filmov
tv
Skewness and Kurtosis with SPSS Tutorial (SPSS Tutorial Video #11)
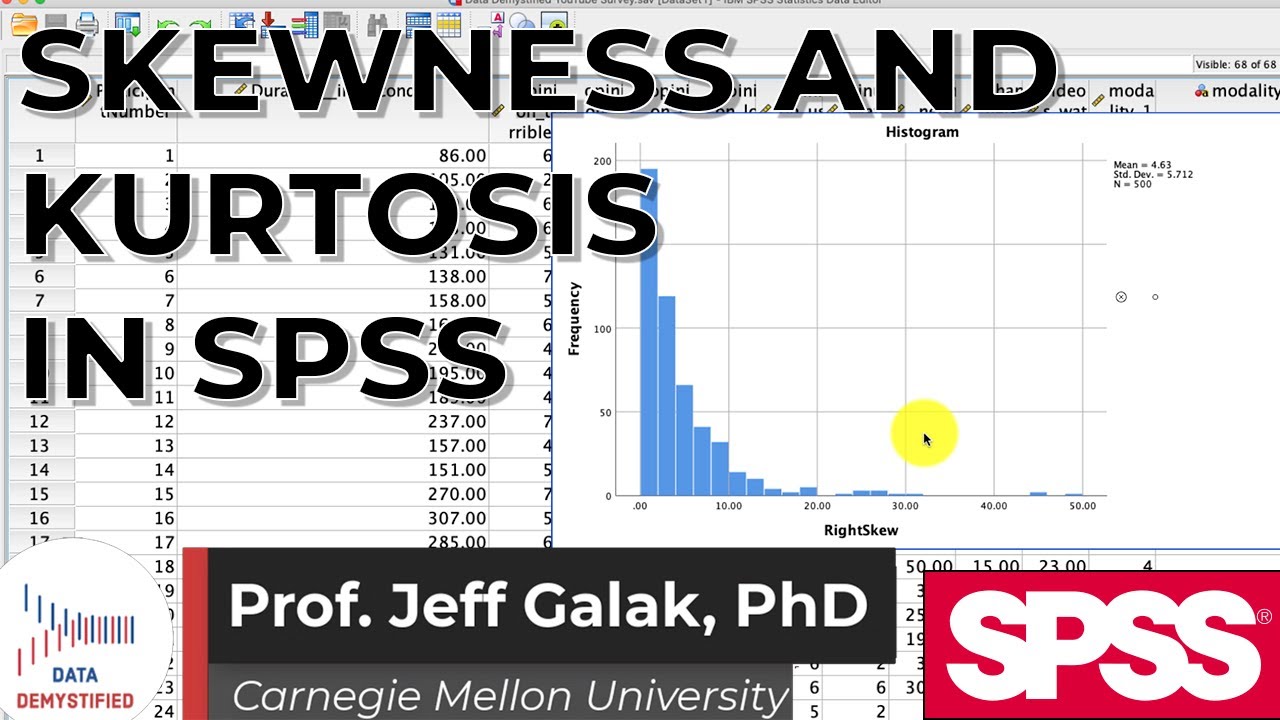
Показать описание
In this video, I show you how to determine and interpret the SKEWNESS and KURTOSIS of a distribution. These are two useful metrics for describing the shape of a distribution. I also give you a chance to try and to it yourself.
This SPSS tutorial series is designed to teach you the basics of how to analyze and interpret the results of data using SPSS. I will cover everything from the very basics of the main windows within SPSS, to manipulating data, to running and interpreting meaningful analyses like t-tests, ANOVA, regression, and many more, and visualizing results.
Follow me at:
Equipment Used for Filming:
This SPSS tutorial series is designed to teach you the basics of how to analyze and interpret the results of data using SPSS. I will cover everything from the very basics of the main windows within SPSS, to manipulating data, to running and interpreting meaningful analyses like t-tests, ANOVA, regression, and many more, and visualizing results.
Follow me at:
Equipment Used for Filming:
Skewness and Kurtosis with SPSS Tutorial (SPSS Tutorial Video #11)
SPSS (7): Descriptive Statistics | Mean, Range, Standard Deviation, Skewness, Kurtosis
Skewness - SPSS (part 1)
V2.7 - How to calculate kurtosis - SPSS (Sleep Data)
SPSS: Skew and Kurtosis (Non-Normal Distributions) - 3 different ways
Schiefe und Kurtosis in SPSS - Test auf Normalverteilung der Daten - Daten analysieren in SPSS (34)
Skewness and Kurtosis in SPSS
V6 17 - Skew, kurtosis & histograms across groups in SPSS
Nonparametric Statistics by Corder/Foreman C2 find Skewness and Kurtosis with SPSS
Calculating Skewness and Kurtosis Coefficients in IBM SPSS
SPSS Skewness & Kurtosis
Kurtosis in SPSS exploring normal distribution of data
Checking normality using skewness, kurtosis, Kolmogorov–Smirnov and Shapiro-Wilk tests
SD, Variance, Skew, Kurtosis and Standardized Scores in SPSS
An example Descriptive Statistics, Skew And Kurtosis in SPSS [SPSS] [Descriptive Statistics]
Skewness And Kurtosis And Moments | What Is Skewness And Kurtosis? | Statistics | Simplilearn
What is Skewness & Kurtosis ? | Difference Between Skewness and Kurtosis in Statistics
Kurtosis - SPSS (part 1)
SPSS - Mean, Median, Mode, Standard Deviation & Range
Normality Tests for Skewness And Kurtosis With SPSS
Data Transformation for Positively and Negatively Skewed Distributions in SPSS
How to check Data Normality, calculate and interpret descriptive statistics in SPSS (Lesson 7)
Normallik Testi SPSS, Normal dağılım testi, Normal Dağılmayan Veri için Çözümler
How to conduct descriptive analysis in SPSS || Mean / Median / Mode / SD / Skew & Kurtosis
Комментарии