filmov
tv
The Math Behind Bayesian Classifiers Clearly Explained!
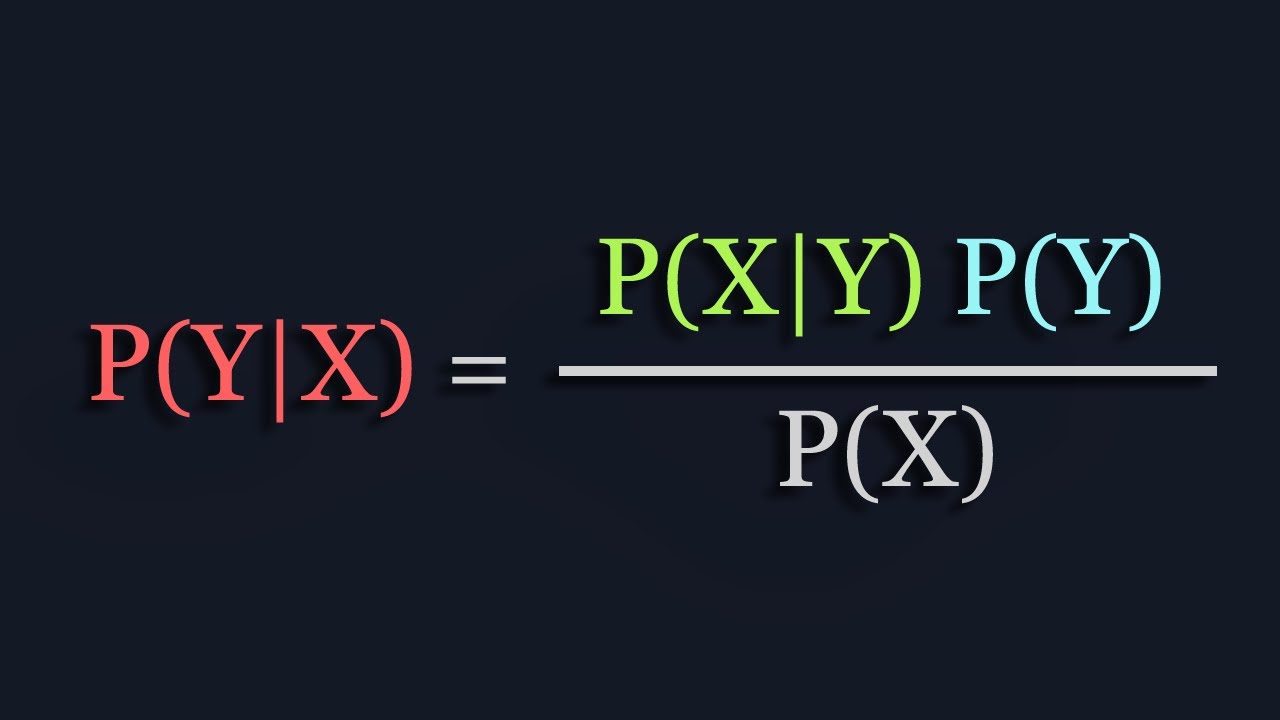
Показать описание
In this video, I've explained the math behind Bayes classifiers with an example. I've also covered the Naive Bayes model.
#machinelearning #datascience
For more videos please subscribe -
Support me if you can ❤️
The math behind GANs -
Source code -
3blue1brown -
Facebook -
Instagram -
Twitter -
#machinelearning #datascience
For more videos please subscribe -
Support me if you can ❤️
The math behind GANs -
Source code -
3blue1brown -
Facebook -
Instagram -
Twitter -
The Math Behind Bayesian Classifiers Clearly Explained!
Bayes theorem, the geometry of changing beliefs
Bayes' Theorem EXPLAINED with Examples
Naive Bayes, Clearly Explained!!!
Bayesian classification
Tutorial 48- Naive Bayes' Classifier Indepth Intuition- Machine Learning
Naive Bayes Classifier ! Complete Intuition and Maths Behind ! 🔥🔥🔥
#19 Bayesian Classification - Bayes Theorem, Naive Bayes Classifier |DM|
Naive Bayes Classifier | Naive Bayes Algorithm | Naive Bayes Classifier With Example | Simplilearn
1. Solved Example Naive Bayes Classifier to classify New Instance PlayTennis Example Mahesh Huddar
Naive Bayes classifier: A friendly approach
Naive bayes classifier explained for beginners
Naive Bayes Lecture 2 The Naive Bayes Classifier
#44 Naive Bayes Classifier With Example |ML|
Lec-8: Naive Bayes Classification Full Explanation with examples | Supervised Learning
Why Naive Bayes Is Naive? #datascience #machinelearning #artificialintelligence #statistics
Gaussian Naive Bayes, Clearly Explained!!!
Naive Bayes Classification technique Explained Clearly.
Naive Bayes Classifiers
Naive Bayes Maths clearly explained!
7. Solved Example Naive Bayes Classification Age Income Student Credit Rating Buys Computer Mahesh
#43 Bayes Optimal Classifier with Example & Gibs Algorithm |ML|
Naïve Bayes Model in 1 Minute
Classification with Bayes Theorem
Комментарии