filmov
tv
Benjamin Bloem-Reddy: Probabilistic Symmetry and Invariant Neural Networks
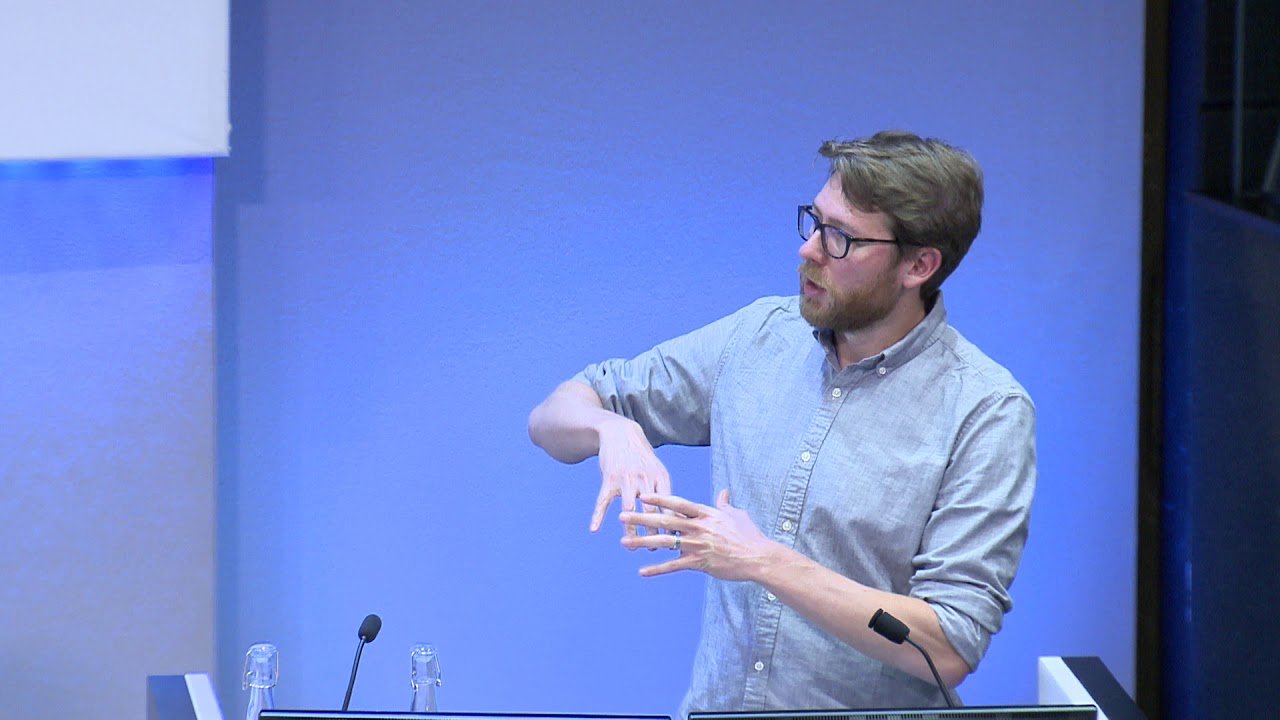
Показать описание
In an effort to improve the performance of deep neural networks in data-scarce, non-i.i.d., or unsupervised settings, much recent research has been devoted to encoding invariance under symmetry transformations into neural network architectures. We treat the neural network input and output as random variables, and consider group invariance from the perspective of probabilistic symmetry---a field with a long history, dating back at least to Laplace. Drawing on tools from probability and statistics, we establish a link between functional and probabilistic symmetry, and obtain functional representations of probability distributions that encode symmetry under the action of a compact group. Those representations characterize the structure of neural networks that can be used to represent such distributions and yield a general program for constructing invariant stochastic or deterministic neural networks. We develop the details of the general program for exchangeable sequences and arrays, recovering a number of recent examples as special cases.
Bio: Benjamin Bloem-Reddy is a postdoctoral researcher in the Computational Statistics and Machine Learning group, led by Yee Whye Teh, at the University of Oxford. Ben obtained his PhD in Statistics from Columbia University. His research has focused on probabilistic and statistical methodology for analysis of discrete data like graphs, partitions, and permutations. Natural applications of these ideas arise in, for example, modeling networks or text, and in matrix factorization. Recently, he has also worked on problems involving probabilistic symmetry in neural networks and in probabilistic programming. In summer 2019, he will join the faculty in the Department of Statistics at the University of British Columbia.
*Sponsors*
Man AHL: At Man AHL, we mix machine learning, computer science and engineering with terabytes of data to invest billions of dollars every day.
Bio: Benjamin Bloem-Reddy is a postdoctoral researcher in the Computational Statistics and Machine Learning group, led by Yee Whye Teh, at the University of Oxford. Ben obtained his PhD in Statistics from Columbia University. His research has focused on probabilistic and statistical methodology for analysis of discrete data like graphs, partitions, and permutations. Natural applications of these ideas arise in, for example, modeling networks or text, and in matrix factorization. Recently, he has also worked on problems involving probabilistic symmetry in neural networks and in probabilistic programming. In summer 2019, he will join the faculty in the Department of Statistics at the University of British Columbia.
*Sponsors*
Man AHL: At Man AHL, we mix machine learning, computer science and engineering with terabytes of data to invest billions of dollars every day.