filmov
tv
Linear Discriminant Analysis (LDA) | Machine Learning Tutorial | myTectra
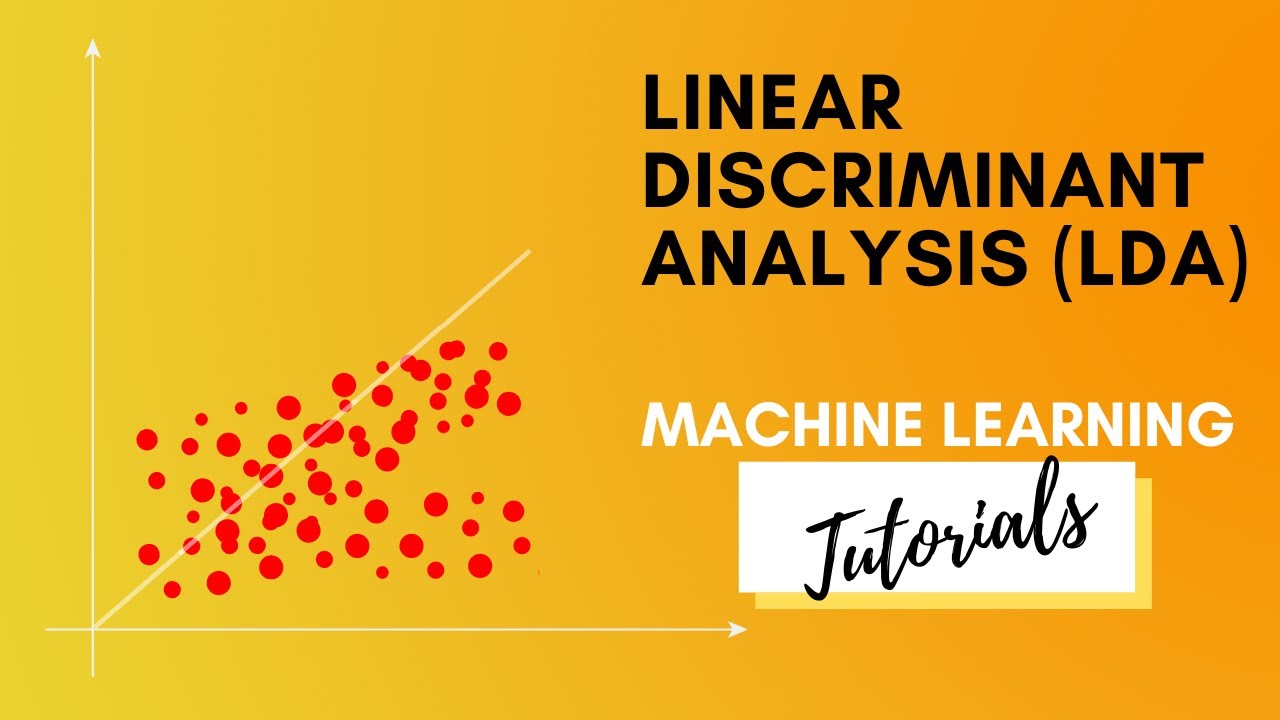
Показать описание
In this video, we are going to discuss Linear Discriminant Analysis (LDA)
and Principal factor analysis(PCA).
There are many available techniques for the classification of data. Principal factor analysis (PCA) and Linear Discriminant Analysis (LDA) are two commonly used techniques for data classification and dimensionality reduction. PCA finds the most accurate data representation in a lower-dimensional space. It projects data in the directions of maximum variance. However, the directions of maximum variance may not be useful for classification.
Starting from PCA, and the method used to solve it, Singular Value disintegration, we are taking you slow and steady so that the simplicity of the method is revealed and clearly explained. Fundamental Component Analysis is one of the most useful data analysis and machine learning methods out there. It can be used to identify patterns in highly complex datasets and it can tell you what variables in your data are the most important.
Linear Discriminant Analysis (LDA) is most commonly used as a dimensionality reduction technique in the pre-processing step for pattern classification and machine learning applications. The goal is to project a dataset onto a lower-dimensional space with good class-separability in order to avoid over-fitting (“curse of dimensionality”) and also reduce computational costs.
=================================================
Connect With Us!
Call us on +91 8047112411
WhatsApp on +91 9513261570
#lineardiscriminantanalysis #lda #ldaalgorithm #ldamachinelearning #ldainartificialintelligence #ml#mytectra #mltraining #machinelearning #mytectratutorial #machinelearningtraining #lineardiscriminantanalysisinmachinelearning
and Principal factor analysis(PCA).
There are many available techniques for the classification of data. Principal factor analysis (PCA) and Linear Discriminant Analysis (LDA) are two commonly used techniques for data classification and dimensionality reduction. PCA finds the most accurate data representation in a lower-dimensional space. It projects data in the directions of maximum variance. However, the directions of maximum variance may not be useful for classification.
Starting from PCA, and the method used to solve it, Singular Value disintegration, we are taking you slow and steady so that the simplicity of the method is revealed and clearly explained. Fundamental Component Analysis is one of the most useful data analysis and machine learning methods out there. It can be used to identify patterns in highly complex datasets and it can tell you what variables in your data are the most important.
Linear Discriminant Analysis (LDA) is most commonly used as a dimensionality reduction technique in the pre-processing step for pattern classification and machine learning applications. The goal is to project a dataset onto a lower-dimensional space with good class-separability in order to avoid over-fitting (“curse of dimensionality”) and also reduce computational costs.
=================================================
Connect With Us!
Call us on +91 8047112411
WhatsApp on +91 9513261570
#lineardiscriminantanalysis #lda #ldaalgorithm #ldamachinelearning #ldainartificialintelligence #ml#mytectra #mltraining #machinelearning #mytectratutorial #machinelearningtraining #lineardiscriminantanalysisinmachinelearning