filmov
tv
Lesson 4: Deep Learning 2018
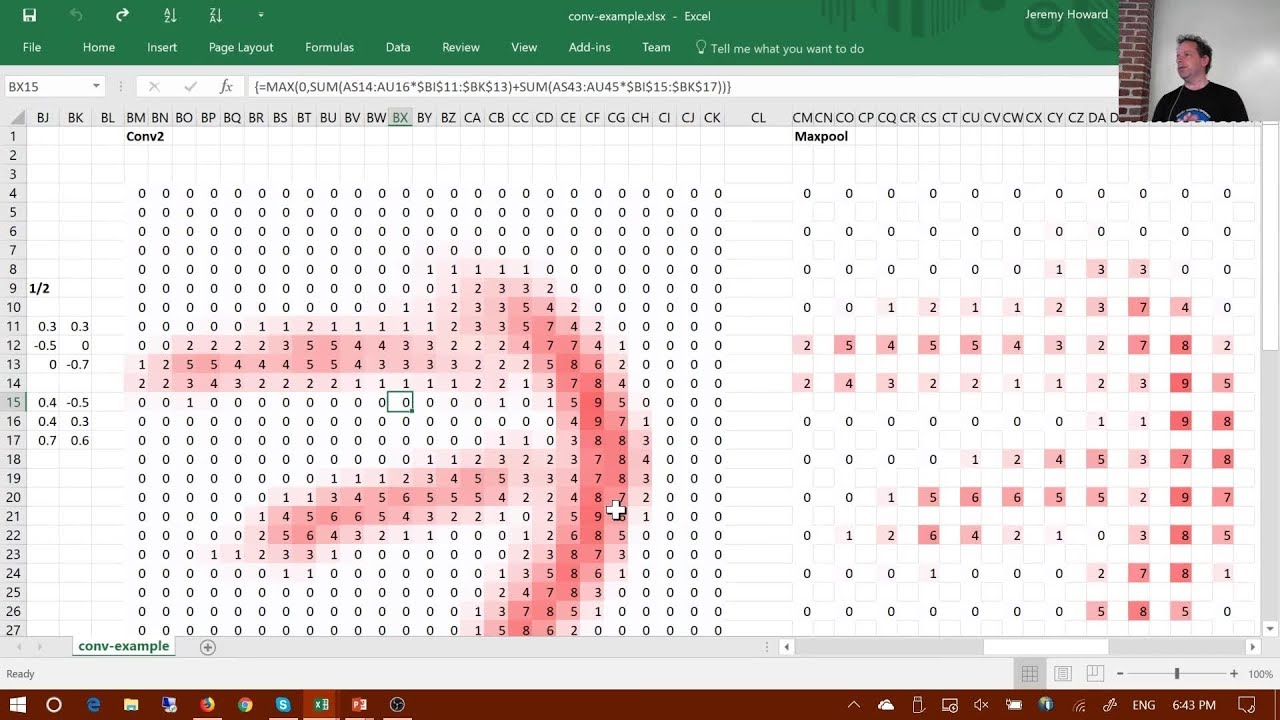
Показать описание
We complete our work from the previous lesson on tabular/structured, time-series data, and learn about how to avoid overfitting by using dropout regularization. We then introduce natural language processing with recurrent neural networks, and start work on a language model.
Lesson 4: Deep Learning 2018
Intro to Deep Learning 2018 - Lesson 4
Lesson 4: Deep Learning 2019 - NLP; Tabular data; Collaborative filtering; Embeddings
Lesson 4 - Deep Learning for Coders (2020)
Lesson 4: Practical Deep Learning for Coders 2022
Lesson 4: Practical Deep Learning for Coders
#18 Machine Learning Specialization [Course 1, Week 1, Lesson 4]
Intro to Machine Learning: Lesson 4
1 - 4 Lesson 4 Deep Learning 2019 NLP; Tabular data; Collaborative filtering; Embeddings
3 - 4 Lesson 4 Deep Learning 2019 NLP; Tabular data; Collaborative filtering; Embeddings
Lesson 3: Deep Learning 2018
Lesson 5: Deep Learning 2018
Deep Learning | What is Deep Learning? | Deep Learning Tutorial For Beginners | 2023 | Simplilearn
Lesson 9: Deep Learning Part 2 2018 - Multi-object detection
Lesson 6: Deep Learning 2018
Lesson 10: Deep Learning Part 2 2018 - NLP Classification and Translation
2 - 4 Lesson 4 Deep Learning 2019 NLP; Tabular data; Collaborative filtering; Embeddings
#38 Machine Learning Specialization [Course 1, Week 3, Lesson 4]
#40 Machine Learning Specialization [Course 1, Week 3, Lesson 4]
TWiML & AI x Fast.ai Machine Learning Study Group – Session 4 – October 28, 2018
Lesson 1: Deep Learning 2018
Lesson 6 - Deep Learning for Coders (2020)
#39 Machine Learning Specialization [Course 1, Week 3, Lesson 4]
#16 Machine Learning Specialization [Course 1, Week 1, Lesson 4]
Комментарии