filmov
tv
Sampling Distributions of the Least-Squares Estimators When the Error Terms are Normally Distributed
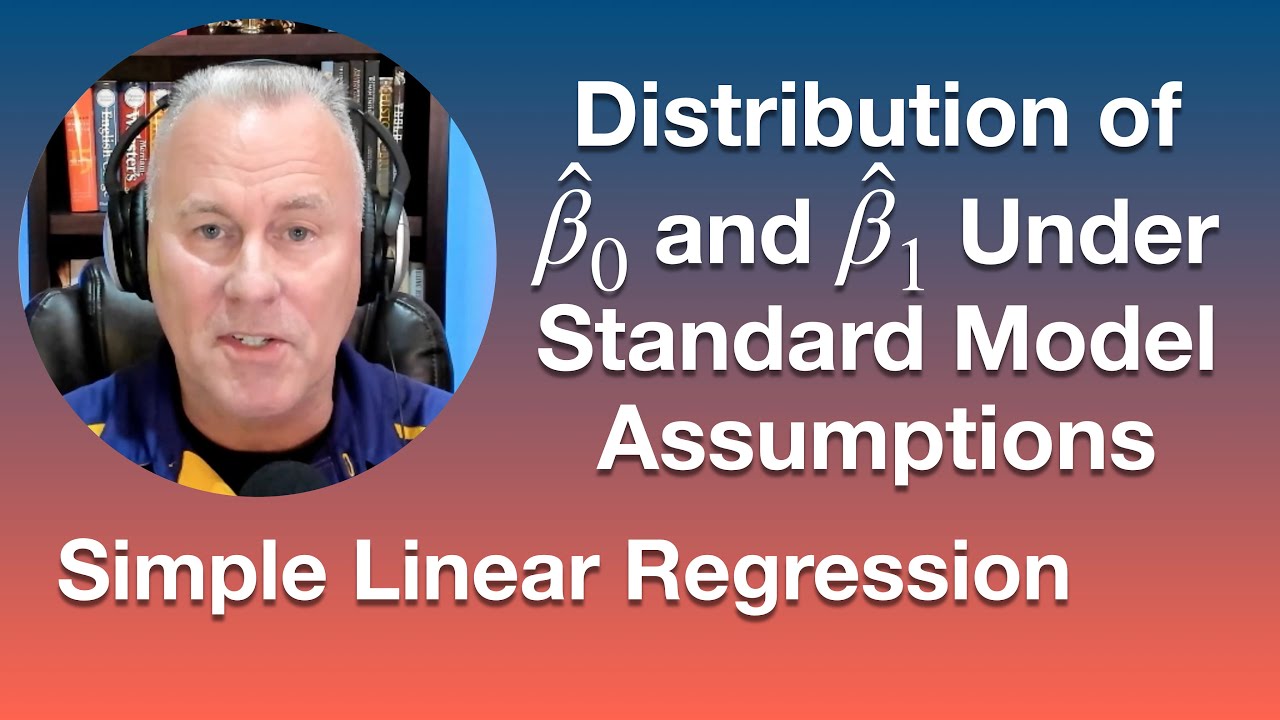
Показать описание
In previous lecture videos we derived characteristics of the sampling distributions of the least-squares estimators of the beta parameters in the simple linear regression model. Using the means and the standard deviations of these sampling distributions, we looked at two ways of approximating probabilities of events involving the least-squares estimators.
The first approach we looked at was based on Chebyshev’s Inequality. This approach gives bounds on probabilities that are GUARANTEED to hold, and it is applicable in ANY situation in which the standard deviation of the sampling distribution of the estimator is finite. But because this approach IS so broadly applicable, and because the bounds it gives are guaranteed, the bounds are very, very conservative in most cases, and when more information is available about the sampling distributions (for example, their shapes), better approximations can often be obtained.
We then looked at approximating probabilities using The Empirical Rule. This approach gives more accurate approximations than Chebyshev’s Inequality when the sampling distribution of the least-squares estimator is bell-shaped.
This raises the question: under what conditions will the sampling distributions of the least-squares estimators be bell-shaped?
In this lecture video we begin looking at this question, and provide sufficient conditions for the sampling distributions of the least-squares estimators to be bell-shaped, and more specifically, Normally distributed. This will be the case when the model error terms are themselves Normally distributed.
Here is an outline of the topics covered in this video:
Sampling Distribution of beta_1_hat
Identify the Sampling Distribution of beta_1_hat when the Model Error Terms are Normally Distributed
Provide the Functional Form of the pdf of the Marginal Distribution of beta_1_hat
Identify the Sampling Distribution of the Standardized Version of beta_1_hat
Probabilities Involving beta_1_hat
Sampling Distribution of beta_0_hat
Identify the Sampling Distribution of beta_0_hat when the Model Error Terms are Normally Distributed
Provide the Functional Form of the pdf of the Marginal Distribution of beta_0_hat
Identify the Sampling Distribution of the Standardized Version of beta_0_hat
Probabilities Involving beta_0_hat
Identify the Joint Sampling Distribution of beta_0_hat and beta_1_hat
Mean Vector of the Joint Distribution of beta_0_hat and beta_1_hat
Specify the Form of the Covariance Matrix of the Joint Distribution of beta_0_hat and beta_1_hat
Specify the Form of the Correlation Matrix of the Joint Distribution of beta_0_hat and beta_1_hat
Provide the Functional Form of the pdf of the Joint Distribution of beta_0_hat and beta_1_hat
Here is the textbook we are using:
Title: "Introduction to Linear Regression Analysis 5th Edition"
Authors: Douglas C. Montgomery, Elizabeth A. Peck, G. Geoffrey Vining.
