filmov
tv
10-01. Stochastic processes - Filtrations, martingales and Markov chains.
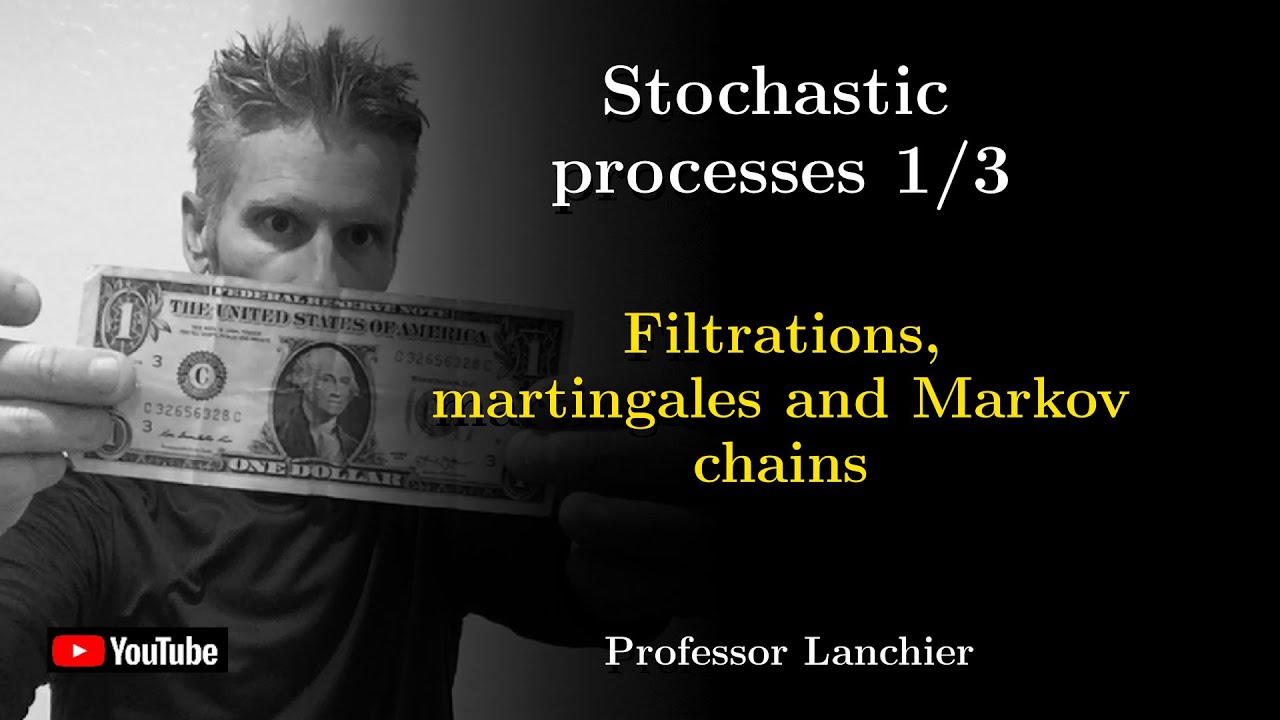
Показать описание
In this video, we define the general concept of stochastic process. We also define the concept of filtration in the context of discrete-time stochastic processes, as well as martingales and Markov chains, and explain their interpretation. These definitions rely on the notions of random variable and conditional expectation defined in previous videos. This is Chapter 4 of my Stochastic Modeling book.
10-01. Stochastic processes - Filtrations, martingales and Markov chains.
Stochastic Process, Filtration | Part 1 Stochastic Calculus for Quantitative Finance
EL6333 Lect 10 April 2014 'Stationary Stochastic Processes to LTI systems, Poisson Processes&ap...
5. Stochastic Processes I
35.1 Stochastic Processes
Stochastic Processes part 1
Hao Ni, High Rank Path Development: an approach of learning the filtration of stochastic processes
Mathematical Finance L 2-1: Stochastic processes
Stochastic Process
25-Random Variables & Stochastic Processes: Filtering Stochastic Processes
Pillai EL6333 Lecture 9 April 10, 2014 'Introduction to Stochastic Processes'
stochasticprocesses 1
BMA4104: STOCHASTIC PROCESSES Lesson 1
Martingales
Measuring properties of stochastic processes
Pillai Grad Lecture 10A 'Power Spectrum of Stationary Stochastic Processes' (1/2)
Stochastic Processes -- Lecture 10
Pillai: Grad Lecture 10B 'Power Spectrum of Stationary Stochastic Processes' (2/2)
Stochastic Process Exam Help
Filtration and Natural Filtration
#1-Random Variables & Stochastic Processes: History
#17-Random Variables & Stochastic Processes: Stochastic Processes
Stochastic Processes
Stochastic Processes: LECTURE 1
Комментарии