filmov
tv
Multiple Imputation and Checking Regression Assumptions - What Data Should We Use?
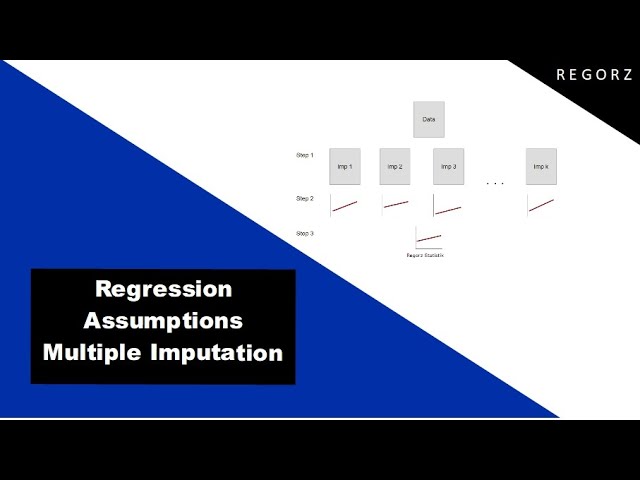
Показать описание
If you use multiple imputation for missing data in a regression analysis you have to check the regression assumptions (e.g. normality, homoskedasticity, linearity). This tutorial explains what data (cases with complete data or imputed datasets) you should use for the assumption checks in a linear regression with multiple imputation, depending on the missing data mechanism (missing completely at random, missing at random).
Here is a text version of this tutorial complete with references:
Regorz, A. (2022). Checking regression assumptions when using multiple imputation for missing
data (Issues in Applied Statistics 1/22). Regorz Statistik.
Here are two Youtube videos explaining the missing data mechanisms (MCAR, MAR, MNAR):
Here is a text version of this tutorial complete with references:
Regorz, A. (2022). Checking regression assumptions when using multiple imputation for missing
data (Issues in Applied Statistics 1/22). Regorz Statistik.
Here are two Youtube videos explaining the missing data mechanisms (MCAR, MAR, MNAR):
Multiple Imputation and Checking Regression Assumptions - What Data Should We Use?
R: Regression With Multiple Imputation (missing data handling)
Multiple imputation in Stata®: Linear regression
Dealing With Missing Data - Multiple Imputation
How to Use SPSS-Replacing Missing Data Using Multiple Imputation (Regression Method)
Multiple imputation in Stata®: Logistic regression
Missing Data Analysis: Multiple Imputation and Maximum Likelihood Methods
Missing Data? No Problem!
Missing value analysis in R using multiple imputation
Multiple imputation
Lab 6 (Part 1c) Multiple Imputation and Steps for addressing missing data imputation
How to impute missing data using mice package in R programming
Regression mit Multipler Imputation in R (für fehlende Werte)
Regressionsannahmen prüfen bei multipler Imputation - mit welchen Daten?
Missing Data SPSS Tutorial
Missing Data & Multiple Imputation
Imputation of missing data - Multiple imputation using SPSS
Understanding multiple imputations
Workflow for multiple imputation analysis
Handle Missing Values: Imputation using R ('mice') Explained
20_3 data imputation Regression imputation
Online Lecture #18.1: Missing Data and Multiple Imputation
Understanding missing data and missing values. 5 ways to deal with missing data using R programming
Handling Missing Data in Stata
Комментарии