filmov
tv
How to Do Speech Recognition with Arduino | Digi-Key Electronics
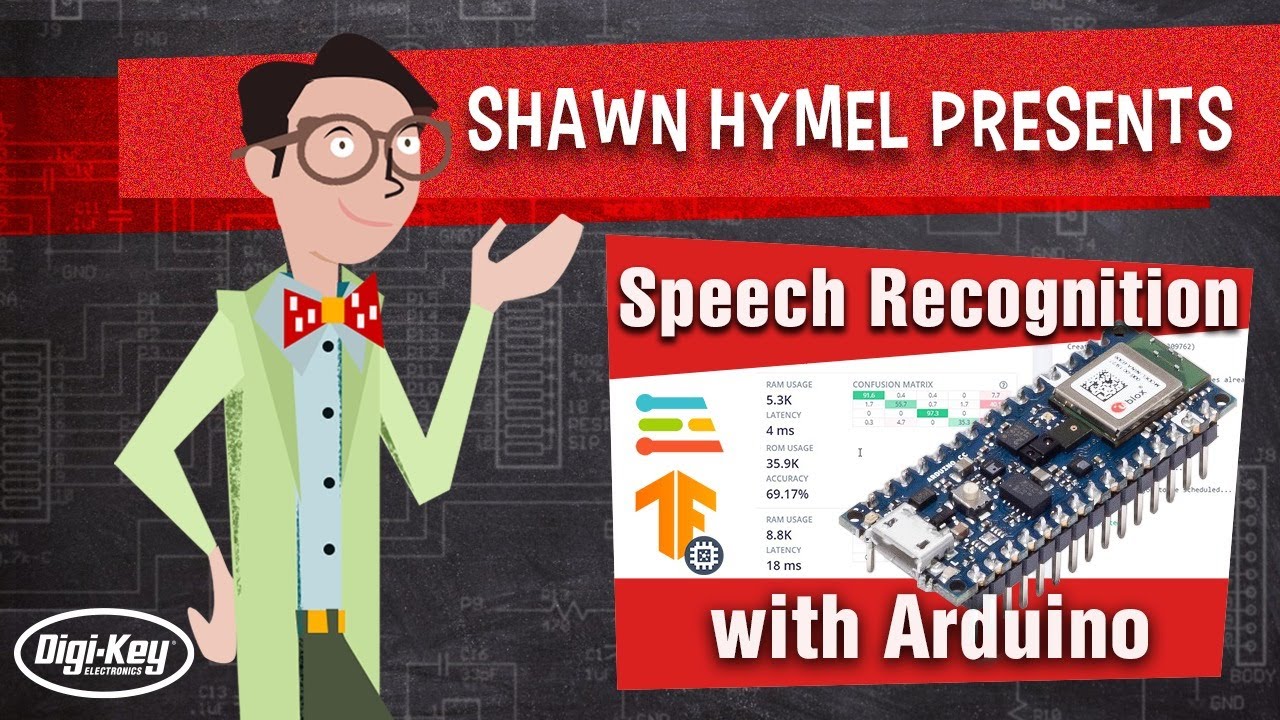
Показать описание
Speech recognition is the process of using computers to recognize and understand human speech. Being able to understand full sentences or questions requires a lot of processing power, as it often relies on the complex algorithms found in natural language processing (NLP).
Most microcontrollers (and Arduino boards) cannot run NLP due to their limited resources. However, we can train a neural network to perform basic keyword spotting, which still has many uses (such as enabling a smart speaker by saying “Alexa” or shouting “stop” to halt a machine).
In this video, we will use Edge Impulse to train a neural network to identify and classify a few custom keywords. We will then deploy this trained model to an Arduino Nano 33 BLE Sense to perform keyword spotting in real time.
To begin, we collect samples of the keywords we wish to identify. These can be collected on any number of recording devices and then edited using Audacity to create 1-second snippets. We recommend collecting at least 50 samples to start.
After, we run a custom Python script that mixes the samples with random snippets of background noise and curates the custom keywords along with keywords found in the Google Speech Commands dataset.
From there, we upload our curated dataset to Edge Impulse. We use Edge Impulse as a tool to extract features from the audio samples, which are the Mel frequency cepstral coefficients (MFCCs). We then use it to train a neural network to identify our target keywords. Once done, we can test the model and download it as part of an Arduino library.
We load the library into Arduino and use it to perform inference in real time. The Arduino example code continually captures audio data, extracts features (computes MFCCs), and uses those MFCCs as inputs to the trained model. The model returns (what is essentially) the probabilities that it thinks it heard our target keywords.
We can compare those output values to thresholds to take action whenever it hears the desired keyword! To start, we’ll blink a simple LED (because who doesn’t love an overly complicated blinky program?).
Product Links:
Related Videos:
What is Edge AI?
Intro to TensorFlow Lite Part 1: Wake Word Feature Extraction
Intro to TensorFlow Lite Part 2: Speech Recognition Model Training
Intro to TensorFlow Lite Part 3: Speech Recognition on Raspberry Pi
Getting Started with TensorFlow Lite for Microcontrollers
Related Project Links:
Related Articles:
Getting Started with TensorFlow Lite for Microcontrollers -
Most microcontrollers (and Arduino boards) cannot run NLP due to their limited resources. However, we can train a neural network to perform basic keyword spotting, which still has many uses (such as enabling a smart speaker by saying “Alexa” or shouting “stop” to halt a machine).
In this video, we will use Edge Impulse to train a neural network to identify and classify a few custom keywords. We will then deploy this trained model to an Arduino Nano 33 BLE Sense to perform keyword spotting in real time.
To begin, we collect samples of the keywords we wish to identify. These can be collected on any number of recording devices and then edited using Audacity to create 1-second snippets. We recommend collecting at least 50 samples to start.
After, we run a custom Python script that mixes the samples with random snippets of background noise and curates the custom keywords along with keywords found in the Google Speech Commands dataset.
From there, we upload our curated dataset to Edge Impulse. We use Edge Impulse as a tool to extract features from the audio samples, which are the Mel frequency cepstral coefficients (MFCCs). We then use it to train a neural network to identify our target keywords. Once done, we can test the model and download it as part of an Arduino library.
We load the library into Arduino and use it to perform inference in real time. The Arduino example code continually captures audio data, extracts features (computes MFCCs), and uses those MFCCs as inputs to the trained model. The model returns (what is essentially) the probabilities that it thinks it heard our target keywords.
We can compare those output values to thresholds to take action whenever it hears the desired keyword! To start, we’ll blink a simple LED (because who doesn’t love an overly complicated blinky program?).
Product Links:
Related Videos:
What is Edge AI?
Intro to TensorFlow Lite Part 1: Wake Word Feature Extraction
Intro to TensorFlow Lite Part 2: Speech Recognition Model Training
Intro to TensorFlow Lite Part 3: Speech Recognition on Raspberry Pi
Getting Started with TensorFlow Lite for Microcontrollers
Related Project Links:
Related Articles:
Getting Started with TensorFlow Lite for Microcontrollers -
Комментарии