filmov
tv
All Formulas of Conditional Probability #shorts #formula #probability
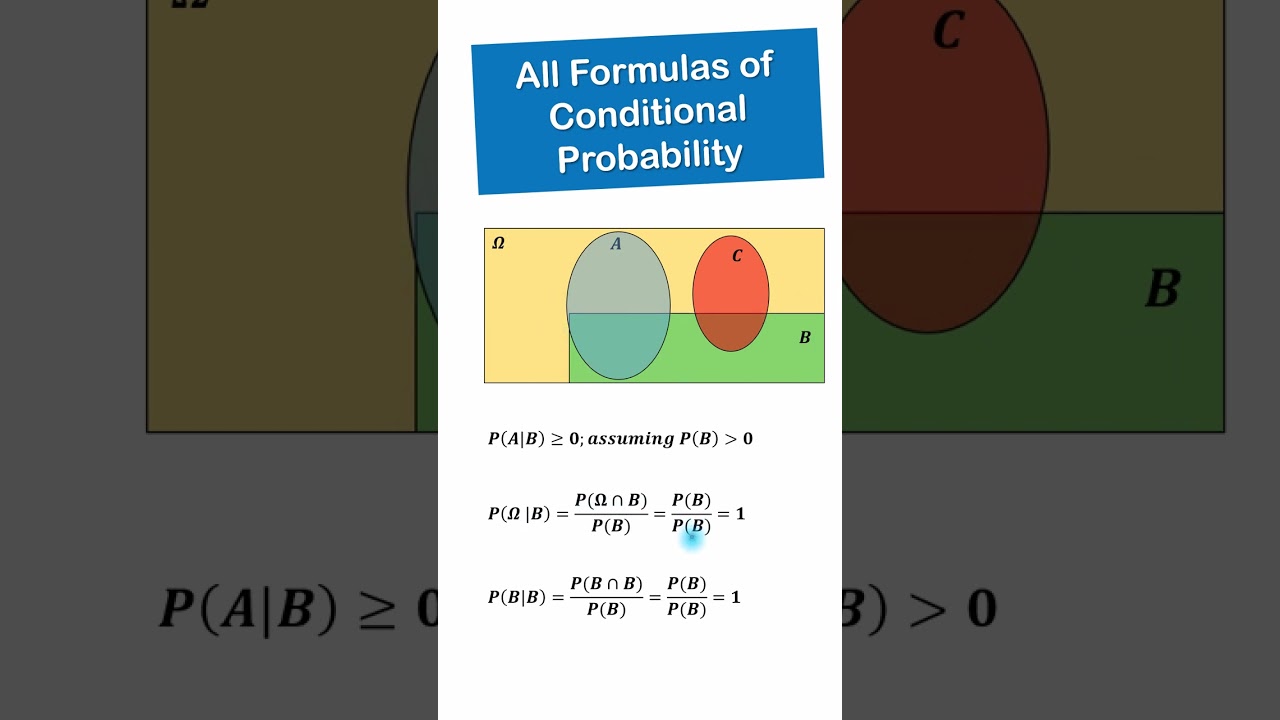
Показать описание
All formulas of conditional probability. Conditional probabilities are just the same as ordinary probabilities applied to a different situation. They do not taste or smell or behave any differently than ordinary probabilities. What do I mean by that? I mean that they satisfy the usual probability axioms. For example, ordinary probabilities must also be non-negative. Is this true for conditional probabilities? Of course it is true, because conditional probabilities are defined as a ratio of two probabilities. Probabilities are non-negative. So the ratio will also be non-negative, of course as long as it is well-defined. And here we need to remember that we only talk about conditional probabilities when we condition on an event that itself has positive probability. How about another axiom? What is the probability of the entire sample space, given the event B? Let's check it out. By definition, the conditional probability is the probability of the intersection of the two events involved divided by the probability of the conditioning event. Now, what is the intersection of omega with B? B is a subset of omega. So when we intersect the two sets, we're left just with B itself. So the numerator becomes the probability of B. We're dividing by the probability of B, and so the answer is equal to 1. So indeed, the sample space has unit probability, even under the conditional model. Now, remember that when we condition on an event B, we could still work with the original sample space. However, possible outcomes that do not belong to B are considered impossible, so we might as well think of B itself as being our sample space. If we proceed like that and think now of B as being our new sample space, what is the probability of this new sample space in the conditional model? Let's apply the definition once more. It's the probability of the intersection of the two events involved, B intersection B, divided by the probability of the conditioning event. What is the numerator? The intersection of B with itself is just B, so the numerator is the probability of B. We're dividing by the probability of B. So the answer is, again, 1. Finally, we need to check the additivity axiom. Recall what the additivity axiom says. If we have two events, two subsets of the sample space that are disjoint, then the probability of their union is equal to the sum of their individual probabilities. Is this going to be the case if we now condition on a certain event? What we want to prove is the following statement. If we take two events that are disjoint, they have empty intersection, then the probability of the union is the sum of their individual probabilities, but where now the probabilities that we're employing are the conditional probabilities, given the event B. So let us verify whether this relation, this fact is correct or not. Let us take this quantity and use the definition to write it out. By definition, this conditional probability is the probability of the intersection of the first event of interest, the one that appears on this side of the conditioning, intersection with the event on which we are conditioning. And then we divide by the probability of the conditioning event, B. Now, let's look at this quantity, what is it? Here's an interesting observation. The events A and C are disjoint. The piece of A that also belongs in B, therefore, is disjoint from the piece of C that also belongs to B. Therefore, this set here and that set here are disjoint. Since they are disjoint, the probability of their union has to be equal to the sum of their individual probabilities. So here we're using the additivity axiom on the original probabilities to break this probability up into two pieces. And now we observe that here we have the ratio of an intersection by the probability of B. This is just the conditional probability of A given B using the definition of conditional probabilities. And the second part is the conditional probability of C given B, where, again, we're using the definition of conditional probabilities. So we have indeed checked that this additivity property is true for the case of conditional probabilities when we consider two disjoint events. Now, we could repeat the same derivation and verify that it is also true for the case of a disjoint union, of finitely many events, or even for countably many disjoint events. So we do have finite and countable additivity. We're not proving it, but the argument is exactly the same as for the case of two events. So conditional probabilities do satisfy all of the standard axioms of probability theory. So conditional probabilities are just like ordinary probabilities. This actually has a very important implication. Since conditional probabilities satisfy all of the probability axioms, any formula or theorem that we ever derive for ordinary probabilities will remain true for conditional probabilities as well