021 Keynote by Michael Bronstein (Imperial College London / IDSIA / Twitter)
“Symmetry, as wide or as narrow as you may define its meaning, is one idea by which man through the ages has tried to comprehend and create order, beauty, and perfection.” This poetic definition comes from the great mathematician Hermann Weyl, credited with laying the foundation of our modern theory of the universe. Another great physicist, Philip Anderson, said that "it is only slightly overstating the case to say that physics is the study of symmetry."
In mathematics, symmetry was crucial in the foundation of geometry as we know it in the 19th century. Now it could have a similar impact on another emerging field. Deep Learning success in recent decades is significant – from revolutionising data science to landmark achievements in computer vision, board games, and protein folding. At the same time, a lack of unifying principles makes it is difficult to understand the relations between different neural network architectures resulting in the reinvention and re-branding of the same concepts.
Michael Bronstein is a professor at Imperial College London and Head of Graph ML Research at Twitter, who is working to bring geometric unification of deep learning through the lens of symmetry. In his ICLR 2021 keynote lecture, he presents a common mathematical framework to study the most successful network architectures, giving a constructive procedure to build future machine learning in a principled way that could be applied in new domains such as social science, biology, and drug design.
Animation: Jakub Makowski
“Symmetry, as wide or as narrow as you may define its meaning, is one idea by which man through the ages has tried to comprehend and create order, beauty, and perfection.” This poetic definition comes from the great mathematician Hermann Weyl, credited with laying the foundation of our modern theory of the universe. Another great physicist, Philip Anderson, said that "it is only slightly overstating the case to say that physics is the study of symmetry."
In mathematics, symmetry was crucial in the foundation of geometry as we know it in the 19th century. Now it could have a similar impact on another emerging field. Deep Learning success in recent decades is significant – from revolutionising data science to landmark achievements in computer vision, board games, and protein folding. At the same time, a lack of unifying principles makes it is difficult to understand the relations between different neural network architectures resulting in the reinvention and re-branding of the same concepts.
Michael Bronstein is a professor at Imperial College London and Head of Graph ML Research at Twitter, who is working to bring geometric unification of deep learning through the lens of symmetry. In his ICLR 2021 keynote lecture, he presents a common mathematical framework to study the most successful network architectures, giving a constructive procedure to build future machine learning in a principled way that could be applied in new domains such as social science, biology, and drug design.
Animation: Jakub Makowski
Комментарии