filmov
tv
Distributed and Decentralized Learning - Ce Zhang | Stanford MLSys #68
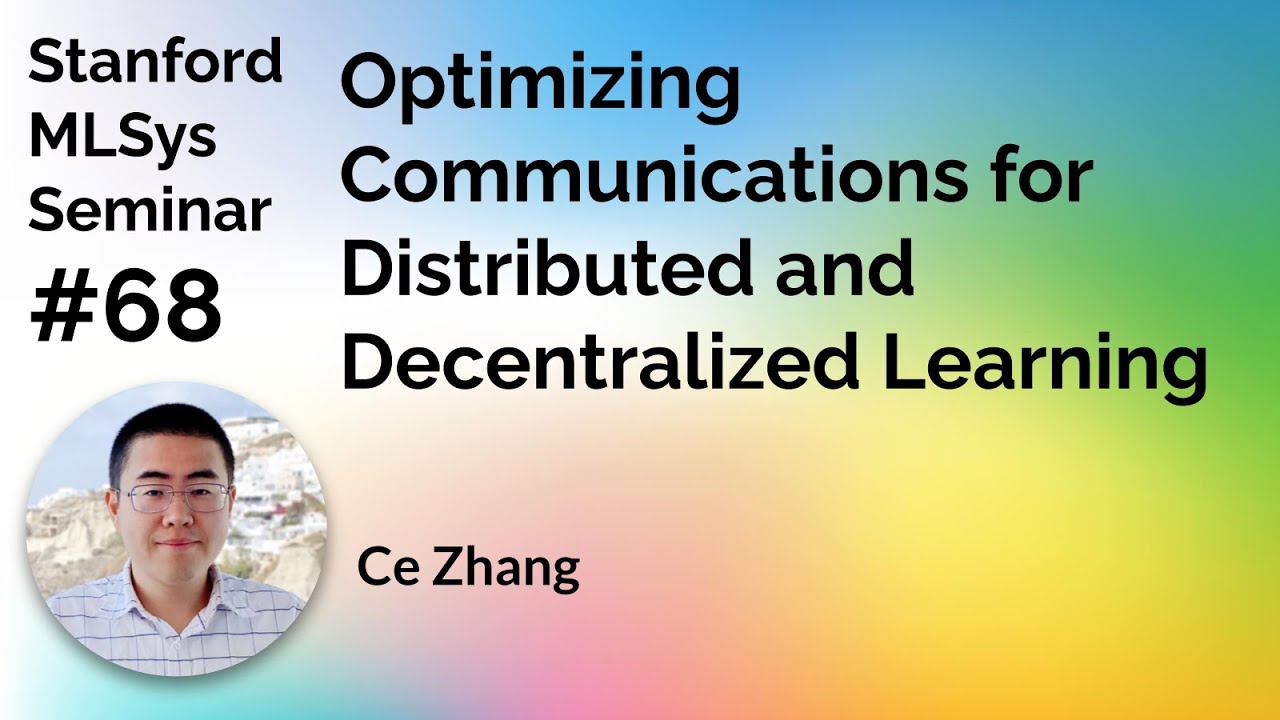
Показать описание
Episode 68 of the Stanford MLSys Seminar “Foundation Models Limited Series”!
Speaker: Ce Zhang
Abstract:
The rapid progress of machine learning in the last decade has been fueled by the increasing scale of data and compute. Today's training algorithms are often communication heavy, as a result, large-scale models are trained dominantly in a centralized environment such as data centers with fast network connections. This strong dependency on fast interconnections is becoming the limiting factor of further scaling, not only for the data center setting but also for alternative decentralized infrastructures such as spot instances and geo-distributed volunteer computes. In this talk, I will discuss our research in communication-efficient distributed learning and our current effort in training foundation models in a decentralized way.
Bio:
Ce is an Assistant Professor in Computer Science at ETH Zurich. The mission of his research is to make machine learning techniques widely accessible---while being cost-efficient and trustworthy---to everyone who wants to use them to make our world a better place. He believes in a system approach to enabling this goal, and his current research focuses on building next-generation machine learning platforms and systems that are data-centric, human-centric, and declaratively scalable. Before joining ETH, Ce finished his PhD at the University of Wisconsin-Madison and spent another year as a postdoctoral researcher at Stanford, both advised by Christopher Ré. His work has received recognitions such as the SIGMOD Best Paper Award, SIGMOD Research Highlight Award, Google Focused Research Award, an ERC Starting Grant, and has been featured and reported by Science, Nature, the Communications of the ACM, and a various media outlets such as Atlantic, WIRED, Quanta Magazine, etc.
Speaker: Ce Zhang
Abstract:
The rapid progress of machine learning in the last decade has been fueled by the increasing scale of data and compute. Today's training algorithms are often communication heavy, as a result, large-scale models are trained dominantly in a centralized environment such as data centers with fast network connections. This strong dependency on fast interconnections is becoming the limiting factor of further scaling, not only for the data center setting but also for alternative decentralized infrastructures such as spot instances and geo-distributed volunteer computes. In this talk, I will discuss our research in communication-efficient distributed learning and our current effort in training foundation models in a decentralized way.
Bio:
Ce is an Assistant Professor in Computer Science at ETH Zurich. The mission of his research is to make machine learning techniques widely accessible---while being cost-efficient and trustworthy---to everyone who wants to use them to make our world a better place. He believes in a system approach to enabling this goal, and his current research focuses on building next-generation machine learning platforms and systems that are data-centric, human-centric, and declaratively scalable. Before joining ETH, Ce finished his PhD at the University of Wisconsin-Madison and spent another year as a postdoctoral researcher at Stanford, both advised by Christopher Ré. His work has received recognitions such as the SIGMOD Best Paper Award, SIGMOD Research Highlight Award, Google Focused Research Award, an ERC Starting Grant, and has been featured and reported by Science, Nature, the Communications of the ACM, and a various media outlets such as Atlantic, WIRED, Quanta Magazine, etc.
Комментарии