filmov
tv
Combining deep learning model compression techniques
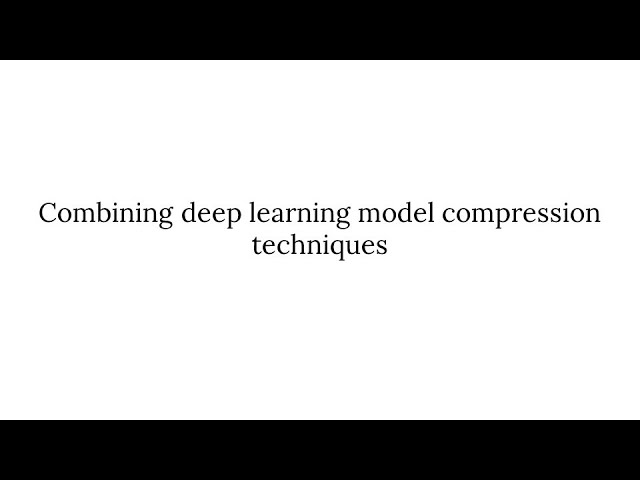
Показать описание
In this article, we evaluate the performance of combining several model compression techniques. The techniques assessed were dark knowledge distillation, pruning, and quantization. We found that in the scenario in which we developed the experiments, classification of chest x-rays, the combination of these three techniques yielded a new model capable of aggregating the individual advantages of each one. In the experiments we used a combination of deep models with 95.05% accuracy, a value higher than that reported in some related works but lower than the state of the art, whose accuracy is 96.39%. The accuracy of the compressed model in turn was 90.86%, a small loss compared to the gain obtained from the reduction, in bytes, in relation to the size of the original model. The size has been reduced from 841MB to 40KB, which opens up the possibility for using deep models in edge computing applications.