filmov
tv
Statistical Mechanics Lecture 4
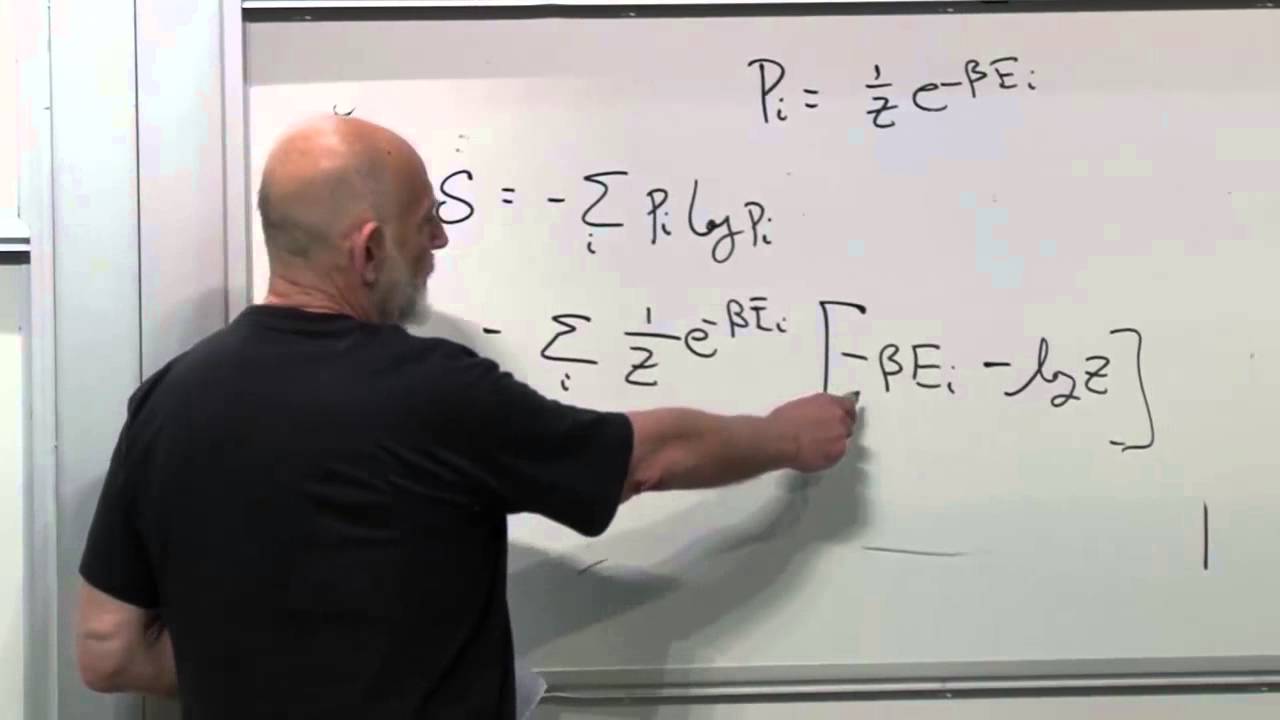
Показать описание
(April 23, 2013) Leonard Susskind completes the derivation of the Boltzman distribution of states of a system. This distribution describes a system in equilibrium and with maximum entropy.
Originally presented in the Stanford Continuing Studies Program.
Stanford University:
Continuing Studies Program:
Stanford University Channel on YouTube:
Originally presented in the Stanford Continuing Studies Program.
Stanford University:
Continuing Studies Program:
Stanford University Channel on YouTube:
Statistical Mechanics Lecture 4
Statistical Mechanics (CMP-SM) Lecture 4 Part 1
Statistical Mechanics (CMP-SM) Lecture 4
Lecture 4: Statistical mechanics of long-range interacting systems
Statistical Mechanics - Week 4 | Lecture 3
Statistical Mechanics - Week 4 | Lecture 1
Statistical Mechanics (CMP-SM) Lecture 4
Lecture 4: Statistical mechanics of long-range interacting systems
Wavelet-inspired Nash’s Iteration (Lecture 4) by Vikram Giri
Statistical Physics of Biological Evolution by Joachim Krug ( Lecture - 4 )
Teach Yourself Statistical Mechanics In One Video
Statistical Mechanics Lecture 1
Statistical Mechanics - Week 4 | Lecture 2
Statistical Mechanics Lecture 5
4. Thermodynamics Part 4
Statistical Physics of Turbulence (Lecture 4) by Jeremie Bec
Lecture 04, concept 11: Statistical mechanics connects microstates to macrostates
Dr. Arnab Sen : Lecture 4 : Quantum Statistical Mechanics
Statistical Mechanics Lecture 3
Statistical Mechanics (CMP-SM) Lecture 4 Part 2
Statistical Mechanics (Overview)
Gibbs paradox : Statistical Mechanics 4 Reference R K Pathria
Why greatest Mathematicians are not trying to prove Riemann Hypothesis? || #short #terencetao #maths
Statistical Mechanics Lecture 6
Комментарии