filmov
tv
How AI Discovered a Faster Matrix Multiplication Algorithm
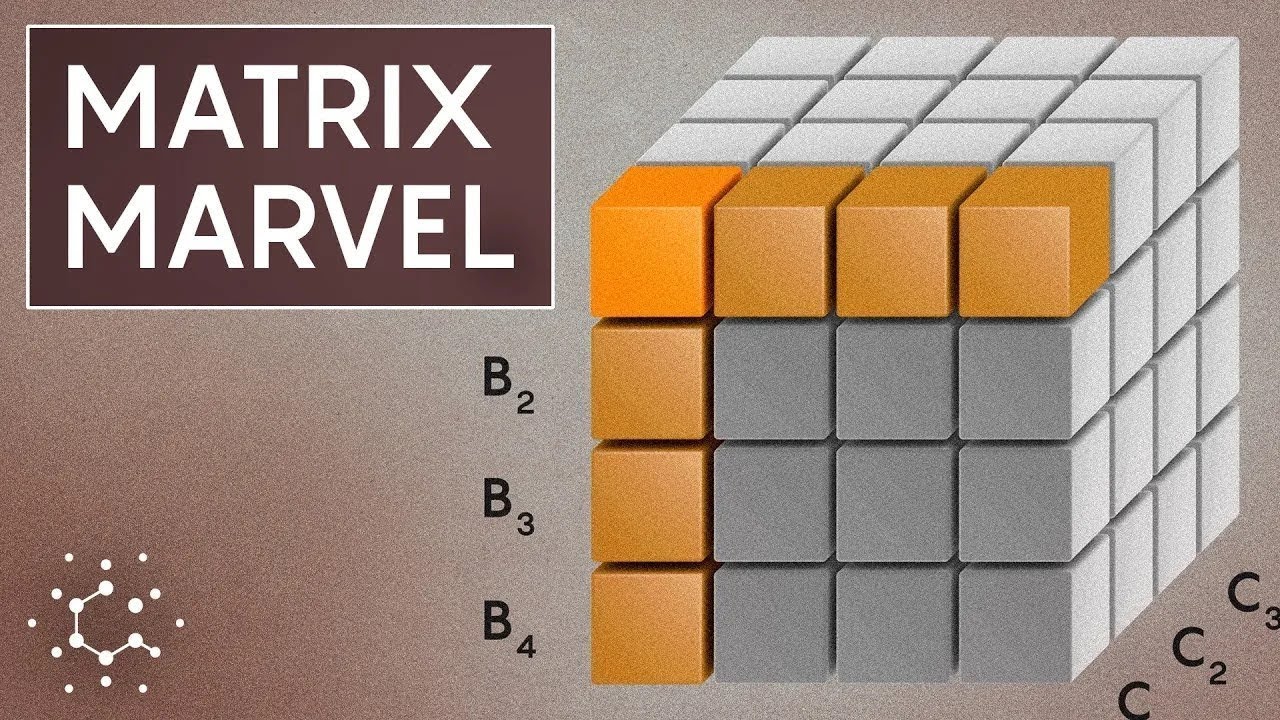
Показать описание
Researchers at Google research lab DeepMind trained an AI system called AlphaTensor to find new, faster algorithms to tackle an age-old math problem: matrix multiplication. Advances in matrix multiplication could lead to breakthroughs in physics, engineering and computer science.
AlphaTensor quickly rediscovered - and surpassed, for some cases - the reigning algorithm discovered by German mathematician Volker Strassen in 1969. However, mathematicians soon took inspiration from the results of the game-playing neural network to make advances of their own.
Correction: At 2:53 in the video, the text previously read "67% less" but has been changed to "67%" for accuracy.
00:00 What is matrix multiplication?
01:06 The standard algorithm for multiplying matrices
02:06 Strassen's faster algorithm for faster matrix multiplication methods
03:55 DeepMind AlphaGo beats a human
04:28 DeepMind uses AI system AlphaTensor to search for new algorithms
05:18 A computer helps prove the four color theorem
06:17 What is a tensor?
07:16 Tensor decomposition explained
08:48 AlphaTensor discovers new and faster faster matrix multiplication algorithms
11:09 Mathematician Manuel Kauers improves on AlphaTensor's results
#math #AlphaTensor #matrices
AlphaTensor quickly rediscovered - and surpassed, for some cases - the reigning algorithm discovered by German mathematician Volker Strassen in 1969. However, mathematicians soon took inspiration from the results of the game-playing neural network to make advances of their own.
Correction: At 2:53 in the video, the text previously read "67% less" but has been changed to "67%" for accuracy.
00:00 What is matrix multiplication?
01:06 The standard algorithm for multiplying matrices
02:06 Strassen's faster algorithm for faster matrix multiplication methods
03:55 DeepMind AlphaGo beats a human
04:28 DeepMind uses AI system AlphaTensor to search for new algorithms
05:18 A computer helps prove the four color theorem
06:17 What is a tensor?
07:16 Tensor decomposition explained
08:48 AlphaTensor discovers new and faster faster matrix multiplication algorithms
11:09 Mathematician Manuel Kauers improves on AlphaTensor's results
#math #AlphaTensor #matrices
Комментарии