filmov
tv
Hypothesis Testing - Introductory Statistics; null hypothesis; alternative hypothesis
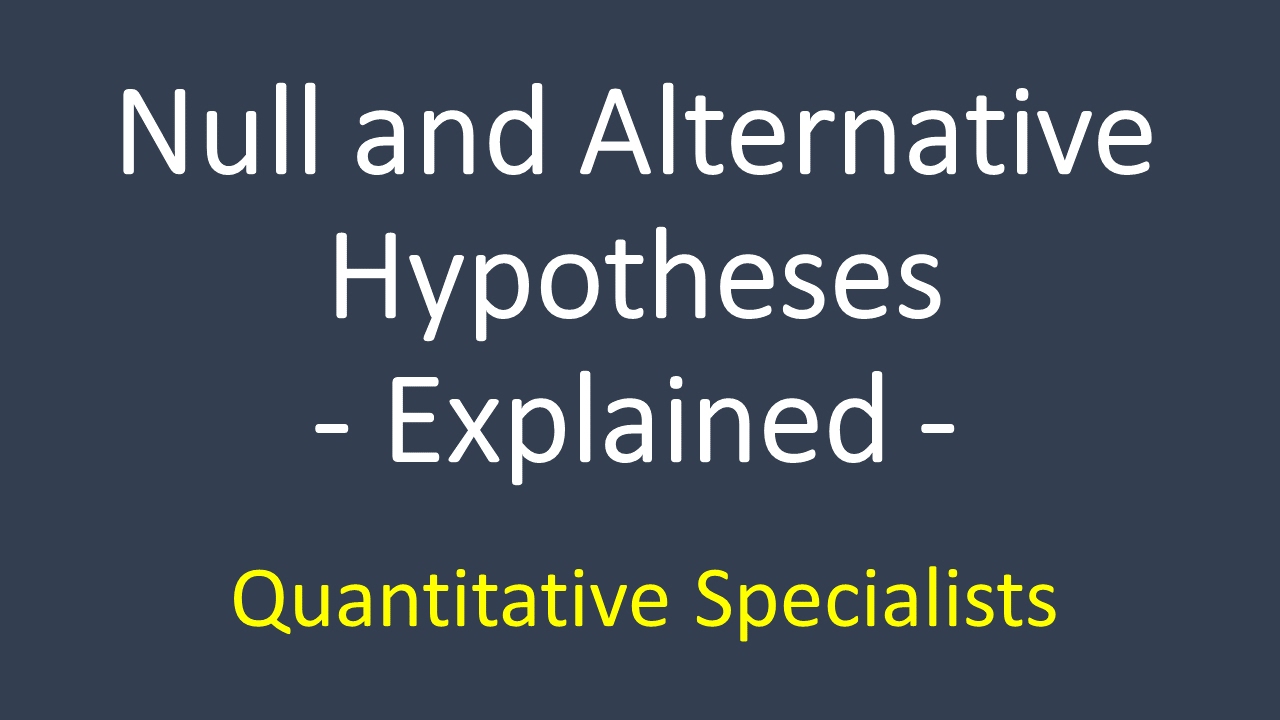
Показать описание
In this video we examine hypothesis tests, including the null and alternative hypotheses. We take a look at a few different examples, with a focus on two-tailed tests in this video.
Null hypothesis
Alternative hypothesis
H0
H1
Video Transcript: In this video we'll take a look at null and alternative hypotheses. Now the null hypothesis means there's no effect, or nothing happened, or there's no difference. The null hypothesis is often represented by H Sub zero. And it makes a statement about the population, not the sample. So in other words we put population values or symbols in our null hypothesis. Now the alternative hypothesis is really the opposite. It states or it means that there was an effect, or something happened, or there was a difference. The alternative hypothesis is often represented by H sub 1 or H sub A, and it also makes a statement about the population, not the sample. So if we take a look at these two side-by-side, once again, in review, the null is stated by H sub 0 the alternative is H sub 1 or H sub A. The null basically states nothing happened, and look at the opposite here, the alternative states something happened. Or the null can state no effect, the alternative states there was an effect. And, finally, the null can state no difference effectively, and the alternative would state the opposite, there was a difference. And once again both hypotheses refer to the population. Let's go ahead and take a look at an example using the Pearson correlation or Pearson's r. Now correlation measures the degree of the linear relationship, if there's any at all, between two variables, and it's known as Pearson's r. Let's go and take a look at the null and alternative hypotheses for correlation, or fir Pearson's r here. In words the null would state there is not a relationship between the two variables in the population. The alternative would state the opposite: it would state there is a relationship between the two variables in the population. Notice how the null states no effect, or there's no relationship, whereas the alternative states there is an effect, or there is a relationship. Using symbols we could say the following: the null, and that little thing that looks like a p there, that stands for rho, and it's the correlation in the population. So we would say null rho x,y equals 0 and then the alternative would say rho x,y does not equal zero. Or, in other words, the null would state there's no correlation between x and y, two variables in the population, whereas the alternative would state there is a correlation between the two variables, x and y, in the population. And 0 here means no relationship in correlation. So when the null says it's equal to 0, it's saying there's no relationship. When the alternative says it's not equal to 0, it stating there is a relationship. So, in review, the null states there's no effect or zero relationship, whereas the alternative states there is an effect, or a non-zero relationship. Now hypotheses need to be mutually exclusive and exhaustive. Exclusive means there's no overlap between the null and the alternative. And if you look at our two statements up above, where it says rho x,y equals 0, and rho x,y does not equal zero, notice that those do not overlap at all, equals and not equals are completely non overlapping. It's either 0, which is the null in that case, or it's not zero, which is the alternative. So they're completely exclusive, they do not overlap. And then exhaustive means they must cover, or exhaust, all possibilities, the null and alternative when taken together. And notice that they do, as every possible value for Pearson's r is either 0 or not 0, so it does exhaust all possibilities. So once again it’s exclusive, because they don't overlap, and it's exhaustive, because they cover all possibilities. Now notice how the alternative has a not equal sign, implying that the alternative hypothesis can be either greater than zero, or less than zero, or in other words correlation can be positive or negative. This is known as a two-tailed test, since the alternative hypothesis consists of two possibilities, either greater than zero or less than zero. Alternatively, one-tailed tests can also be used in hypothesis testing, and we'll examine one-tailed tests in another video.
