filmov
tv
ORCA 2 | Microsoft's BREAKTHROUGH in Open Source LLMs - AI training the next gen AI 🔥
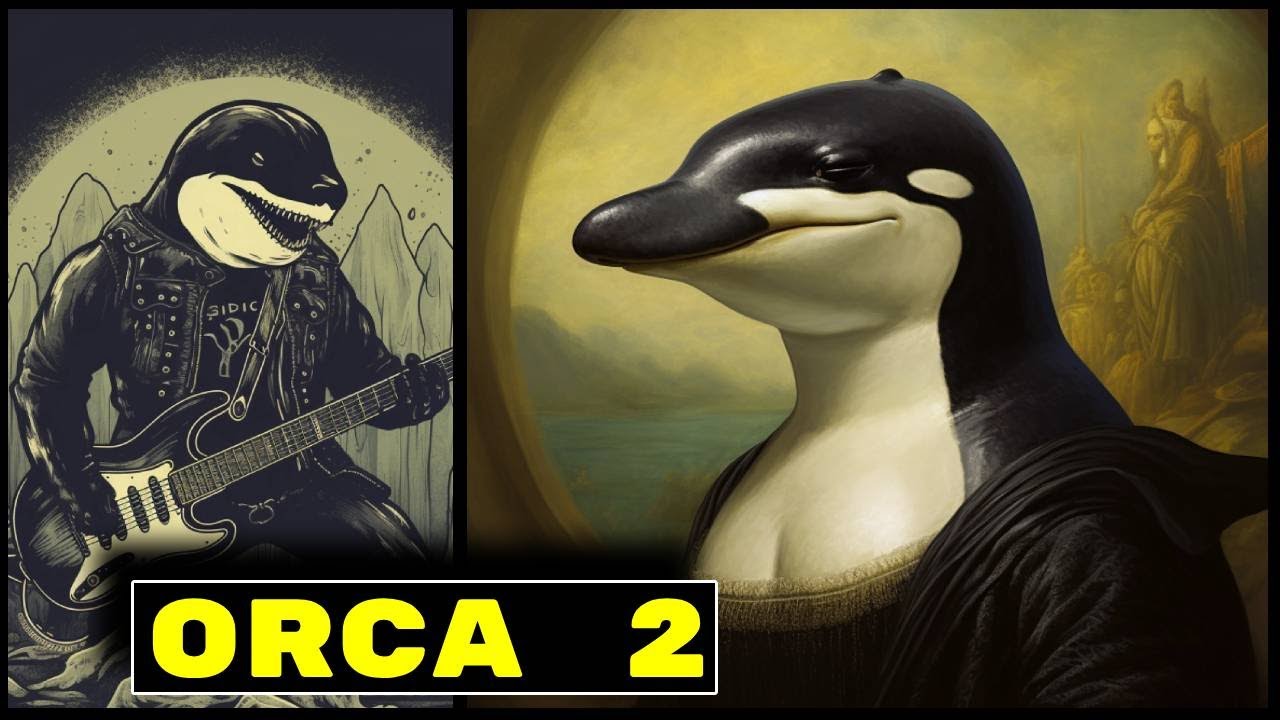
Показать описание
Get on my daily AI newsletter 🔥
[News, Research and Tutorials on AI]
See more at:
My AI Playlist:
[News, Research and Tutorials on AI]
See more at:
My AI Playlist:
ORCA 2 | Microsoft's BREAKTHROUGH in Open Source LLMs - AI training the next gen AI 🔥
Orca 2 🐳 GIANT Breakthrough For AI Logic/Reasoning
Microsoft's New AI Orca-2 Just Changed EVERYTHING! (Synthetic Data Breakthrough)
ORCA 2 Microsoft's BREAKTHROUGH in Open Source LLMs | Installing & Testing ORCA-2 🔥🔥
How Orca 2 Beats Bigger AI Models: Microsoft's New AI Revolutionizes Reasoning Abilities!
Microsoft’s ORCA 2: New AI Outperforms GPT-4 in Intricate Tasks! PT. 1 | AI News
Orca - New ChatGPT competitor made by Microsoft.
Microsoft's ORCA 2: New AI Outperforms GPT-4 in Intricate Tasks! PT. 2 |AI News
Microsoft's ORCA 2: New AI Outperforms GPT-4 in Intricate Tasks!
Microsoft’s NEW Insane 'KOSMOS 2' Taking Everyone by SURPRISE! (Multimodal)
Smaller, Smarter, Stronger: Orca 2 Challenges GPT-4's Dominance
Unveiling Microsoft's AI Marvel - ORCA 2: Surpassing GPT-4 in Complex Tasks! 🚀🧠#shorts
Microsoft’s Orca SHOCKS the entire industry - STUNNING GPT 4 competitor
Microsoft's New AI 'ORCA' INSANE GPT Rival
Insane New AI Model - ORCA - That Finally Beats GPT-4
Orca LLM: Opensource Model SURPRISING Everyone!
Microsoft's Kosmos-2 Leading The Entire Industry To AGI
ORCA 2: Andrej Karpathy, Synthetic Data, GPT4 - AI Paper Explained
Microsoft Orca: The Game-Changing AI You NEED to See!!!
Did you hear about Small Language Models? 🤯👀😱
NEW 'Orca' 🐳 Model 'Free Willy' Has Surprising Results
Microsoft's New 'ORCA' Takes Everyone by Surprise!
KOSMOS-2: Microsoft's New AI Breakthrough Generating Text, Images, Video & Sound in Real-Ti...
📱Microsoft Orca: The Groundbreaking Tech Announcement That's Taking the World By Storm!🌪️ #orca...
Комментарии