filmov
tv
Simplify and Scale Data Engineering Pipelines with Delta Lake
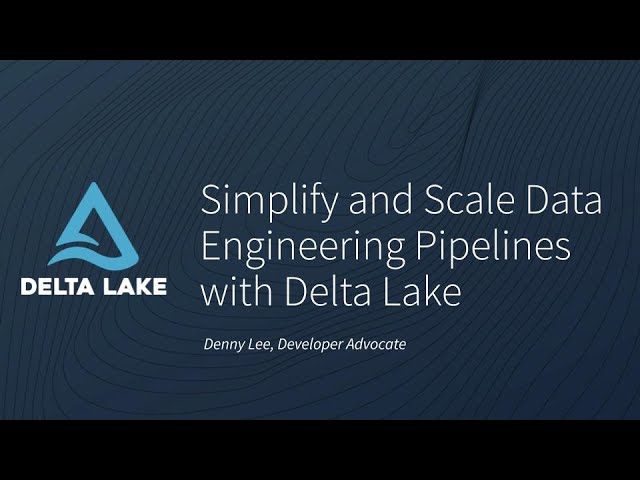
Показать описание
Online Tech Talk with Denny Lee, Developer Advocate @ Databricks
A common data engineering pipeline architecture uses tables that correspond to different quality levels, progressively adding structure to the data: data ingestion (“Bronze” tables), transformation/feature engineering (“Silver” tables), and machine learning training or prediction (“Gold” tables). Combined, we refer to these tables as a “multi-hop” architecture. It allows data engineers to build a pipeline that begins with raw data as a “single source of truth” from which everything flows. In this session, we will show how to build a scalable data engineering data pipeline using Delta Lake.
Delta Lake is an open-source storage layer that brings reliability to data lakes. Delta Lake offers ACID transactions, scalable metadata handling, and unifies streaming and batch data processing. It runs on top of your existing data lake and is fully compatible with Apache Spark APIs.
In this session you will learn about:
- The data engineering pipeline architecture
- Data engineering pipeline scenarios
- Data engineering pipeline best practices
- How Delta Lake enhances data engineering pipelines
- The ease of adopting Delta Lake for building your data engineering pipelines
See full Getting Started with Delta Lake tutorial series here:
A common data engineering pipeline architecture uses tables that correspond to different quality levels, progressively adding structure to the data: data ingestion (“Bronze” tables), transformation/feature engineering (“Silver” tables), and machine learning training or prediction (“Gold” tables). Combined, we refer to these tables as a “multi-hop” architecture. It allows data engineers to build a pipeline that begins with raw data as a “single source of truth” from which everything flows. In this session, we will show how to build a scalable data engineering data pipeline using Delta Lake.
Delta Lake is an open-source storage layer that brings reliability to data lakes. Delta Lake offers ACID transactions, scalable metadata handling, and unifies streaming and batch data processing. It runs on top of your existing data lake and is fully compatible with Apache Spark APIs.
In this session you will learn about:
- The data engineering pipeline architecture
- Data engineering pipeline scenarios
- Data engineering pipeline best practices
- How Delta Lake enhances data engineering pipelines
- The ease of adopting Delta Lake for building your data engineering pipelines
See full Getting Started with Delta Lake tutorial series here:
Комментарии