filmov
tv
Conditional Probability (1 of 7: A surprising example)
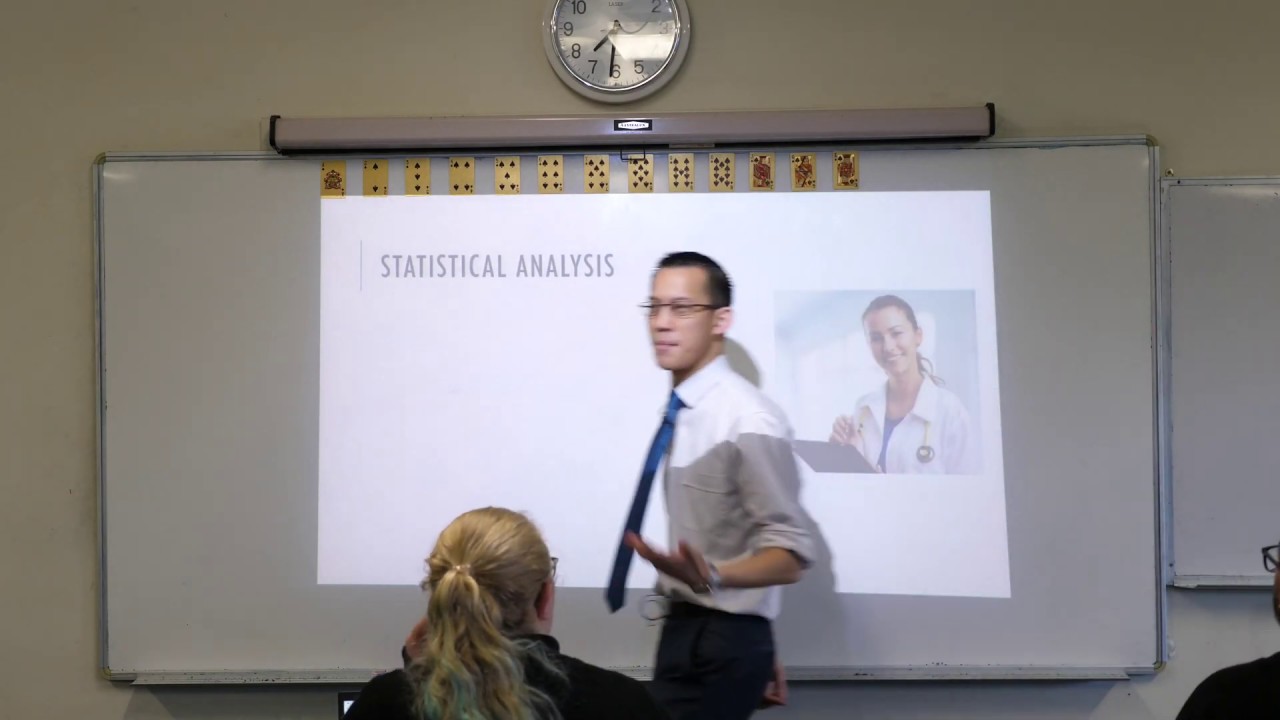
Показать описание
Conditional Probability (1 of 7: A surprising example)
Intro to Conditional Probability
Edexcel S1 Tutorial 15 Conditional Probability
Data Science 1 - Probability 7 - Conditional Probability
Ch.2 Conditional Probability (1-7)
Conditional probability | Q9 P1 | 10A | EoT2 |
7 Conditional Probability - Part 1 | Definition and Example
Conditional Probabilities, Clearly Explained!!!
Conditional Probability | GCSE Maths 2025
Probability Formulas, Symbols & Notations - Marginal, Joint, & Conditional Probabilities
Prob 7 1 Conditional Distribution
Conditional Probability - Example 1
Math Antics - Basic Probability
Conditional Probability with Easiest Explanation & Example
AQA Statistics 1 2.05 Introducing Conditional Probability
Conditional Probability With Venn Diagrams & Contingency Tables
Calculating conditional probability | Probability and Statistics | Khan Academy
Tutorial 7 || Conditional probability || Conditional probability in a nutshell || Probability theory
Venn Diagrams (A intersection B, A' union B')
Finite Math 4 7 Part 1 Conditional Probability
1,23 Geo 7 Conditional probability and testing for independence
Probability Of Rolling Dice | How To Calculate Dice Probabilities #Shorts #NowWeKnow
Conditional Probability
[Discrete Mathematics] Conditional Probability
Комментарии