filmov
tv
Mixture of Experts LLM - MoE explained in simple terms
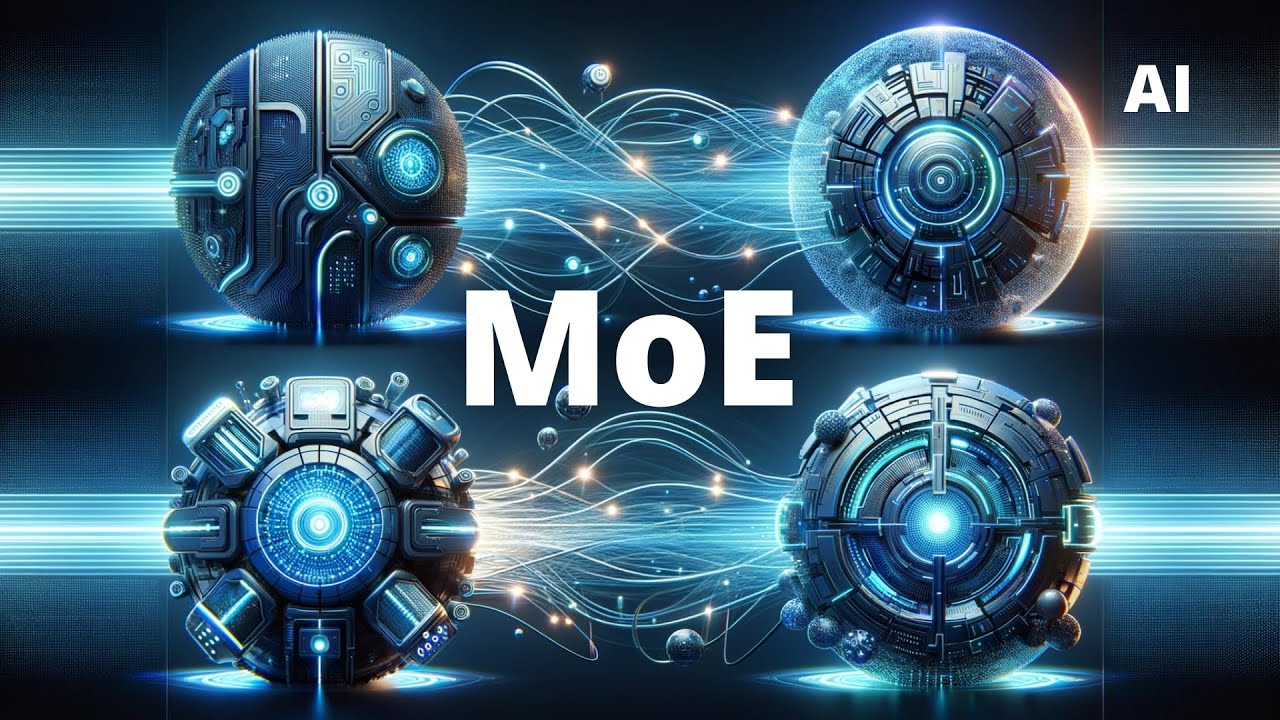
Показать описание
Mixture of Experts - MoE explained in simple terms with three easy examples.
You can test Mixtral 8x7B through this link (sign-in required, beta version only, beware):
GPT-4 generated text:
The video transcript provides a comprehensive overview of the development and optimization of mixture of experts (MoE) systems in the context of Large Language Models (LLMs). The presenter begins by introducing the concept of MoE as a framework for decomposing LLMs into smaller, specialized systems that focus on distinct aspects of input data. This approach, particularly when sparsely activated, enhances computational efficiency and resource allocation, especially in parallel GPU computing environments. The video traces the evolution of MoE systems from their inception in 2017 by Google Brain, highlighting the integration of MoE layers within recurrent language models and the critical role of the gating network in directing input tokens to the appropriate expert systems.
The technical specifics of MoE systems are delved into, focusing on the gating network's intelligence in assigning tokens to specific expert systems. Various gating functions, such as softmax gating and noisy top-k gating, are discussed, detailing their role in the sparsity and noise addition to the gating process. The presenter emphasizes the importance of backpropagation in training the gating network alongside the rest of the model, ensuring effective assignment of tokens and balancing computational load. The video also addresses the challenges of data parallelism and model parallelism in MoE systems, underlining the need for balanced network bandwidth and utilization.