ISBN-13: 978-0470542811
ISBN-10: 0470542810
Software Packages Used:
Music Title: Urban Morning
Music Artist: Prigida
You can navigate to a particular topic in the lecture by clicking on a timecode below:
Timecodes
0:00 Introduction
1:45 Outline
3:33 Sampling Distribution of Beta_1_hat
5:21 Functional Form of the pdf of the Marginal Distribution of Beta_1_hat
6:15 Sampling Distribution of the Standardized Version of Beta_1_hat
7:21 Probabilities Involving Beta_1_hat
12:14 Standardization + Standard Normal Table
12:35 Using a Calculator with the Normal CDF Built-In
14:04 Sampling Distribution of Beta_0_hat
15:19 Functional Form of the pdf of the Marginal Distribution of Beta_0_hat
16:11 Sampling Distribution of the Standardized Version of Beta_0_hat
17:18 Probabilities Involving Beta_0_hat
19:46 Joint Sampling Distribution of Beta_0_hat and Beta_1_hat
19:54 Mean Vector of the Joint Distribution of Beta_0_hat and Beta_1_hat
20:55 Covariance Matrix of the Joint Distribution of Beta_0_hat and Beta_1_hat
22:55 Correlation Matrix of the Joint Distribution of Beta_0_hat and Beta_1_hat
24:43 Functional Form of the Joint Distribution of Beta_0_hat and Beta_1_hat
26:31 Wrapping Up + Outro Music
28:25 Related Lecture Videos
The first approach we looked at was based on Chebyshev’s Inequality. This approach gives bounds on probabilities that are GUARANTEED to hold, and it is applicable in ANY situation in which the standard deviation of the sampling distribution of the estimator is finite. But because this approach IS so broadly applicable, and because the bounds it gives are guaranteed, the bounds are very, very conservative in most cases, and when more information is available about the sampling distributions (for example, their shapes), better approximations can often be obtained.
We then looked at approximating probabilities using The Empirical Rule. This approach gives more accurate approximations than Chebyshev’s Inequality when the sampling distribution of the least-squares estimator is bell-shaped.
This raises the question: under what conditions will the sampling distributions of the least-squares estimators be bell-shaped?
In this lecture video we begin looking at this question, and provide sufficient conditions for the sampling distributions of the least-squares estimators to be bell-shaped, and more specifically, Normally distributed. This will be the case when the model error terms are themselves Normally distributed.
Here is an outline of the topics covered in this video:
Sampling Distribution of beta_1_hat
Identify the Sampling Distribution of beta_1_hat when the Model Error Terms are Normally Distributed
Provide the Functional Form of the pdf of the Marginal Distribution of beta_1_hat
Identify the Sampling Distribution of the Standardized Version of beta_1_hat
Probabilities Involving beta_1_hat
Sampling Distribution of beta_0_hat
Identify the Sampling Distribution of beta_0_hat when the Model Error Terms are Normally Distributed
Provide the Functional Form of the pdf of the Marginal Distribution of beta_0_hat
Identify the Sampling Distribution of the Standardized Version of beta_0_hat
Probabilities Involving beta_0_hat
Identify the Joint Sampling Distribution of beta_0_hat and beta_1_hat
Mean Vector of the Joint Distribution of beta_0_hat and beta_1_hat
Specify the Form of the Covariance Matrix of the Joint Distribution of beta_0_hat and beta_1_hat
Specify the Form of the Correlation Matrix of the Joint Distribution of beta_0_hat and beta_1_hat
Provide the Functional Form of the pdf of the Joint Distribution of beta_0_hat and beta_1_hat
Here is the textbook we are using:
Title: "Introduction to Linear Regression Analysis 5th Edition"
Authors: Douglas C. Montgomery, Elizabeth A. Peck, G. Geoffrey Vining.
ISBN-13: 978-0470542811
ISBN-10: 0470542810
Software Packages Used:
Music Title: Urban Morning
Music Artist: Prigida
You can navigate to a particular topic in the lecture by clicking on a timecode below:
Timecodes
0:00 Introduction
1:45 Outline
3:33 Sampling Distribution of Beta_1_hat
5:21 Functional Form of the pdf of the Marginal Distribution of Beta_1_hat
6:15 Sampling Distribution of the Standardized Version of Beta_1_hat
7:21 Probabilities Involving Beta_1_hat
12:14 Standardization + Standard Normal Table
12:35 Using a Calculator with the Normal CDF Built-In
14:04 Sampling Distribution of Beta_0_hat
15:19 Functional Form of the pdf of the Marginal Distribution of Beta_0_hat
16:11 Sampling Distribution of the Standardized Version of Beta_0_hat
17:18 Probabilities Involving Beta_0_hat
19:46 Joint Sampling Distribution of Beta_0_hat and Beta_1_hat
19:54 Mean Vector of the Joint Distribution of Beta_0_hat and Beta_1_hat
20:55 Covariance Matrix of the Joint Distribution of Beta_0_hat and Beta_1_hat
22:55 Correlation Matrix of the Joint Distribution of Beta_0_hat and Beta_1_hat
24:43 Functional Form of the Joint Distribution of Beta_0_hat and Beta_1_hat
26:31 Wrapping Up + Outro Music
28:25 Related Lecture Videos
Комментарии