filmov
tv
Why Large Language Models Hallucinate
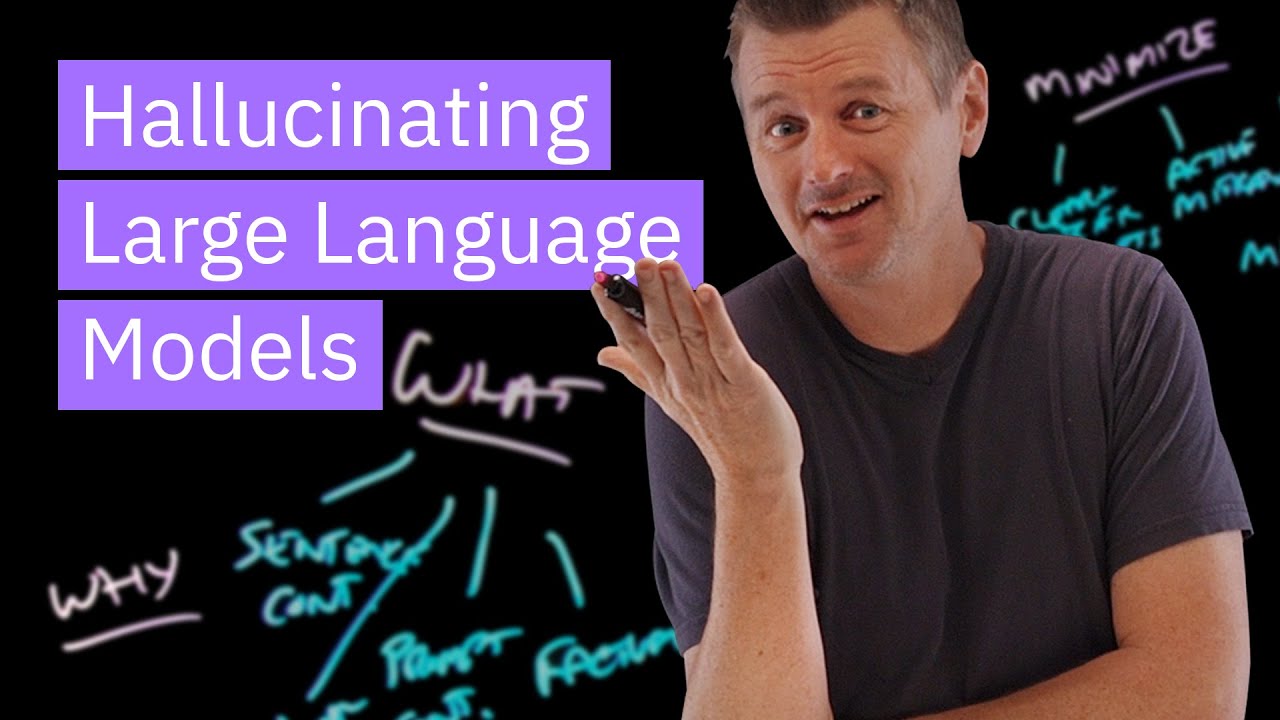
Показать описание
Large language models (LLMs) like chatGPT can generate authoritative-sounding prose on many topics and domains, they are also prone to just "make stuff up". Literally plausible sounding nonsense! In this video, Martin Keen explains the different types of "LLMs hallucinations", why they happen, and ends with recommending steps that you, as a LLM user, can take to minimize their occurrence.
#AI #Software #Dev #lightboard #IBM #MartinKeen #llm
Why Large Language Models Hallucinate
Why LLMs hallucinate | Yann LeCun and Lex Fridman
Ai hallucinations explained
Why Language Models Hallucinate
Risks of Large Language Models (LLM)
LLM Limitations and Hallucinations
LLM hallucinations explained | Marc Andreessen and Lex Fridman
Ai Hallucinations Explained in Non Nerd English
Tech-Talk: The Evolution of AI in Marketing
Hallucinations in Large Language Models (LLMs)
Hallucination in Large Language Models (LLMs)
Why do LLMs | Large Language Models Hallucinate
Grounding AI Explained: How to stop AI hallucinations
Why large language models hallucinate #datascience #machinelearning #chatgpt #llama2 #deeplearning
How do we prevent AI hallucinations
ChatGPT Hallucinations and Why Large Language Models Hallucinate | False Information | Google OpenAI
Understanding Large Language Model Hallucinations
Why Large Language Models Hallucinate?
Reducing Hallucinations in LLMs | Retrieval QA w/ LangChain + Ray + Weights & Biases
Ep 6. Conquer LLM Hallucinations with an Evaluation Framework
Why Does AI Hallucinate?
Ray Kurzweil on LLM hallucinations
Stopping Hallucinations From Hurting Your LLMs // Atindriyo Sanyal // LLMs in Prod Conference Part 2
10 Limitations of Large Language Models: Dealing Hallucinations, Stale Data and Beyond
Комментарии