filmov
tv
Deriving the Normal Distribution Probability Density Function Formula
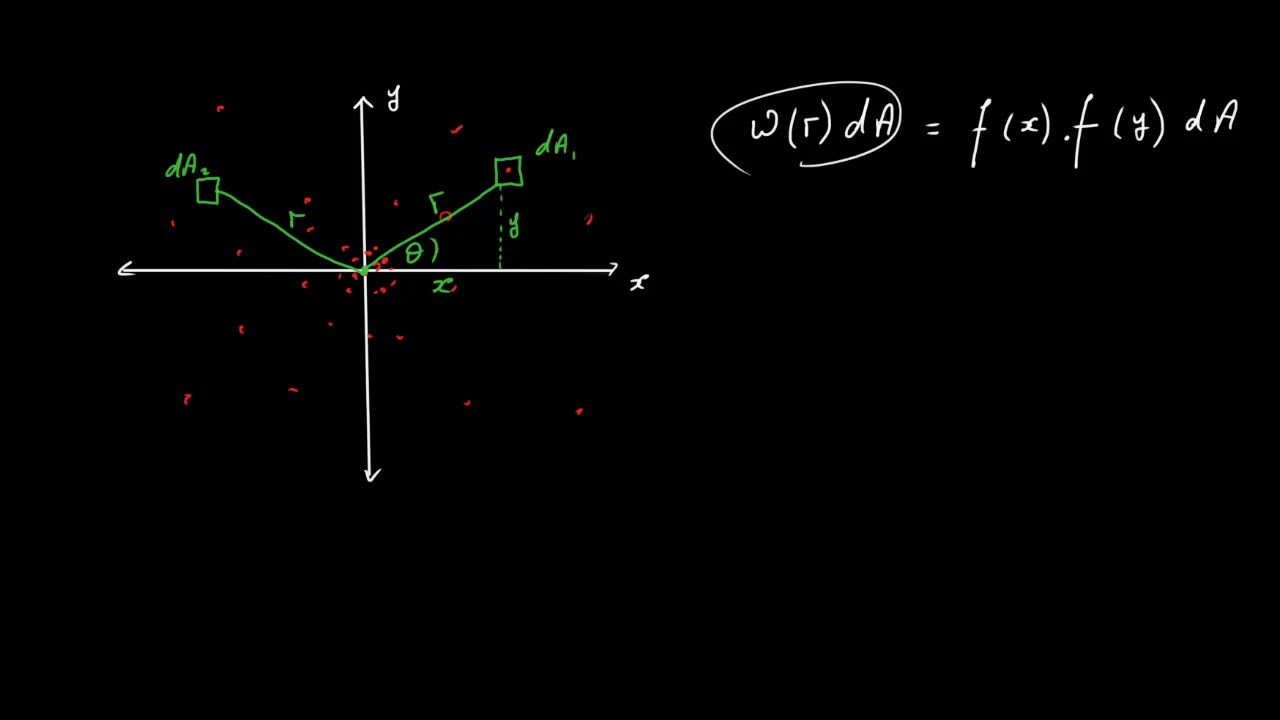
Показать описание
Normal distribution's probability density function derived in 5min
Deriving the Normal Distribution Probability Density Function Formula
Why π is in the normal distribution (beyond integral tricks)
Normal Distribution EXPLAINED with Examples
The Normal Distribution, Clearly Explained!!!
Probability Density Function of the Normal Distribution
Normal Distribution & Probability Problems
But what is the Central Limit Theorem?
Using the Normal Distribution with Excel
An Introduction to the Normal Distribution
How do you DERIVE the BELL CURVE?
Normal Distribution: Calculating Probabilities/Areas (z-table)
Normal prior Normal likelihood Normal posterior distribution
Intro to the Normal Distribution and how to Calculate Probability
Standard Normal Distribution Tables, Z Scores, Probability & Empirical Rule - Stats
Normal Distribution: Probability Density Function Derivation
Proof: Integral of PDF of Normal Distribution is Equal to 1 (in English)
Binomial distributions | Probabilities of probabilities, part 1
Normal Distribution|Probability Distribution|Statistics|BBA|BCA|BCOM|Dream Maths
Introduction to the normal distribution | Probability and Statistics | Khan Academy
Deriving the Mean and Variance of a Continuous Probability Distribution
The Bell Curve (Normal/Gaussian Distribution) Explained in One Minute: From Definition to Examples
Normal Distribution | Mean and Variance of Normal Distribution
Mod-01 Lec-06 Normal probability distribution
Комментарии