filmov
tv
New AI Chatbot - Pi - Outperforms ChatGPT (AI with VOICE)
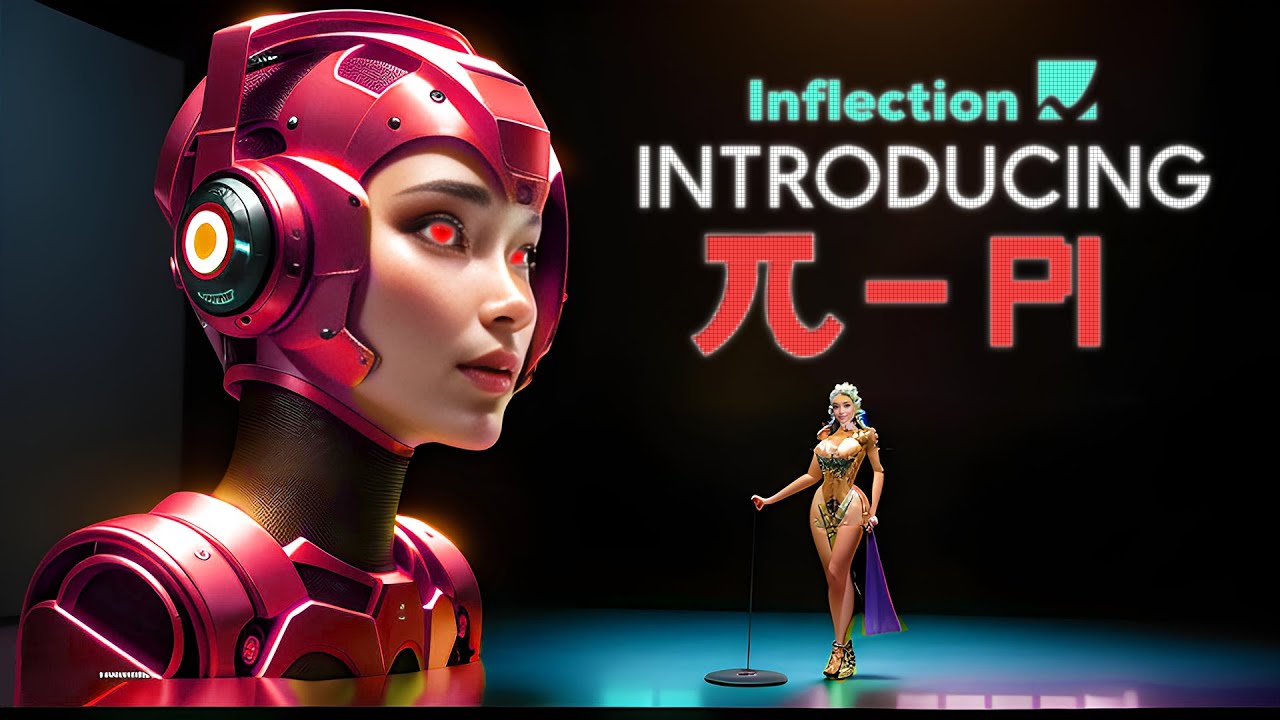
Показать описание
Meet Pi, the AI chatbot designed for personal assistance and emotional support, which outperforms ChatGPT with its empathetic understanding of human emotions. Using Deep Reinforcement Learning and Natural Language Processing techniques, Pi learns from interactions with users and can even help improve their empathy and emotional intelligence skills. While some critics question the implications of becoming emotionally attached to a machine, Pi offers an interesting glimpse into the potential future of AI companionship.
Meet Your New AI Bestie - Pi - The World’s Friendliest Chatbot!
New AI Chatbot - Pi - Outperforms ChatGPT (AI with VOICE)
ChatGPT Meets Pi: An AI 'Duel' Reveals the Future of Voice Assistants
I Tested the New Pi AI, and This Happened ...
New AI Chatbot - Pi - Outperforms ChatGPT (Artificial Intelligence with VOICE)
I Tried PI AI: A New AI Assistant And THIS Happened
Meet Pi AI: The Chatbot Revolutionizing Conversations! 🤖✨
I Ran ChatGPT on a Raspberry Pi Locally!
The Pi Cast (6/8) Particle Founder & CEO Zach Supalla Talks Tachyon SBC
Is the new Raspberry Pi AI Kit better than Google Coral?
Pi vs. Pi: What happens when AI's talk to each other?
How to use PI by inflection AI (for Beginners)
The New AI Chatbot Will Get You PROMOTED [PI Personal Intelligence]
New AI Chatbot - Pi | Outperforms ChatGPT (AI with VOICE)
12 New AI Projects using Raspberry-Pi, Jetson Nano & more
ChatGPT Running Locally on Raspberry Pi (ft. Ryan Reynolds)
Don't Sleep on Inflection AI's Pi - Big update!
Stop Using ChatGPT, Use PI AI Instead.
Pi AI - The NEW Personal AI That Will Change EVERYTHING!
Pi Ai Product Review
Inflection Pi AI chatbot #aipodcast #podcast #artificialintelligence
I Ran Advanced LLMs on the Raspberry Pi 5!
Creepy or Cool? Exploring Personal AI - PI AI Blew Our Minds!
DER BESTE KI-CHATBOT, VON DEM DU NOCH NIE GEHÖRT HAST – Pi von Inflection ist das KI-Tool der Woche...
Комментарии