filmov
tv
Semantic segmentation using Deep Learning models
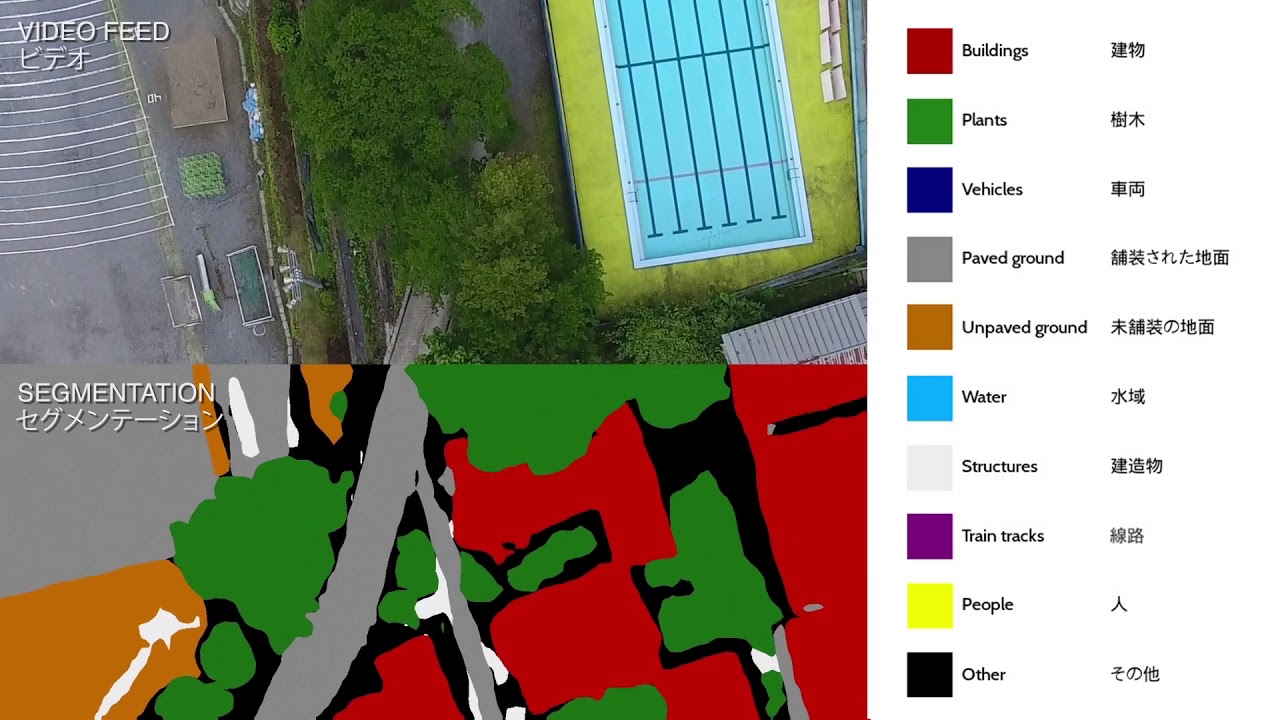
Показать описание
Speedup of deep learning ensembles for semantic segmentation using a model compression technique.
Highlights:
• We apply model compression to the problem of semantic segmentation (sem. seg.).
• Compressed models have real-time performance and similar accuracy to ensembles.
• Compression works on ensembles containing entirely distinct deep architectures.
• A good upscaling technique matters more than network depth for sem. seg.
• The sem. seg. outputs show what parts of an ensemble are preserved by compression.
Check our paper at the link:
Many thanks to the NII, Tokyo for sponsoring my stay in Tokyo and Prendinger Lab for hosting and facilitating the research. Thanks to Andrew Holliday, Mohammadamin Barekatain, Helmut Prendinger,
Johannes Laurmaa, and Tada Naoki.
Highlights:
• We apply model compression to the problem of semantic segmentation (sem. seg.).
• Compressed models have real-time performance and similar accuracy to ensembles.
• Compression works on ensembles containing entirely distinct deep architectures.
• A good upscaling technique matters more than network depth for sem. seg.
• The sem. seg. outputs show what parts of an ensemble are preserved by compression.
Check our paper at the link:
Many thanks to the NII, Tokyo for sponsoring my stay in Tokyo and Prendinger Lab for hosting and facilitating the research. Thanks to Andrew Holliday, Mohammadamin Barekatain, Helmut Prendinger,
Johannes Laurmaa, and Tada Naoki.