Null hypothesis
Alternative hypothesis
H0
H1
Video Transcript: In this video we'll take a look at null and alternative hypotheses. Now the null hypothesis means there's no effect, or nothing happened, or there's no difference. The null hypothesis is often represented by H Sub zero. And it makes a statement about the population, not the sample. So in other words we put population values or symbols in our null hypothesis. Now the alternative hypothesis is really the opposite. It states or it means that there was an effect, or something happened, or there was a difference. The alternative hypothesis is often represented by H sub 1 or H sub A, and it also makes a statement about the population, not the sample. So if we take a look at these two side-by-side, once again, in review, the null is stated by H sub 0 the alternative is H sub 1 or H sub A. The null basically states nothing happened, and look at the opposite here, the alternative states something happened. Or the null can state no effect, the alternative states there was an effect. And, finally, the null can state no difference effectively, and the alternative would state the opposite, there was a difference. And once again both hypotheses refer to the population. Let's go ahead and take a look at an example using the Pearson correlation or Pearson's r. Now correlation measures the degree of the linear relationship, if there's any at all, between two variables, and it's known as Pearson's r. Let's go and take a look at the null and alternative hypotheses for correlation, or fir Pearson's r here. In words the null would state there is not a relationship between the two variables in the population. The alternative would state the opposite: it would state there is a relationship between the two variables in the population. Notice how the null states no effect, or there's no relationship, whereas the alternative states there is an effect, or there is a relationship. Using symbols we could say the following: the null, and that little thing that looks like a p there, that stands for rho, and it's the correlation in the population. So we would say null rho x,y equals 0 and then the alternative would say rho x,y does not equal zero. Or, in other words, the null would state there's no correlation between x and y, two variables in the population, whereas the alternative would state there is a correlation between the two variables, x and y, in the population. And 0 here means no relationship in correlation. So when the null says it's equal to 0, it's saying there's no relationship. When the alternative says it's not equal to 0, it stating there is a relationship. So, in review, the null states there's no effect or zero relationship, whereas the alternative states there is an effect, or a non-zero relationship. Now hypotheses need to be mutually exclusive and exhaustive. Exclusive means there's no overlap between the null and the alternative. And if you look at our two statements up above, where it says rho x,y equals 0, and rho x,y does not equal zero, notice that those do not overlap at all, equals and not equals are completely non overlapping. It's either 0, which is the null in that case, or it's not zero, which is the alternative. So they're completely exclusive, they do not overlap. And then exhaustive means they must cover, or exhaust, all possibilities, the null and alternative when taken together. And notice that they do, as every possible value for Pearson's r is either 0 or not 0, so it does exhaust all possibilities. So once again it’s exclusive, because they don't overlap, and it's exhaustive, because they cover all possibilities. Now notice how the alternative has a not equal sign, implying that the alternative hypothesis can be either greater than zero, or less than zero, or in other words correlation can be positive or negative. This is known as a two-tailed test, since the alternative hypothesis consists of two possibilities, either greater than zero or less than zero. Alternatively, one-tailed tests can also be used in hypothesis testing, and we'll examine one-tailed tests in another video.
Комментарии