filmov
tv
Stanford CS25: V4 I Demystifying Mixtral of Experts
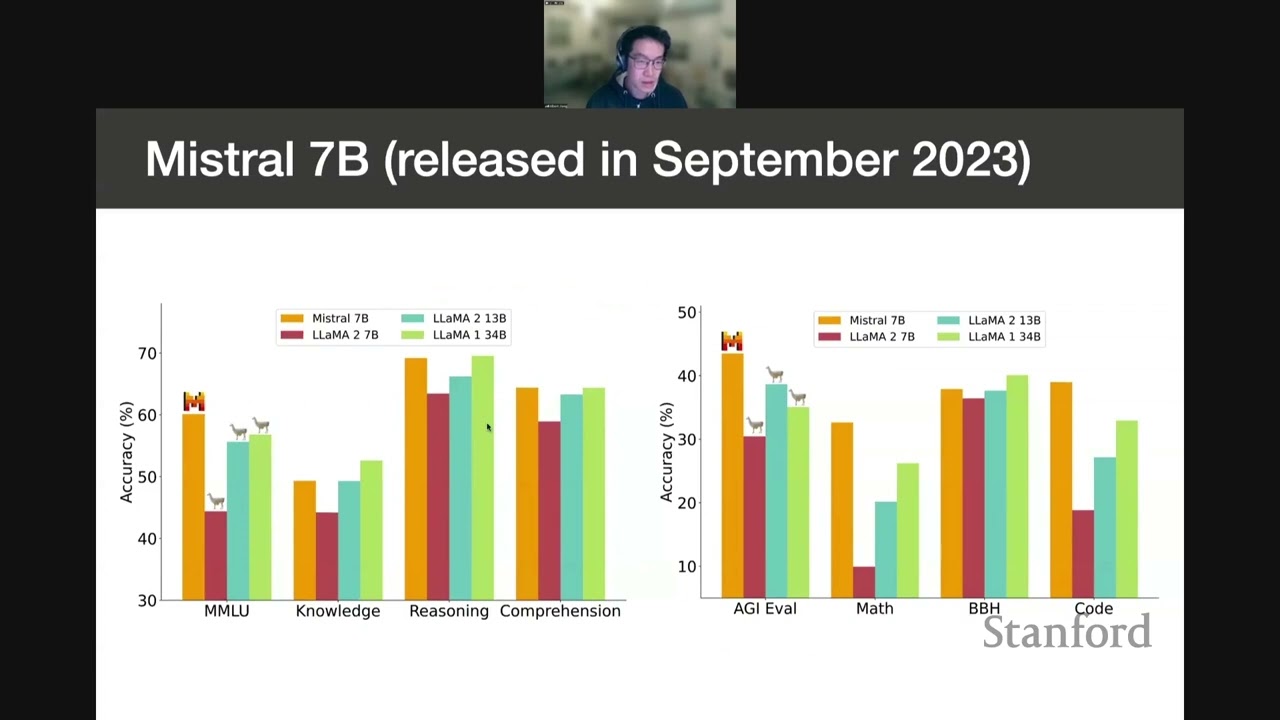
Показать описание
April 25, 2024
Speaker: Albert Jiang, Mistral AI / University of Cambridge
Demystifying Mixtral of Experts
In this talk I will introduce Mixtral 8x7B, a Sparse Mixture of Experts (SMoE) language model. Mixtral has the same architecture as Mistral 7B, with the difference that each layer is composed of 8 feedforward blocks (i.e. experts). For every token, at each layer, a router network selects two experts to process the current state and combines their outputs. Even though each token only sees two experts, the selected experts can be different at each timestep. As a result, each token has access to 47B parameters, but only uses 13B active parameters during inference. I will go into the architectural details and analyse the expert routing decisions made by the model.
About the speaker:
Albert Jiang is an AI scientist at Mistral AI, and a final-year PhD student at the computer science department of Cambridge University. He works on language model pretraining and reasoning at Mistral AI, and language models for mathematics at Cambridge.
Speaker: Albert Jiang, Mistral AI / University of Cambridge
Demystifying Mixtral of Experts
In this talk I will introduce Mixtral 8x7B, a Sparse Mixture of Experts (SMoE) language model. Mixtral has the same architecture as Mistral 7B, with the difference that each layer is composed of 8 feedforward blocks (i.e. experts). For every token, at each layer, a router network selects two experts to process the current state and combines their outputs. Even though each token only sees two experts, the selected experts can be different at each timestep. As a result, each token has access to 47B parameters, but only uses 13B active parameters during inference. I will go into the architectural details and analyse the expert routing decisions made by the model.
About the speaker:
Albert Jiang is an AI scientist at Mistral AI, and a final-year PhD student at the computer science department of Cambridge University. He works on language model pretraining and reasoning at Mistral AI, and language models for mathematics at Cambridge.
Stanford CS25: V4 I Demystifying Mixtral of Experts
Stanford CS25: V1 I Transformers United: DL Models that have revolutionized NLP, CV, RL
Stanford CS25: V1 I Mixture of Experts (MoE) paradigm and the Switch Transformer
Stanford CS25: V1 I Transformers in Vision: Tackling problems in Computer Vision
What is mechanistic interpretability? Neel Nanda explains.
Textbooks Are All You Need
George Hotz - GPT-4's real architecture is a 220B parameter mixture model with 8 sets of weight...
How Witty Works leverages Hugging Face to scale inclusive language
Transformer Series: Part-1 Basic Introduction (Dr. Aditya Nigam)
1L Attention - Theory [rough early thoughts]
Formalizing Explanations of Neural Network Behaviors
Create ChatGPT From Scratch in 40 Minute
Open Problems in Mechanistic Interpretability: A Whirlwind Tour
Transformer Encoder in 100 lines of code!
PyTorch Implementation of Transformers
[ 100k Special ] Transformers: Zero to Hero
Lukasz Kaiser – Transformers - How Far Can They Go?
Hands-On Workshop on Training and Using Transformers -- Kickoff Session
GPT-1 | Paper Explained & PyTorch Implementation
Lecture 20 - Efficient Transformers | MIT 6.S965
Building with Pre-Trained BART, BERT, and GPT-Style LLMs
【EP3】Large-Scale Visual Representation Learning with Vision Transformers
Vision Transformer
Deep Learning (Spring 2022) L5: Recurrent Neural Networks and Transformers
Комментарии