filmov
tv
Real-World Dataset Cleaning with Python Pandas! (Olympic Athletes Dataset)
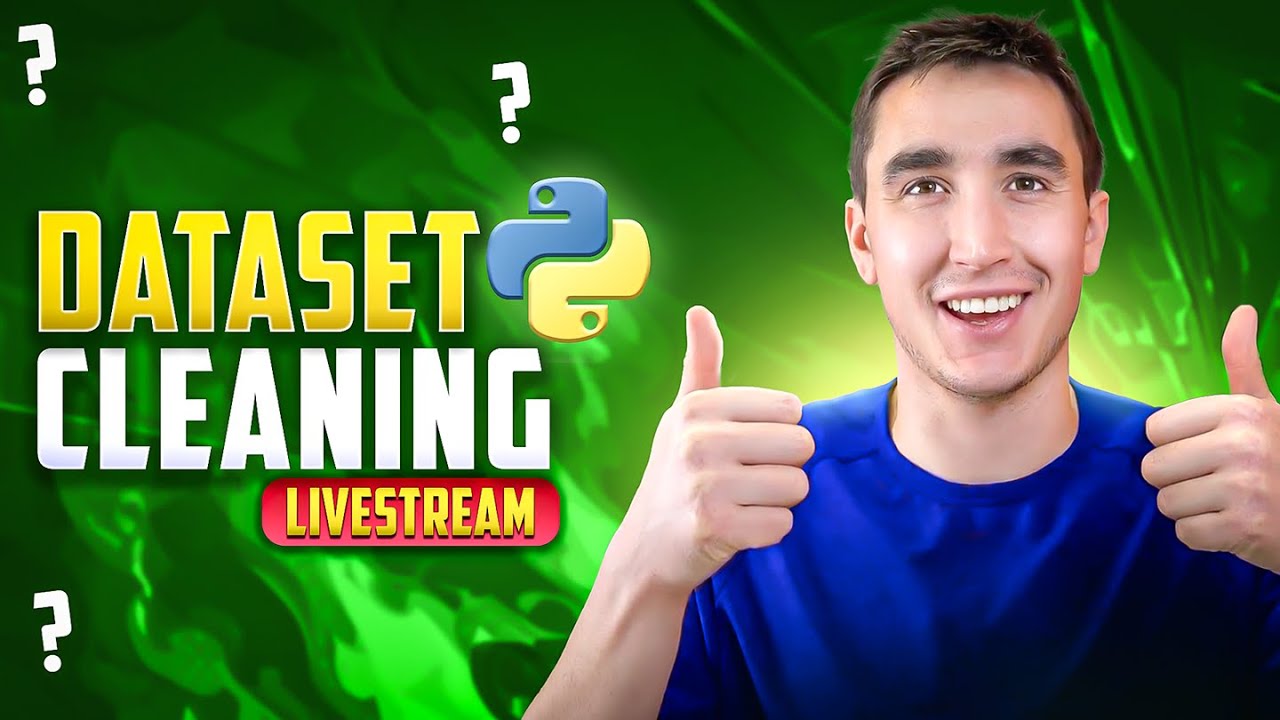
Показать описание
I'm prepping a dataset for an upcoming tutorial and I figured walking through the process of cleaning it would work well for a livestream! We use various Python Pandas functions to accomplish our data cleaning goals.
We'll be working off of this repo:
Some topics that we cover:
- How you can use web scraping to collect data like this (Python beautifulsoup).
- Splitting strings into separate columns
- Using regular expressions (regexes) to extract specific details from columns
- Converting columns to datetime & numeric types
- Grabbing only a subset of our columns
Sorry that this was a bit last minute scheduling-wise, will try to give more advance notice in the future!
Video timeline!
0:00 - Livestream Overview
4:00 - About the Olympics dataset (source website and how it was scraped)
9:50 - Cleaning the dataset (getting started with code & data)
19:26 - What aspects of our data should be cleaned?
29:08 - Get rid of bullet points in Used name column
34:08 - How to split Measurements into two separate height/weight numeric columns.
1:05:00 - Parse out dates from Born & Died columns
1:25:43 - Parse out city, region, and country from Born column (working with regular expressions)
1:41:15 - Get rid of the extra columns
1:49:41 - Questions & Answers
-------------------------
Follow me on social media!
-------------------------
Practice your Python Pandas data science skills with problems on StrataScratch!
Join the Python Army to get access to perks!
*I use affiliate links on the products that I recommend. I may earn a purchase commission or a referral bonus from the usage of these links.
We'll be working off of this repo:
Some topics that we cover:
- How you can use web scraping to collect data like this (Python beautifulsoup).
- Splitting strings into separate columns
- Using regular expressions (regexes) to extract specific details from columns
- Converting columns to datetime & numeric types
- Grabbing only a subset of our columns
Sorry that this was a bit last minute scheduling-wise, will try to give more advance notice in the future!
Video timeline!
0:00 - Livestream Overview
4:00 - About the Olympics dataset (source website and how it was scraped)
9:50 - Cleaning the dataset (getting started with code & data)
19:26 - What aspects of our data should be cleaned?
29:08 - Get rid of bullet points in Used name column
34:08 - How to split Measurements into two separate height/weight numeric columns.
1:05:00 - Parse out dates from Born & Died columns
1:25:43 - Parse out city, region, and country from Born column (working with regular expressions)
1:41:15 - Get rid of the extra columns
1:49:41 - Questions & Answers
-------------------------
Follow me on social media!
-------------------------
Practice your Python Pandas data science skills with problems on StrataScratch!
Join the Python Army to get access to perks!
*I use affiliate links on the products that I recommend. I may earn a purchase commission or a referral bonus from the usage of these links.
Комментарии