filmov
tv
David Duvenaud | Explaining decisions by generating counterfactuals
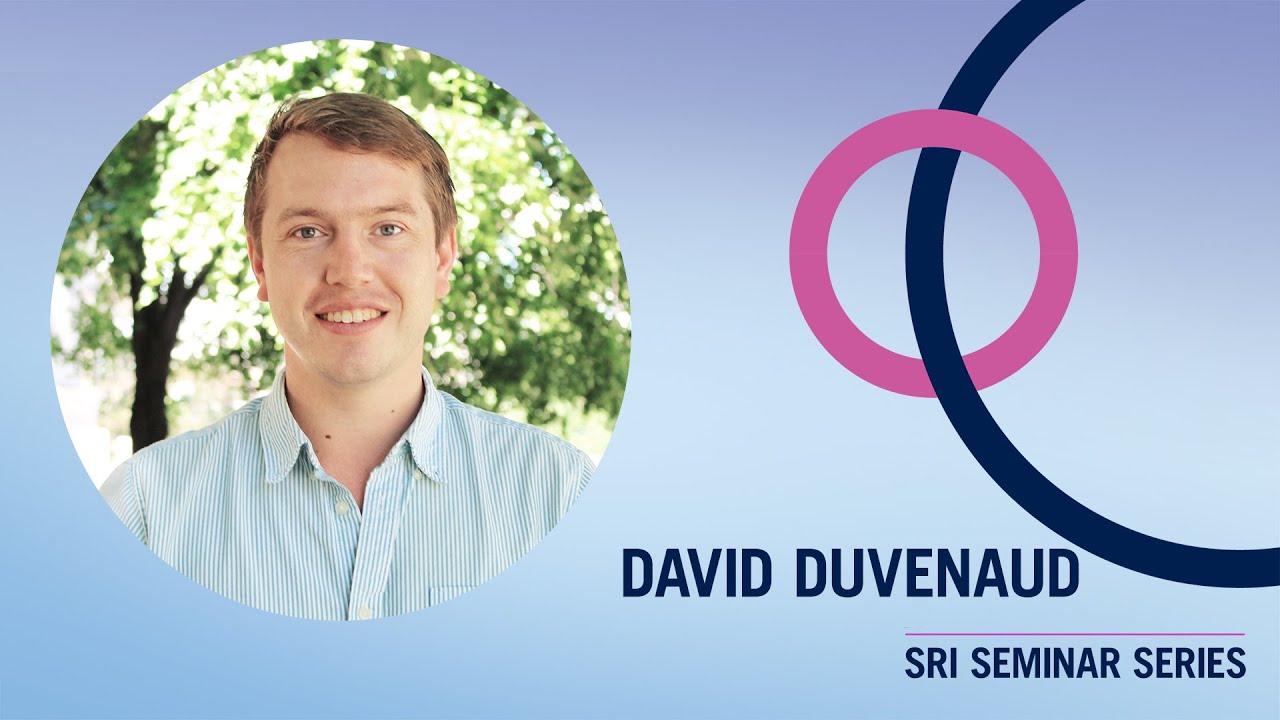
Показать описание
Our weekly SRI Seminar Series welcomes David Duvenaud, an assistant professor in computer science and statistics at the University of Toronto. Duvenaud holds a Canada Research Chair in generative models, and is a founding member of the Vector Institute. Duvenaud’s research is focused on hyperparameter optimization, variational inference, deep learning, and automatic chemical design. He previously worked as part of the machine vision team at Google Research, and also co-founded Invenia, an energy forecasting and trading company.
In this talk, Duvenaud will explore how deep generative computer models can be used to provide explanations for the decisions and predictions of machine learning (ML) systems. As ML systems are increasingly used in various social contexts with a wide range of applications, questions of explainability have become central to ensuring their methods can be interpreted and understood, and that the outcomes they generate are fair and equitable.
Talk title:
“Explaining decisions by generating counterfactuals”
Abstract:
This will be a high-level talk, with concrete examples, about using deep generative models to explain the decisions and predictions of machine learning models. For example, when an image classifier makes a prediction, which parts of the image were relevant and why? We argue that answering this question requires considering images that could have been seen but weren't. Machine learning has recently gained the ability to generate plausible completions of images and other types of data, letting us generate detailed counterfactual examples on a case-by-case basis. We'll show prototype applications of this approach to image classifiers, and predictions of medical outcomes. Finally, we'll talk about the open problem of automatically communicating about abstract aspects of a situation.
About David Duvenaud
David Duvenaud is an assistant professor in computer science and statistics at the University of Toronto, and holds a Canada Research Chair in generative models. He is also a founding member of the Vector Institute. Duvenaud’s postdoc was at Harvard University, where he worked on hyperparameter optimization, variational inference, deep learning, and automatic chemical design. He did his PhD at the University of Cambridge, studying Bayesian nonparametrics with Zoubin Ghahramani and Carl Rasmussen. Duvenaud has worked with the machine vision team at Google Research, and also co-founded Invenia, an energy forecasting and trading company.
About the SRI Seminar Series
The SRI Seminar Series brings together the Schwartz Reisman community and beyond for a robust exchange of ideas that advance scholarship at the intersection of technology and society. Seminars are led by a leading or emerging scholar and feature extensive discussion.
In this talk, Duvenaud will explore how deep generative computer models can be used to provide explanations for the decisions and predictions of machine learning (ML) systems. As ML systems are increasingly used in various social contexts with a wide range of applications, questions of explainability have become central to ensuring their methods can be interpreted and understood, and that the outcomes they generate are fair and equitable.
Talk title:
“Explaining decisions by generating counterfactuals”
Abstract:
This will be a high-level talk, with concrete examples, about using deep generative models to explain the decisions and predictions of machine learning models. For example, when an image classifier makes a prediction, which parts of the image were relevant and why? We argue that answering this question requires considering images that could have been seen but weren't. Machine learning has recently gained the ability to generate plausible completions of images and other types of data, letting us generate detailed counterfactual examples on a case-by-case basis. We'll show prototype applications of this approach to image classifiers, and predictions of medical outcomes. Finally, we'll talk about the open problem of automatically communicating about abstract aspects of a situation.
About David Duvenaud
David Duvenaud is an assistant professor in computer science and statistics at the University of Toronto, and holds a Canada Research Chair in generative models. He is also a founding member of the Vector Institute. Duvenaud’s postdoc was at Harvard University, where he worked on hyperparameter optimization, variational inference, deep learning, and automatic chemical design. He did his PhD at the University of Cambridge, studying Bayesian nonparametrics with Zoubin Ghahramani and Carl Rasmussen. Duvenaud has worked with the machine vision team at Google Research, and also co-founded Invenia, an energy forecasting and trading company.
About the SRI Seminar Series
The SRI Seminar Series brings together the Schwartz Reisman community and beyond for a robust exchange of ideas that advance scholarship at the intersection of technology and society. Seminars are led by a leading or emerging scholar and feature extensive discussion.