filmov
tv
DAY - 1 | Introduction to Generative AI Community Course LIVE ! #genai #ineuron
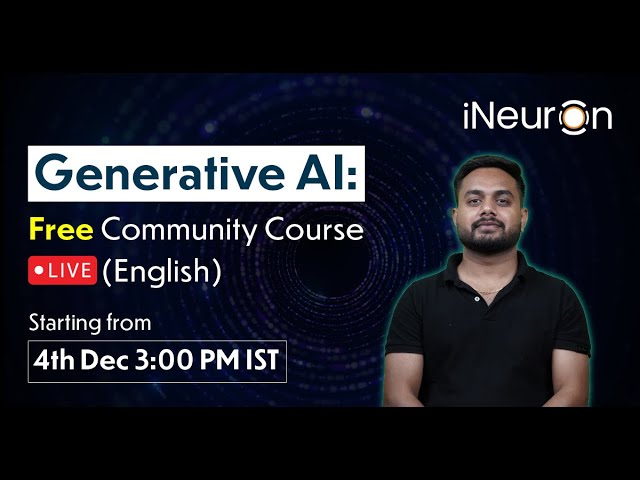
Показать описание
Day - 1 | Introduction to Generative AI
The moment we've all been eagerly waiting for is finally here! Today marks the commencement of our much-anticipated course on GENERATIVE AI. 🎉
We'll be diving into fascinating topics like LLM, LlamaIndex, Vector DB, and much more. It's your chance to embark on a comprehensive exploration of cutting-edge concepts.
Connect with us on : +91 8071176111
Social Media Links:-
The moment we've all been eagerly waiting for is finally here! Today marks the commencement of our much-anticipated course on GENERATIVE AI. 🎉
We'll be diving into fascinating topics like LLM, LlamaIndex, Vector DB, and much more. It's your chance to embark on a comprehensive exploration of cutting-edge concepts.
Connect with us on : +91 8071176111
Social Media Links:-
First Day of Class teacher introduction activities, ideas and icebreakers
Day 1 Workout. Introduction.
Introductions | Beginner English | How to Introduce yourself in English
FIRST DAY OF CLASS TEACHER INTRODUCTION ACTIVITIES, TIPS, CREATIVE WAYS AND IDEAS 2021
Blender Day 1 - Absolute Basics - Introduction Series for Beginners ( compatible with 4.1)
How to Give a 60 Second Self-Introduction Presentation
Day 1 Coding Beginners Module | Introduction
Learn to Sew Introduction | Day 1 of 30 Day Challenge | SEWING FOR BEGINNERS
Introduction | Day 1 | Data Structures and Algorithms | Mindslate Learning
(ENGLISH LANGUAGE COURSE DAY 1) How to give introduction In English
Self-Introduction Video for ESL Teaching| Self-Introduction in English| Online English Teacher| Leri
Kapenda's Vlog Day 1: Introduction
Learn German for Beginners - German A1 - Session 1 - Introduction to German
Introduction to Salesforce Flow Builder | Day 1
How to Introduce Yourself as a Teacher with Students | Teacher Self Introduction in school
Introduction to Programming & Python | Python Tutorial - Day #1
Self Introduction | English Cafe
Introduction - Japanese Lesson 1
First Day of School | CoComelon Nursery Rhymes & Kids Songs
day 1 introduction
Free Day Trading Course: (Lesson 1 of 10) Introduction To Day Trading Stocks
Learn Chinese for Beginners | Beginner Chinese Lesson 1: Self-Introduction in Chinese Mandarin 1.1
Lecture 1: Introduction to Power and Politics in Today’s World
SELF INTRODUCTION | How to Introduce Yourself in English | Tell Me About Yourself Interview Answer
Комментарии