filmov
tv
PDF Sampling: MCMC - Metropolis-Hastings algorithm
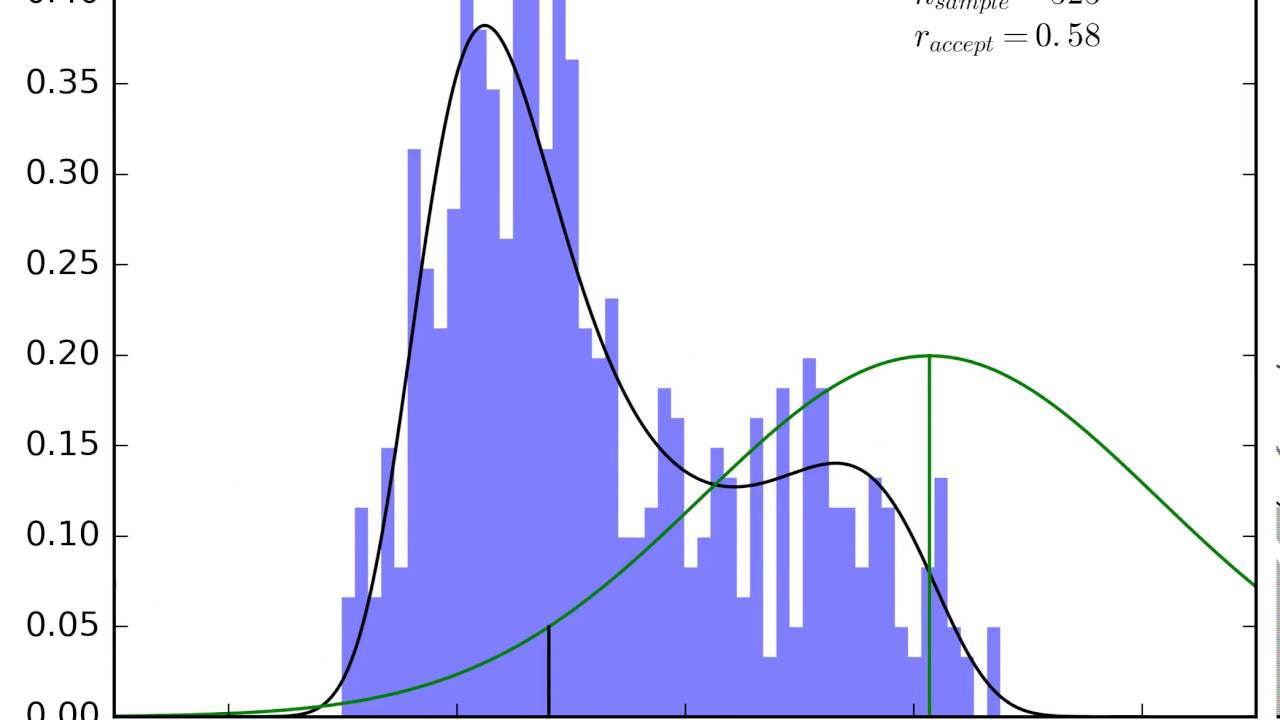
Показать описание
Probability density function sampling using Markov-Chain Monte Carlo: Metropolis-Hastings algorithm
Green curve is the proposed distribution.
Green vertical line is the position of last state
Black vertical line is the proposed state that accepted
Red vertical line is the position of the last state that become a sample again because proposed state is rejected
Green curve is the proposed distribution.
Green vertical line is the position of last state
Black vertical line is the proposed state that accepted
Red vertical line is the position of the last state that become a sample again because proposed state is rejected
PDF Sampling: MCMC - Metropolis-Hastings algorithm
Introduction to Bayesian statistics, part 2: MCMC and the Metropolis–Hastings algorithm
Understanding Metropolis-Hastings algorithm
Lecture 16. Gibbs Sampling
Machine Learning II Lecture 9
Metropolis-Hastings - VISUALLY EXPLAINED!
An introduction to the Random Walk Metropolis algorithm
MCMC (7): The Metropolis-Hastings method
Metropolis-Hastings sampling algorithm
Advanced Bayesian Methods: Metropolis Hastings
39 - Metropolis Hastings
Metroplis-Hastings independence sampler example
Gibbs Sampling : Data Science Concepts
Metropolis Hasting algorithms under MCMC
A Beginner's Guide to Monte Carlo Markov Chain MCMC Analysis 2016
Metropolis Hastings sampling
Optimization of Proposal Distribution for the Metropolis-Hastings Algorithm
M4 | MCMC sampling & Optimization methods | CIV6540E
Lecture 17. Markov Chain Monte Carlo and Metropolis-Hasting Algorithm
Using the Random Walk Metropolis algorithm to sample from a cow surface distribution
Metropolis-Hastings MCMC Demo
2023-01-23 PRML - MCMC
Metropolis Hastings MCMC
Markov Chain Monte Carlo: Metropolis-Hastings-Sampler Part1
Комментарии