filmov
tv
Create a Large Language Model from Scratch with Python – Tutorial
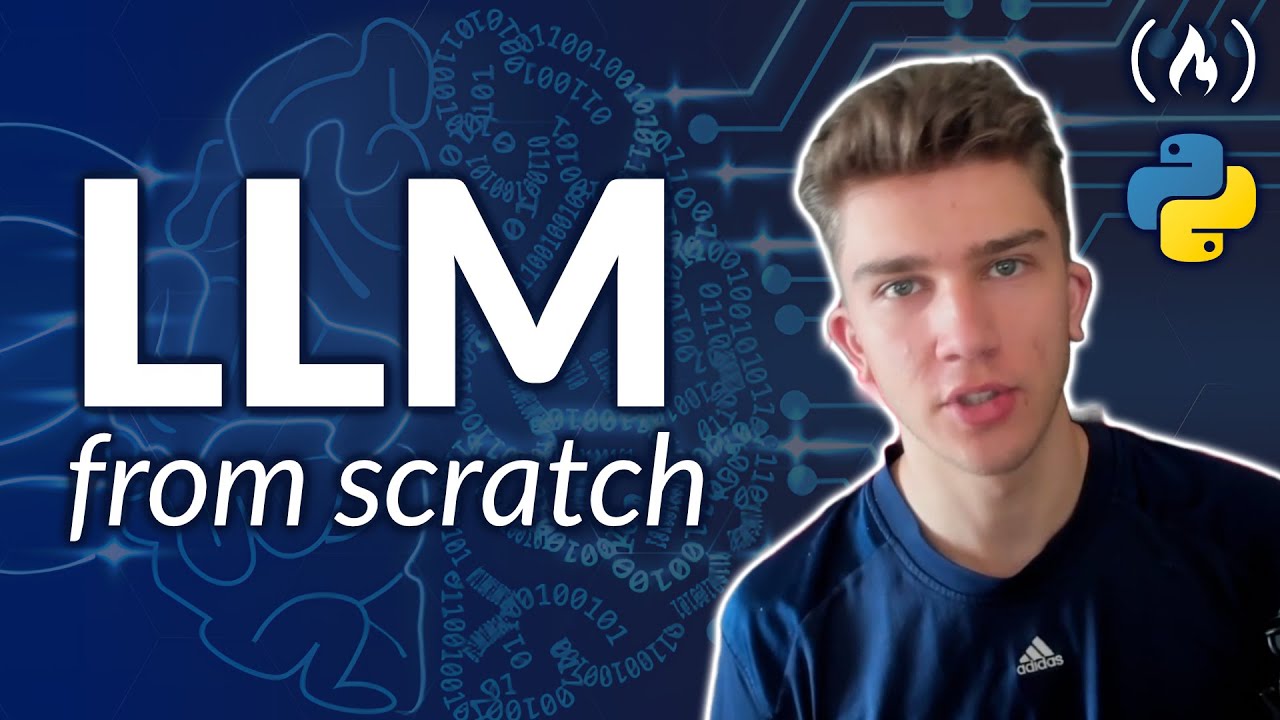
Показать описание
Learn how to build your own large language model, from scratch. This course goes into the data handling, math, and transformers behind large language models. You will use Python.
✏️ Course developed by @elliotarledge
⭐️ Contents ⭐️
(0:00:00) Intro
(0:03:25) Install Libraries
(0:06:24) Pylzma build tools
(0:08:58) Jupyter Notebook
(0:12:11) Download wizard of oz
(0:14:51) Experimenting with text file
(0:17:58) Character-level tokenizer
(0:19:44) Types of tokenizers
(0:20:58) Tensors instead of Arrays
(0:22:37) Linear Algebra heads up
(0:23:29) Train and validation splits
(0:25:30) Premise of Bigram Model
(0:26:41) Inputs and Targets
(0:29:29) Inputs and Targets Implementation
(0:30:10) Batch size hyperparameter
(0:32:13) Switching from CPU to CUDA
(0:33:28) PyTorch Overview
(0:42:49) CPU vs GPU performance in PyTorch
(0:47:49) More PyTorch Functions
(1:06:03) Embedding Vectors
(1:11:33) Embedding Implementation
(1:13:06) Dot Product and Matrix Multiplication
(1:25:42) Matmul Implementation
(1:26:56) Int vs Float
(1:29:52) Recap and get_batch
(1:35:07) nnModule subclass
(1:37:05) Gradient Descent
(1:50:53) Logits and Reshaping
(1:59:28) Generate function and giving the model some context
(2:03:58) Logits Dimensionality
(2:05:17) Training loop + Optimizer + Zerograd explanation
(2:13:56) Optimizers Overview
(2:17:04) Applications of Optimizers
(2:18:11) Loss reporting + Train VS Eval mode
(2:32:54) Normalization Overview
(2:35:45) ReLU, Sigmoid, Tanh Activations
(2:45:15) Transformer and Self-Attention
(2:46:55) Transformer Architecture
(3:17:54) Building a GPT, not Transformer model
(3:19:46) Self-Attention Deep Dive
(3:25:05) GPT architecture
(3:27:07) Switching to Macbook
(3:31:42) Implementing Positional Encoding
(3:36:57) GPTLanguageModel initalization
(3:40:52) GPTLanguageModel forward pass
(3:46:56) Standard Deviation for model parameters
(4:00:50) Transformer Blocks
(4:04:54) FeedForward network
(4:07:53) Multi-head Attention
(4:12:49) Dot product attention
(4:19:43) Why we scale by 1/sqrt(dk)
(4:26:45) Sequential VS ModuleList Processing
(4:30:47) Overview Hyperparameters
(4:32:14) Fixing errors, refining
(4:34:01) Begin training
(4:35:46) OpenWebText download and Survey of LLMs paper
(4:37:56) How the dataloader/batch getter will have to change
(4:41:20) Extract corpus with winrar
(4:43:44) Python data extractor
(4:49:23) Adjusting for train and val splits
(4:57:55) Adding dataloader
(4:59:04) Training on OpenWebText
(5:02:22) Training works well, model loading/saving
(5:04:18) Pickling
(5:05:32) Fixing errors + GPU Memory in task manager
(5:14:05) Command line argument parsing
(5:18:11) Porting code to script
(5:22:04) Prompt: Completion feature + more errors
(5:24:23) nnModule inheritance + generation cropping
(5:27:54) Pretraining vs Finetuning
(5:33:07) R&D pointers
(5:44:38) Outro
🎉 Thanks to our Champion and Sponsor supporters:
