filmov
tv
Mixtral - Mixture of Experts (MoE) from Mistral
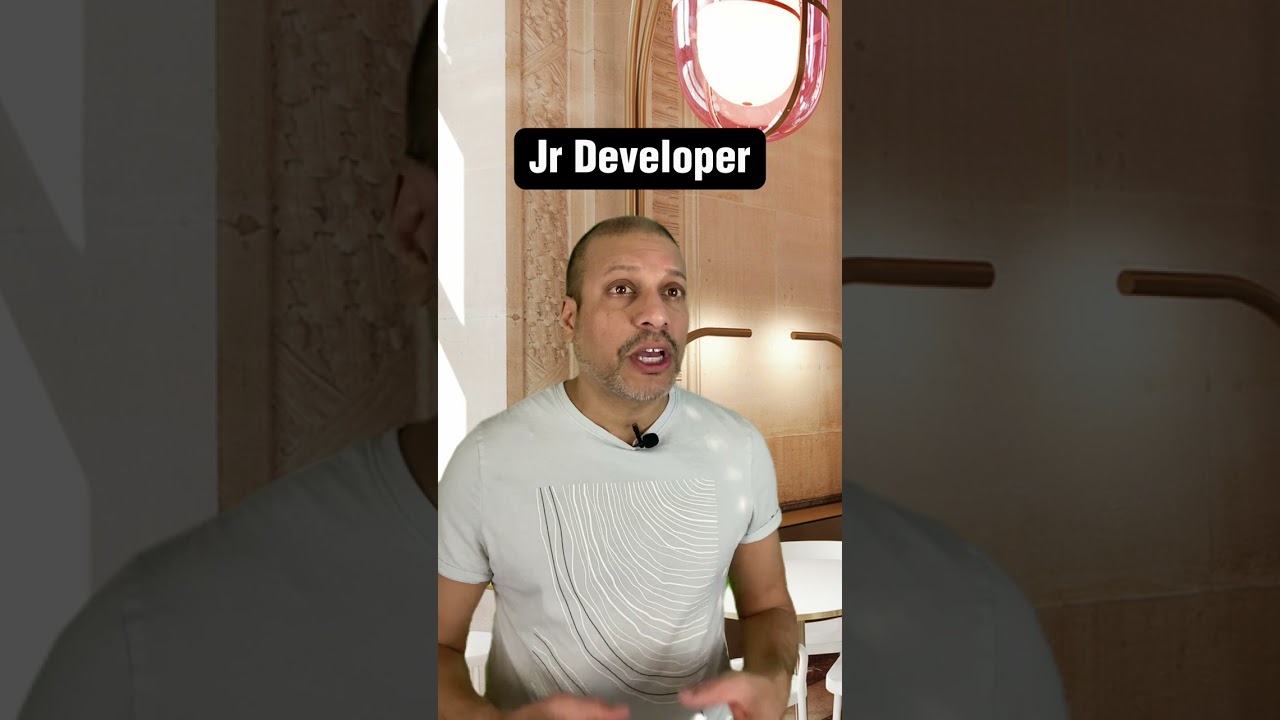
Показать описание
Mixtral is a new model using a mixture of experts (MoE) approach. It consists of 8x7B mistral models. It was pre-released on Friday, look for more details to come.
#largelanguagemodels #mixtral #mistral #rajistics
A version of the Mixtral model is here:
━━━━━━━━━━━━━━━━━━━━━━━━━
★ Rajistics Social Media »
━━━━━━━━━━━━━━━━━━━━━━━━━
#largelanguagemodels #mixtral #mistral #rajistics
A version of the Mixtral model is here:
━━━━━━━━━━━━━━━━━━━━━━━━━
★ Rajistics Social Media »
━━━━━━━━━━━━━━━━━━━━━━━━━
What are Mixture of Experts (GPT4, Mixtral…)?
Mistral 8x7B Part 1- So What is a Mixture of Experts Model?
Mixtral of Experts (Paper Explained)
Mixtral - Mixture of Experts (MoE) from Mistral
What is Mixture of Experts?
How Did Open Source Catch Up To OpenAI? [Mixtral-8x7B]
A Visual Guide to Mixture of Experts (MoE) in LLMs
Exploring Mixtral 8x7B: Mixture of Experts - The Key to Elevating LLMs
Stanford CS25: V4 I Demystifying Mixtral of Experts
Mistral / Mixtral Explained: Sliding Window Attention, Sparse Mixture of Experts, Rolling Buffer
Mistral AI’s New 8X7B Sparse Mixture-of-Experts (SMoE) Model in 5 Minutes
Mixtral 8x7B DESTROYS Other Models (MoE = AGI?)
Mixtral On Your Computer | Mixture-of-Experts LLM | Free GPT-4 Alternative | Tutorial
What is Mixture of Experts and 8*7B in Mixtral
Mixtral of Experts Insane NEW Research Paper! Mistral will beat GPT-4 Soon!
Mixtral of Experts
Fine-Tune Mixtral 8x7B (Mistral's Mixture of Experts MoE) Model - Walkthrough Guide
Deep dive into Mixture of Experts (MOE) with the Mixtral 8x7B paper
Qwen1.5 MoE: Powerful Mixture of Experts Model - On Par with Mixtral!
NEW Mixtral 8x22b Tested - Mistral's New Flagship MoE Open-Source Model
Mixture of Experts LLM - MoE explained in simple terms
[short] Mixtral of Experts
Mixtral - Mixture of Experts (MoE) Free LLM that Rivals ChatGPT (3.5) by Mistral | Overview & De...
Running Mixtral on your machine with Ollama
Комментарии