filmov
tv
Invariance and Stability to Deformations of Deep Convolutional Representations
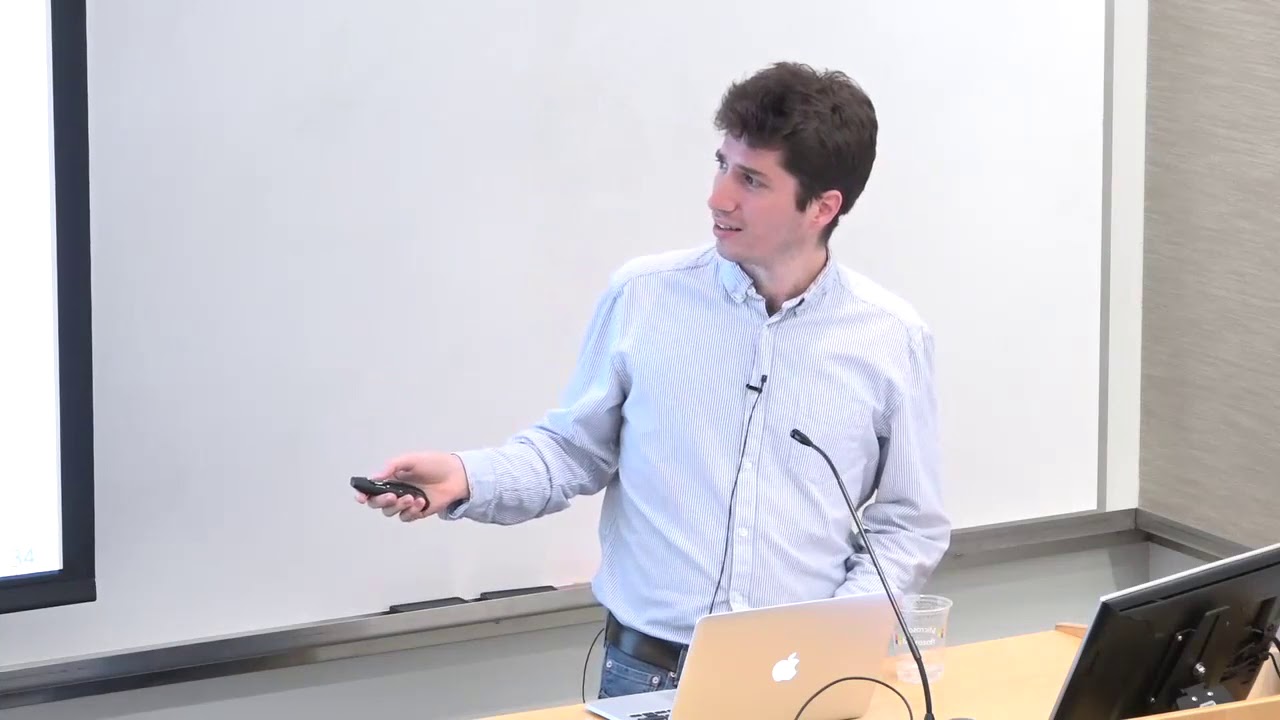
Показать описание
The success of deep convolutional architectures is often attributed in part to their ability to learn multiscale and invariant representations of natural signals. However, a precise study of these properties and how they affect learning guarantees is still missing. In this talk, we consider deep convolutional representations of signals; we study their invariance to translations and to more general groups of transformations, their stability to the action of diffeomorphisms, and their ability to preserve signal information. This analysis is carried by introducing a multilayer kernel based on convolutional kernel networks and by studying the geometry induced by the kernel mapping. We then characterize the corresponding reproducing kernel Hilbert space (RKHS), showing that it contains a large class of convolutional neural networks with smooth activation functions. This analysis allows us to separate data representation from learning, and to provide a canonical measure of model complexity, the RKHS norm, which controls both stability and generalization of any learned model. This theory also leads to new practical regularization strategies for deep learning that are effective when learning on small datasets, or to obtain adversarially robust models.
Invariance and Stability to Deformations of Deep Convolutional Representations
Stéphane Mallat - High Dimensional Classification with Invariant Deep Networks
05 Imperial's Deep learning course: Equivariance and Invariance
Michael Perlmutter, Group Invariant Scattering on Graphs, Manifolds, and Other Spaces, 2022.09.06
Learning multiscale invariants from big data for physics - Stéphane Mallat
Professor Stéphane Mallat: 'High-Dimensional Learning and Deep Neural Networks'
G-invariant Neural Networks (part 1)
Nicolò Zava (3/17/23): Every stable invariant of finite metric spaces produces false positives
The convolution is not shift invariant. | Invariance vs Equivariance | ❓ #AICoffeeBreakQuiz #Shorts...
Signal and Image Classification - Stephane Mallat Technion lecture
Scattering Bricks to Build Invariants for Perception [part 1]
Benjamin Bloem-Reddy: Probabilistic Symmetry and Invariant Neural Networks
Why Convolutional Neural Networks Are Not Permuation Invariant
Modularity of DT invariants on smooth K3 fibrations (Part 1)
Stéphane Mallat: Hamiltonian Estimations by Conditional Renormalisation Group and Convolution Nets
Invariant (mathematics)
26 Invariance of domain
Lecture 16: Isometry invariance and spectral techniques
Joan Bruna: 'On Computational Hardness with Graph Neural Networks'
Neurally plausible mechanisms for learning selective and invariant representations - Fabio Anselmi
Rotation equivariant and invariant neural networks... - Benjamin Chidester - MLCSB - ISMB/ECCB 2019
Invariance of Domain
Invariant and Selective Representations in Visual Cortex
Alexander Zamolodchikov (Stony Brook University): T-Tbar Deformations of 2D Quantum Field Theories 1
Комментарии