👾 davthecoder
👾 jedi-or-sith
👾 南宮千影
👾 Agustín Kussrow
👾 Nattira Maneerat
👾 Heather Wcislo
👾 Serhiy Kalinets
👾 Justin Hual
👾 Otis Morgan
--
✏️ Course developed by @elliotarledge
⭐️ Contents ⭐️
(0:00:00) Intro
(0:03:25) Install Libraries
(0:06:24) Pylzma build tools
(0:08:58) Jupyter Notebook
(0:12:11) Download wizard of oz
(0:14:51) Experimenting with text file
(0:17:58) Character-level tokenizer
(0:19:44) Types of tokenizers
(0:20:58) Tensors instead of Arrays
(0:22:37) Linear Algebra heads up
(0:23:29) Train and validation splits
(0:25:30) Premise of Bigram Model
(0:26:41) Inputs and Targets
(0:29:29) Inputs and Targets Implementation
(0:30:10) Batch size hyperparameter
(0:32:13) Switching from CPU to CUDA
(0:33:28) PyTorch Overview
(0:42:49) CPU vs GPU performance in PyTorch
(0:47:49) More PyTorch Functions
(1:06:03) Embedding Vectors
(1:11:33) Embedding Implementation
(1:13:06) Dot Product and Matrix Multiplication
(1:25:42) Matmul Implementation
(1:26:56) Int vs Float
(1:29:52) Recap and get_batch
(1:35:07) nnModule subclass
(1:37:05) Gradient Descent
(1:50:53) Logits and Reshaping
(1:59:28) Generate function and giving the model some context
(2:03:58) Logits Dimensionality
(2:05:17) Training loop + Optimizer + Zerograd explanation
(2:13:56) Optimizers Overview
(2:17:04) Applications of Optimizers
(2:18:11) Loss reporting + Train VS Eval mode
(2:32:54) Normalization Overview
(2:35:45) ReLU, Sigmoid, Tanh Activations
(2:45:15) Transformer and Self-Attention
(2:46:55) Transformer Architecture
(3:17:54) Building a GPT, not Transformer model
(3:19:46) Self-Attention Deep Dive
(3:25:05) GPT architecture
(3:27:07) Switching to Macbook
(3:31:42) Implementing Positional Encoding
(3:36:57) GPTLanguageModel initalization
(3:40:52) GPTLanguageModel forward pass
(3:46:56) Standard Deviation for model parameters
(4:00:50) Transformer Blocks
(4:04:54) FeedForward network
(4:07:53) Multi-head Attention
(4:12:49) Dot product attention
(4:19:43) Why we scale by 1/sqrt(dk)
(4:26:45) Sequential VS ModuleList Processing
(4:30:47) Overview Hyperparameters
(4:32:14) Fixing errors, refining
(4:34:01) Begin training
(4:35:46) OpenWebText download and Survey of LLMs paper
(4:37:56) How the dataloader/batch getter will have to change
(4:41:20) Extract corpus with winrar
(4:43:44) Python data extractor
(4:49:23) Adjusting for train and val splits
(4:57:55) Adding dataloader
(4:59:04) Training on OpenWebText
(5:02:22) Training works well, model loading/saving
(5:04:18) Pickling
(5:05:32) Fixing errors + GPU Memory in task manager
(5:14:05) Command line argument parsing
(5:18:11) Porting code to script
(5:22:04) Prompt: Completion feature + more errors
(5:24:23) nnModule inheritance + generation cropping
(5:27:54) Pretraining vs Finetuning
(5:33:07) R&D pointers
(5:44:38) Outro
🎉 Thanks to our Champion and Sponsor supporters:
👾 davthecoder
👾 jedi-or-sith
👾 南宮千影
👾 Agustín Kussrow
👾 Nattira Maneerat
👾 Heather Wcislo
👾 Serhiy Kalinets
👾 Justin Hual
👾 Otis Morgan
--
Комментарии