Advancements in MoE systems are discussed, with a particular focus on the development of 'megablocks' in 2022, which tackled limitations of classical MoE systems by reformulating computations in terms of block sparse mathematical operations. This innovation led to the creation of more efficient GPU kernels for block sparse matrix multiplication, significantly enhancing the computational speed. The video concludes by discussing the latest trends in MoE systems, including the integration of instruction tuning in 2023, which further refined the performance of MoE systems on downstream tasks. The presentation provides an in-depth view of the evolution, technical underpinnings, and future directions of MoE systems in the realm of LLMs and vision language models.
Mixtral 8x7B config:
"dim": 4096,
"n_layers": 32,
"head_dim": 128,
"hidden_dim": 14336,
"n_heads": 32,
"n_kv_heads": 8,
"norm_eps": 1e-05,
"vocab_size": 32000,
"moe": {
"num_experts_per_tok": 2,
"num_experts": 8
Unproven info: GPT-4’s 8 experts with 111 billion parameters each.
recommended literature:
---------------------------------
MEGABLOCKS: EFFICIENT SPARSE TRAINING WITH MIXTURE-OF-EXPERTS
OUTRAGEOUSLY LARGE NEURAL NETWORKS:
THE SPARSELY-GATED MIXTURE-OF-EXPERTS LAYER
Github: MegaBlocks is a light-weight library for mixture-of-experts (MoE) training
#ai
#experts
#tutorialyoutube
You can test Mixtral 8x7B through this link (sign-in required, beta version only, beware):
GPT-4 generated text:
The video transcript provides a comprehensive overview of the development and optimization of mixture of experts (MoE) systems in the context of Large Language Models (LLMs). The presenter begins by introducing the concept of MoE as a framework for decomposing LLMs into smaller, specialized systems that focus on distinct aspects of input data. This approach, particularly when sparsely activated, enhances computational efficiency and resource allocation, especially in parallel GPU computing environments. The video traces the evolution of MoE systems from their inception in 2017 by Google Brain, highlighting the integration of MoE layers within recurrent language models and the critical role of the gating network in directing input tokens to the appropriate expert systems.
The technical specifics of MoE systems are delved into, focusing on the gating network's intelligence in assigning tokens to specific expert systems. Various gating functions, such as softmax gating and noisy top-k gating, are discussed, detailing their role in the sparsity and noise addition to the gating process. The presenter emphasizes the importance of backpropagation in training the gating network alongside the rest of the model, ensuring effective assignment of tokens and balancing computational load. The video also addresses the challenges of data parallelism and model parallelism in MoE systems, underlining the need for balanced network bandwidth and utilization.
Advancements in MoE systems are discussed, with a particular focus on the development of 'megablocks' in 2022, which tackled limitations of classical MoE systems by reformulating computations in terms of block sparse mathematical operations. This innovation led to the creation of more efficient GPU kernels for block sparse matrix multiplication, significantly enhancing the computational speed. The video concludes by discussing the latest trends in MoE systems, including the integration of instruction tuning in 2023, which further refined the performance of MoE systems on downstream tasks. The presentation provides an in-depth view of the evolution, technical underpinnings, and future directions of MoE systems in the realm of LLMs and vision language models.
Mixtral 8x7B config:
"dim": 4096,
"n_layers": 32,
"head_dim": 128,
"hidden_dim": 14336,
"n_heads": 32,
"n_kv_heads": 8,
"norm_eps": 1e-05,
"vocab_size": 32000,
"moe": {
"num_experts_per_tok": 2,
"num_experts": 8
Unproven info: GPT-4’s 8 experts with 111 billion parameters each.
recommended literature:
---------------------------------
MEGABLOCKS: EFFICIENT SPARSE TRAINING WITH MIXTURE-OF-EXPERTS
OUTRAGEOUSLY LARGE NEURAL NETWORKS:
THE SPARSELY-GATED MIXTURE-OF-EXPERTS LAYER
Github: MegaBlocks is a light-weight library for mixture-of-experts (MoE) training
#ai
#experts
#tutorialyoutube
Комментарии