filmov
tv
python tensorflow with cuda
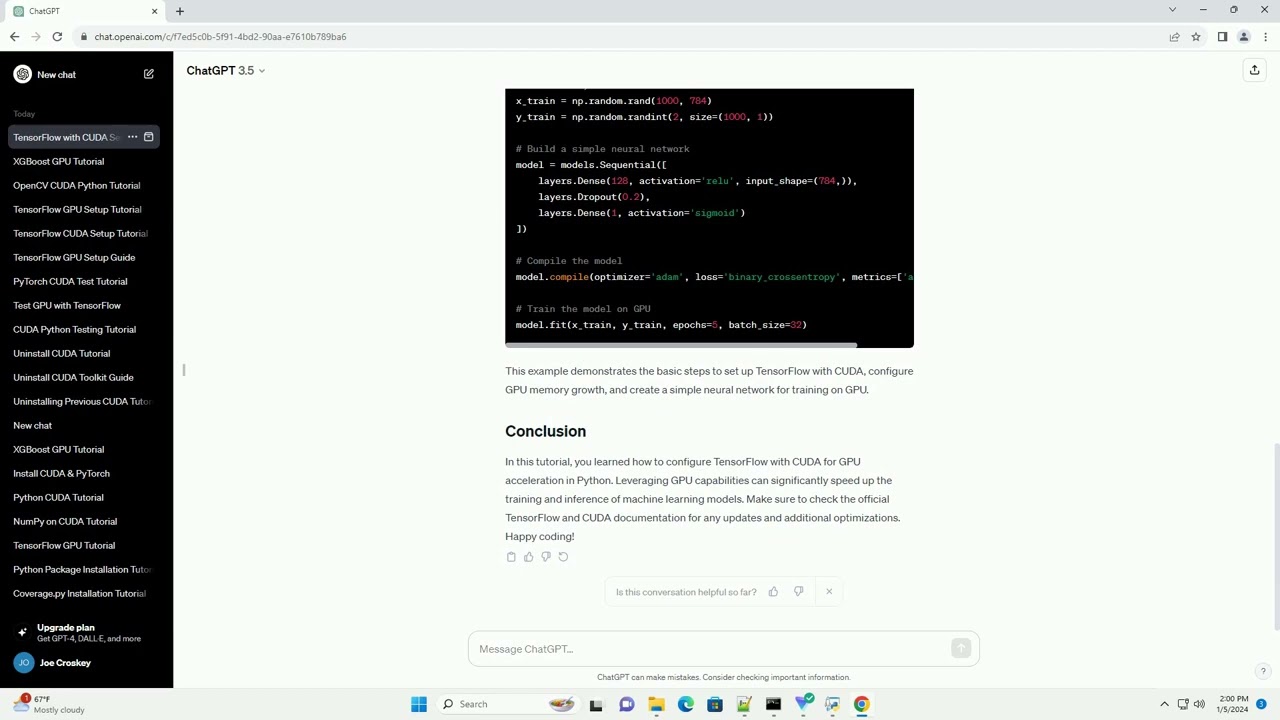
Показать описание
TensorFlow is an open-source machine learning framework developed by the Google Brain team. It provides a comprehensive set of tools for building and deploying machine learning models. CUDA is a parallel computing platform and programming model developed by NVIDIA for GPU acceleration. In this tutorial, we will walk through the process of setting up TensorFlow with CUDA to leverage GPU capabilities for faster training and inference.
NVIDIA GPU: Ensure that you have an NVIDIA GPU that supports CUDA. You can check the official CUDA-enabled GPU list to verify your GPU's compatibility.
NVIDIA CUDA Toolkit: Download and install the NVIDIA CUDA Toolkit on your machine. Follow the installation instructions provided for your operating system.
cuDNN: Download and install the cuDNN library from NVIDIA. cuDNN is a GPU-accelerated library for deep neural networks.
TensorFlow: Install TensorFlow and its dependencies using pip:
Check CUDA Version:
Verify that TensorFlow can detect your CUDA installation:
If the first print statement returns True, and the second one displays your GPU name, TensorFlow is configured to use CUDA.
GPU Memory Growth:
By default, TensorFlow allocates all GPU memory when the program starts. You can enable GPU memory growth to allocate memory as needed:
Let's create a simple example to demonstrate TensorFlow with CUDA. We'll build a basic neural network and train it on a synthetic dataset.
This example demonstrates the basic steps to set up TensorFlow with CUDA, configure GPU memory growth, and create a simple neural network for training on GPU.
In this tutorial, you learned how to configure TensorFlow with CUDA for GPU acceleration in Python. Leveraging GPU capabilities can significantly speed up the training and inference of machine learning models. Make sure to check the official TensorFlow and CUDA documentation for any updates and additional optimizations. Happy coding!
ChatGPT
NVIDIA GPU: Ensure that you have an NVIDIA GPU that supports CUDA. You can check the official CUDA-enabled GPU list to verify your GPU's compatibility.
NVIDIA CUDA Toolkit: Download and install the NVIDIA CUDA Toolkit on your machine. Follow the installation instructions provided for your operating system.
cuDNN: Download and install the cuDNN library from NVIDIA. cuDNN is a GPU-accelerated library for deep neural networks.
TensorFlow: Install TensorFlow and its dependencies using pip:
Check CUDA Version:
Verify that TensorFlow can detect your CUDA installation:
If the first print statement returns True, and the second one displays your GPU name, TensorFlow is configured to use CUDA.
GPU Memory Growth:
By default, TensorFlow allocates all GPU memory when the program starts. You can enable GPU memory growth to allocate memory as needed:
Let's create a simple example to demonstrate TensorFlow with CUDA. We'll build a basic neural network and train it on a synthetic dataset.
This example demonstrates the basic steps to set up TensorFlow with CUDA, configure GPU memory growth, and create a simple neural network for training on GPU.
In this tutorial, you learned how to configure TensorFlow with CUDA for GPU acceleration in Python. Leveraging GPU capabilities can significantly speed up the training and inference of machine learning models. Make sure to check the official TensorFlow and CUDA documentation for any updates and additional optimizations. Happy coding!
ChatGPT