filmov
tv
Learning with Marginalized Corrupted Features - Kilian Weinberger (U of Washington, St. Louis)-2013
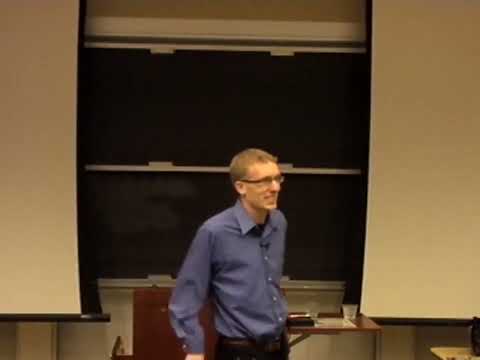
Показать описание
Abstract
If infinite amounts of labeled data are provided, many machine learning algorithms become perfect. With finite amounts of data, regularization or priors have to be used to introduce bias into a classifier. We propose a third option: learning with marginalized corrupted features. We (implicitly) corrupt existing data as a means to generate additional, infinitely many, training samples from a slightly different data distribution — this is computationally tractable because the corruption can be marginalized out in the closed form. Our framework leads to machine learning algorithms that are fast, generalize well and naturally scale to very large data sets. We showcase this technology as regularization for general risk minimization and for marginalized deep learning for document representations. We provide experimental results on part of speech tagging as well as document and image classification.
Biography
Kilian Q. Weinberger is an Assistant Professor in the Department of Computer Science & Engineering at Washington University in St. Louis. He received his Ph.D. from the University of Pennsylvania in Machine Learning under the supervision of Lawrence Saul. Prior to this, he obtained his undergraduate degree in Mathematics and Computer Science at the University of Oxford. During his career, he has won several best paper awards at ICML, CVPR, and AISTATS. In 2011 he was awarded the AAAI senior program chair award and in 2012 he received the NSF CAREER award. Kilian Weinberger’s research is in Machine Learning and its applications. In particular, he focuses on high-dimensional data analysis, metric learning, machine-learned web-search ranking, transfer- and multi-task learning as well as biomedical applications.
If infinite amounts of labeled data are provided, many machine learning algorithms become perfect. With finite amounts of data, regularization or priors have to be used to introduce bias into a classifier. We propose a third option: learning with marginalized corrupted features. We (implicitly) corrupt existing data as a means to generate additional, infinitely many, training samples from a slightly different data distribution — this is computationally tractable because the corruption can be marginalized out in the closed form. Our framework leads to machine learning algorithms that are fast, generalize well and naturally scale to very large data sets. We showcase this technology as regularization for general risk minimization and for marginalized deep learning for document representations. We provide experimental results on part of speech tagging as well as document and image classification.
Biography
Kilian Q. Weinberger is an Assistant Professor in the Department of Computer Science & Engineering at Washington University in St. Louis. He received his Ph.D. from the University of Pennsylvania in Machine Learning under the supervision of Lawrence Saul. Prior to this, he obtained his undergraduate degree in Mathematics and Computer Science at the University of Oxford. During his career, he has won several best paper awards at ICML, CVPR, and AISTATS. In 2011 he was awarded the AAAI senior program chair award and in 2012 he received the NSF CAREER award. Kilian Weinberger’s research is in Machine Learning and its applications. In particular, he focuses on high-dimensional data analysis, metric learning, machine-learned web-search ranking, transfer- and multi-task learning as well as biomedical applications.