filmov
tv
Mask R-CNN on Custom Dataset | Practical Implementation
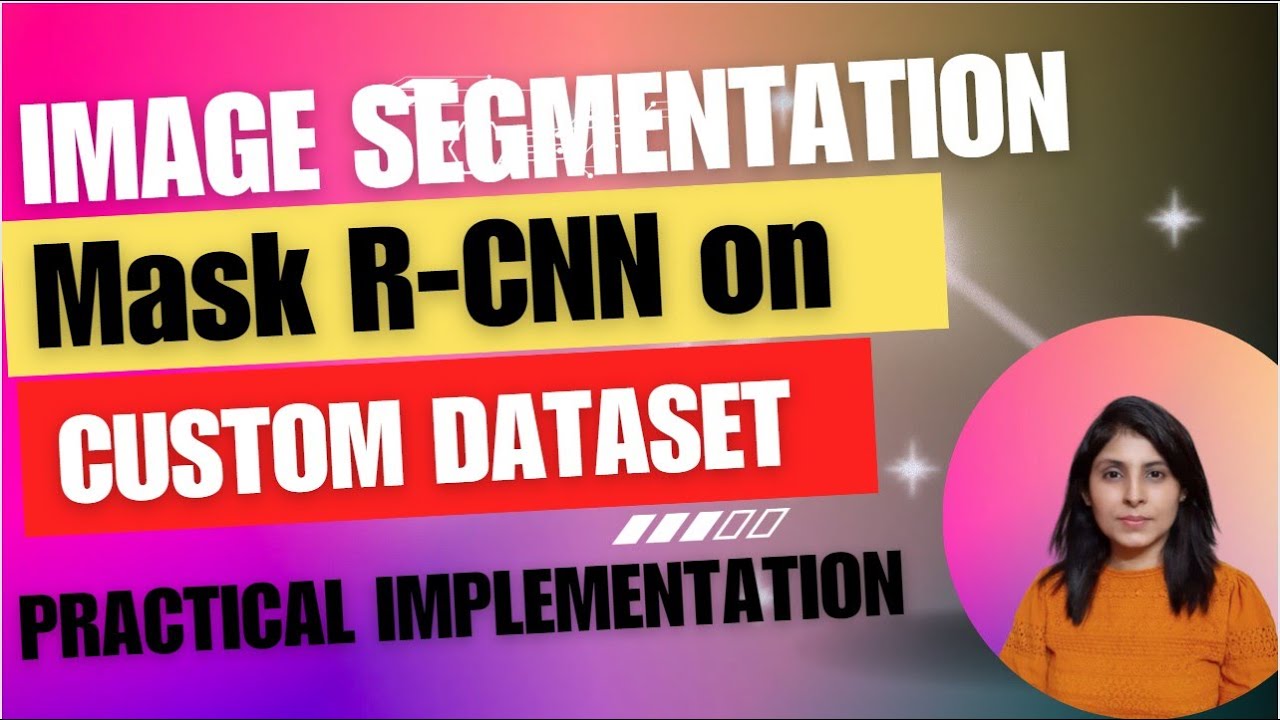
Показать описание
In this video, I have explained step by step how to train Mask R-CNN on Custom Dataset.
If you have any questions with what we covered in this video then feel free to ask in the comment section below & I'll do my best to answer your queries.
Please consider clicking the SUBSCRIBE button to be notified for future videos & thank you all for watching.
Support my channel 🙏 by LIKE ,SHARE & SUBSCRIBE
Instance Segmentation with Mask R-CNN:
Mask RCNN is a deep neural network aimed to solve instance segmentation problem in machine learning or computer vision. In other words, it can separate different objects in a image or a video. You give it a image, it gives you the object bounding boxes, classes and masks.
There are two stages of Mask RCNN. First, it generates proposals about the regions where there might be an object based on the input image. Second, it predicts the class of the object, refines the bounding box and generates a mask in pixel level of the object based on the first stage proposal. Both stages are connected to the backbone structure.
What is backbone? Backbone is a FPN style deep neural network. It consists of a bottom-up pathway , a top-bottom pathway and lateral connections. Bottom-up pathway can be any ConvNet, usually ResNet or VGG, which extracts features from raw images. Top-bottom pathway generates feature pyramid map which is similar in size to bottom-up pathway. Lateral connections are convolution and adding operations between two corresponding levels of the two pathways. FPN outperforms other single ConvNets mainly for the reason that it maintains strong semantically features at various resolution scales.
Now let’s look at the first stage. A light weight neural network called RPN scans all FPN top-bottom pathway( hereinafter referred to feature map) and proposes regions which may contain objects. That’s all it is. While scaning feature map is an efficient way, we need a method to bind features to its raw image location. Here come the anchors. Anchors are a set of boxes with predefined locations and scales relative to images. Ground-truth classes( only object or background binary classified at this stage) and bounding boxes are assigned to individual anchors according to some IoU value. As anchors with different scales bind to different levels of feature map, RPN uses these anchors to figure out where of the feature map ‘should’ get an object and what size of its bounding box is. Here we may agree that convolving, downsampling and upsampling would keep features staying the same relative locations as the objects in original image, and wouldn’t mess them around.
At the second stage, another neural network takes proposed regions by the first stage and assign them to several specific areas of a feature map level, scans these areas, and generates objects classes(multi-categorical classified), bounding boxes and masks. The procedure looks similar to RPN. Differences are that without the help of anchors, stage-two used a trick called ROIAlign to locate the relevant areas of feature map, and there is a branch generating masks for each objects in pixel level. Work completed.
#maskrcnn #objectdetection #deeplearning #ai #artificialintelligence #ml #computervision #cnn #convolutionalneuralnetwork
Комментарии