filmov
tv
Introduction to Structural Equation Modeling
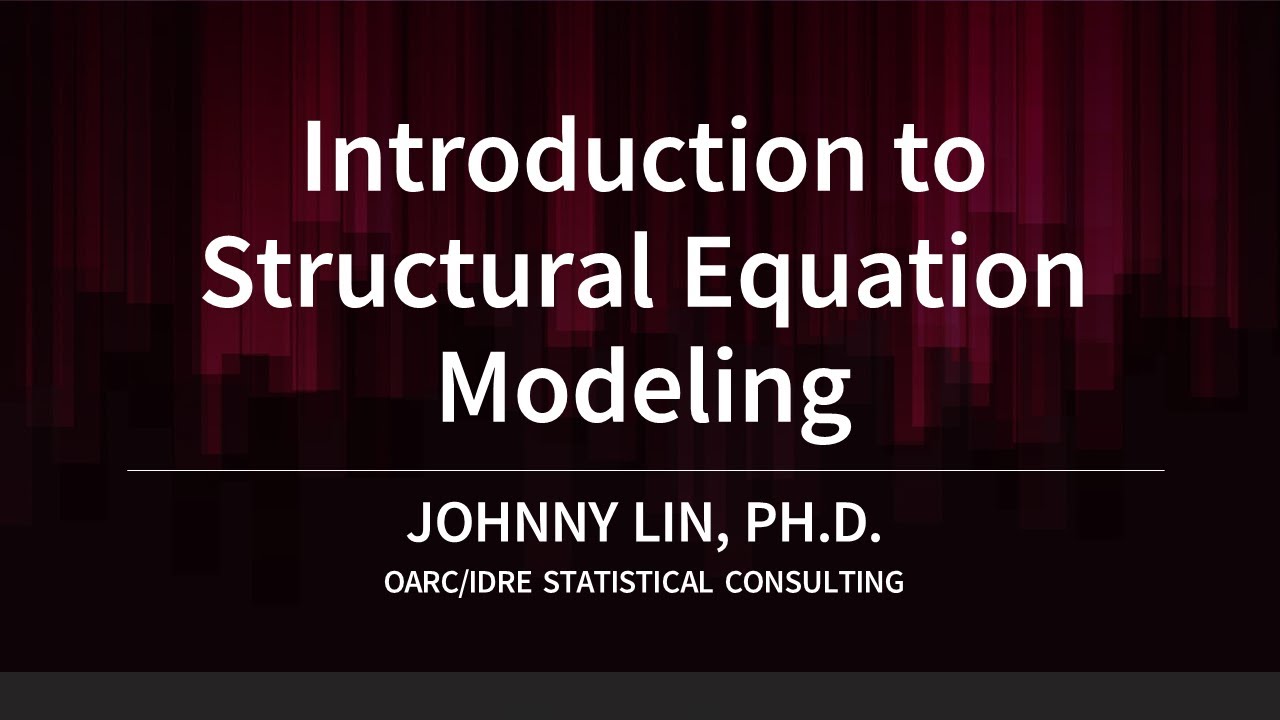
Показать описание
Introduction to SEM seminar originally given on February 22, 2021.
This is the second seminar in a three-part series.
1. Confirmatory Factor Analysis (CFA) in R with lavaan
The first seminar introduces the confirmatory factor analysis model, and discusses model identification, degrees of freedom and model fit.
2. Introduction to Structural Equation Modeling (SEM) in R with lavaan
The second seminar explores structural equation models which is an umbrella term that encompass linear regression, multivariate regression, path analysis, CFA and structural regression.
3. Latent Growth Models (LGM) and Measurement Invariance with R in lavaan
The third seminar introduces latent growth modeling and how it relates to hierarchical linear models (HLM) and 2) measurement invariance in CFA and how to compare model fit between invariance models.
This is the second seminar in a three-part series.
1. Confirmatory Factor Analysis (CFA) in R with lavaan
The first seminar introduces the confirmatory factor analysis model, and discusses model identification, degrees of freedom and model fit.
2. Introduction to Structural Equation Modeling (SEM) in R with lavaan
The second seminar explores structural equation models which is an umbrella term that encompass linear regression, multivariate regression, path analysis, CFA and structural regression.
3. Latent Growth Models (LGM) and Measurement Invariance with R in lavaan
The third seminar introduces latent growth modeling and how it relates to hierarchical linear models (HLM) and 2) measurement invariance in CFA and how to compare model fit between invariance models.
Structural Equation Modeling: what is it and what can we use it for? (part 1 of 6)
SEM (1): What is Structural Equation Modelling and when to use it?
Introduction to Structural Equation Modeling
SEM Episode 1: Introduction to Structural Equation Models
Introduction to Structural Equation Modeling
Introduction to Structural Equation Modeling
Introduction to Structural Equation Modeling, Part 1: Overview
Introduction to Structural Equation Modeling (Lecture 1) | www.pietutors.com
Introduction to Structural Equation Modeling (Lecture 1) | www.pietutors.com
A Gentle Introduction to Structural Equation Modelling
Introduction to Structural Equation Modeling method
Introduction to Structural Equation Modeling
Mild introduction to Structural Equation Modeling (SEM) using R
A Quick Overview of Structural Equation Modeling
Analyze Structural Equation Models in Two Steps
Intro to Structural Equation Modeling Using Stata
01 Structural Equation Modelling(SEM)(AMOS)(introduction)
Introduction to Structural Equation Modeling (Chapter 12 Lecture 1)
Introduction to Structural Equation Modeling (SEM) with Levi Littvay
Structural Equation Models and Latent Variables: An Introduction
Introduction to Structural Equation Modeling
SEM Workshop 1 of 4 : Introduction to Structural Equation Modeling
What is Structural Equation Modeling?
1. SPSS AMOS - Understanding the Fundamentals of Structural Equation Modelling - Research Coach
Комментарии