filmov
tv
Using Artificial Intelligence to automate the languages of discovery
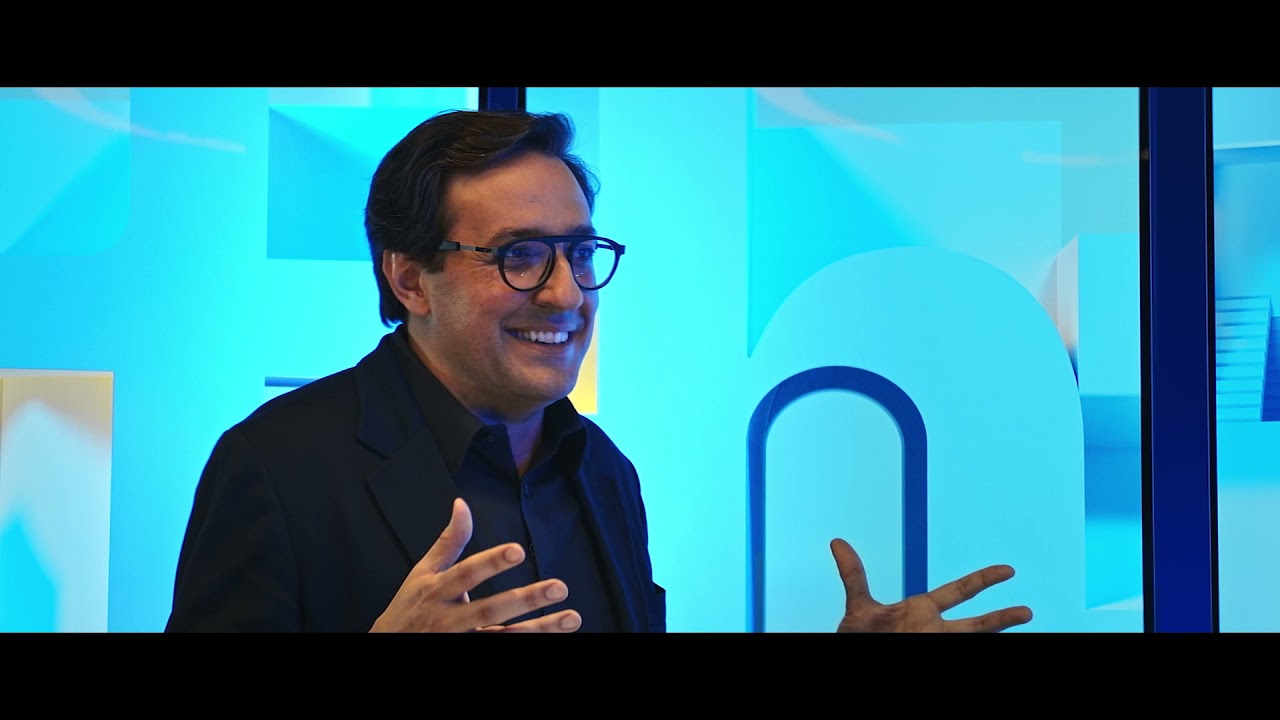
Показать описание
Since the field got started in the 1950s, the journey for AI has been a long and winding one. It's been both promising and confusing and, at times, under-hyped, as well as over-hyped. The grand challenge of AI has long been to create a system that can truly understand human language, not mimic, but understand, reason, and learn from human language. It is an arduous task and one that still challenges us today. But the pace at which we have made progress has been vastly accelerated through new methods such as deep learning.
But what if we turn these powerful new AI tools to look at languages in a completely different way? You see, languages have been the cornerstone of human progress since the beginning. And although we're not the only species to communicate through audible languages, we are, however, the only species that has taken the next step of abstracting that language into symbols. All around us are the symbols of language, from the typographic characters of our spoken language to the numeric symbols representing the properties of mathematics to the diagrammatic maps displaying the chemistry and arrangement of molecules.
Languages surround us. They help us think and reason, then allow us to share those thoughts with others. Now we have seen the power of AI applied to human language. Its importance and pervasiveness is undeniable, but at IBM research, we are also exploring how to teach AI two new languages and their exciting implications, the language of code and the language of chemistry.
AI for code technology is going to fundamentally change how we think about coding. We built an AI model that helped us in a very short amount of time to comb through all the code in an application. The AI model helps us not only identify just which parts of the code are obsolete or no longer in use, which parts of code are redundant, and also which parts of code can be grouped in better, more manageable groups of code or other microservices.
With Project CodeNet, we've made the over 14 million samples of code available as part of the open-source dataset available on GitHub. Our team has extracted the most representative code samples that can help us or help AI train and better help the developers provide the software.
From learning about the implications of teaching AI the language of code, we also demonstrate how AI is learning a different language. This time, the language of chemistry.
We have also been able to teach the language of chemistry to the AI architecture. And the result has been a way of accelerating discovery for designing new materials that, instead of taking years and a millions dollars budget, can now be designed and synthesized in weeks or months.
These are two powerful examples of how IBM Research is advancing the state of the art in AI, and reimagining how teaching AI new languages will accelerate software productivity and scientific discovery.
Language, automation, and trust are the three pillars of IBM's AI strategy. With innovation from IBM Research now being integrated into IBM's Watson AI portfolio, faster than we've ever done, this is the time to scale trusted AI for business.
But what if we turn these powerful new AI tools to look at languages in a completely different way? You see, languages have been the cornerstone of human progress since the beginning. And although we're not the only species to communicate through audible languages, we are, however, the only species that has taken the next step of abstracting that language into symbols. All around us are the symbols of language, from the typographic characters of our spoken language to the numeric symbols representing the properties of mathematics to the diagrammatic maps displaying the chemistry and arrangement of molecules.
Languages surround us. They help us think and reason, then allow us to share those thoughts with others. Now we have seen the power of AI applied to human language. Its importance and pervasiveness is undeniable, but at IBM research, we are also exploring how to teach AI two new languages and their exciting implications, the language of code and the language of chemistry.
AI for code technology is going to fundamentally change how we think about coding. We built an AI model that helped us in a very short amount of time to comb through all the code in an application. The AI model helps us not only identify just which parts of the code are obsolete or no longer in use, which parts of code are redundant, and also which parts of code can be grouped in better, more manageable groups of code or other microservices.
With Project CodeNet, we've made the over 14 million samples of code available as part of the open-source dataset available on GitHub. Our team has extracted the most representative code samples that can help us or help AI train and better help the developers provide the software.
From learning about the implications of teaching AI the language of code, we also demonstrate how AI is learning a different language. This time, the language of chemistry.
We have also been able to teach the language of chemistry to the AI architecture. And the result has been a way of accelerating discovery for designing new materials that, instead of taking years and a millions dollars budget, can now be designed and synthesized in weeks or months.
These are two powerful examples of how IBM Research is advancing the state of the art in AI, and reimagining how teaching AI new languages will accelerate software productivity and scientific discovery.
Language, automation, and trust are the three pillars of IBM's AI strategy. With innovation from IBM Research now being integrated into IBM's Watson AI portfolio, faster than we've ever done, this is the time to scale trusted AI for business.